GigaGAN shows that Generative Adversarial Networks are far from obsolete and could be a faster alternative to Stable Diffusion in the future.
Current generative AI models for images are diffusion models trained on large datasets that generate images based on text descriptions. They have replaced GANs (Generative Adversarial Network), which were widely used in the past, as they outperformed them in the quality of generated images for the first time in 2021.
However, GANs are much faster to synthesize pictures and their structure makes them easier to control. Models such as StyleGAN were practically the standard before the breakthrough of diffusion models.
Now, with GigaGAN, researchers from POSTECH, Carnegie Mellon University, and Adobe Research demonstrate a billion-parameter GAN model that, like Stable Diffusion, DALL-E 2, or Midjourney, has been trained on a large dataset and is capable of text-to-image synthesis.
GigaGAN is significantly faster than Stable Diffusion
GigaGAN is six times larger than the largest GAN to date and was trained by the team using the LAION-2B dataset of over 2 billion image-text pairs and COYO-700M. An upscaler based on GigaGAN was trained using Adobe Stock photos.
According to the paper, this scaling is only possible through architectural changes, some of which are inspired by diffusion models.
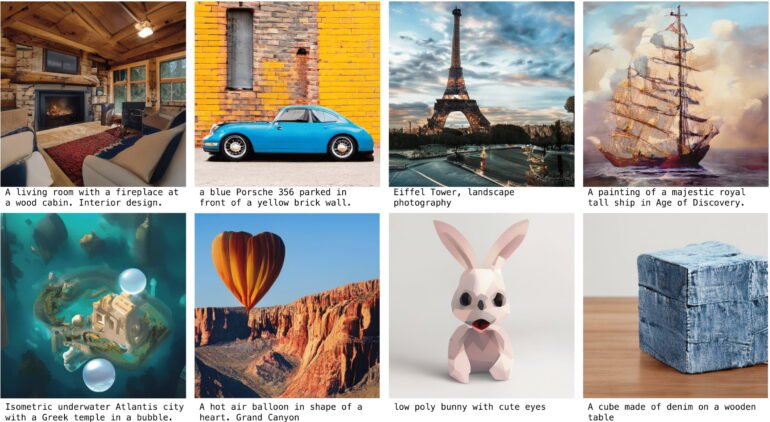
After training, GigaGAN is able to generate 512 x 512 pixel images from text descriptions. The content is clearly recognizable, but does not yet reach the quality of high-quality diffusion models in the examples provided.
On the other hand, GigaGAN is between 10 and 20 times faster than comparable diffusion models: On an Nvidia A100, GAN generates an image in 0.13 seconds, Muse-3B takes 1.3 seconds and Stable Diffusion (v.1.5) 2.9 seconds.
Scaling up to larger models also promises to improve quality, so expect much bigger - and better - GANs in the future.
Further scaling could take GigaGAN to the level of the best generative AI models
"Our GigaGAN architecture opens up a whole new design space for large-scale generative models and brings back key editing capabilities that became challenging with the transition to autoregressive and diffusion models. We expect our performance to improve with larger models."
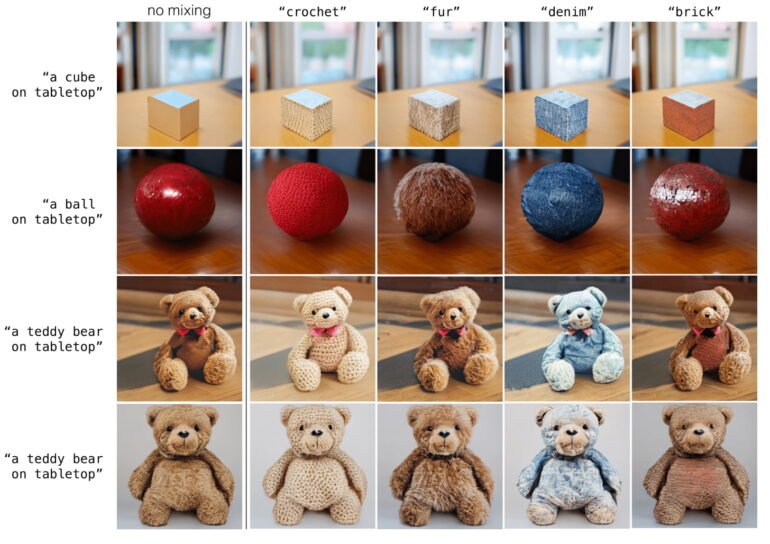
The GAN architecture also allows the images to be easily modified, like changing the material of the objects or the time of day. Diffusion models offer similar possibilities, but they have to rely on external methods, tricks, or manual labor.
GigaGAN's upscaling variant is particularly impressive: the model converts a 128-pixel image into a high-resolution 4K image in 3.66 seconds. The details that the model adds in the examples shown are photo-realistic.
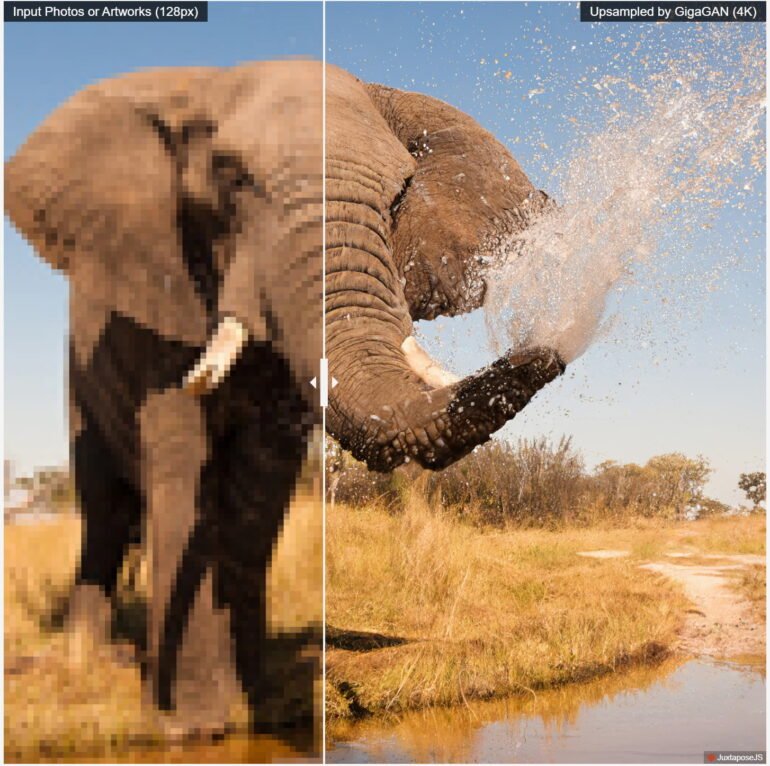
So far, there seem to be no plans to release the models. A variant of the upscaler could be integrated into Adobe Firefly or Photoshop, for example.
More examples and information can be found on the GigaGAN project page.