I-JEPA shows how Metas AI chief Yann LeCun sees the future of AI - and it all starts again with ImageNet benchmarks.
Less than a year ago, AI pioneer and Meta AI chief Yann LeCun unveiled a new AI architecture designed to overcome the limitations of current systems, such as hallucinations and logical weaknesses. With I-JEPA, a team from Meta AI (FAIR), McGill University, Mila, Quebec AI Institute and New York University presents one of the first AI models to follow the "Joint Embedding Predictive Architecture". The researchers include first author Mahmoud Assran and Yann LeCun.
The Vision Transformer-based model achieves high performance in benchmarks ranging from linear classification to object counting and depth prediction, and is more computationally efficient than other widely used computer vision models.
I-JEPA learns with abstract representations
I-JEPA is trained in a self-supervised manner to predict details of the unseen parts of an image. This is done by simply masking large blocks of those images whose content I-JEPA is supposed to predict. Other methods often rely on much more extensive training data.
To ensure that I-JEPA learns semantic, higher-level representations of objects and does not operate at the pixel or token level, Meta places a kind of filter between the prediction and the original image.
In addition to a context encoder, which processes the visible parts of an image, and a predictor, which uses the output of the context encoder to predict the representation of a target block in the image, I-JEPA consists of a target encoder. This target encoder sits in between the full image, which serves as a training signal, and the predictor.
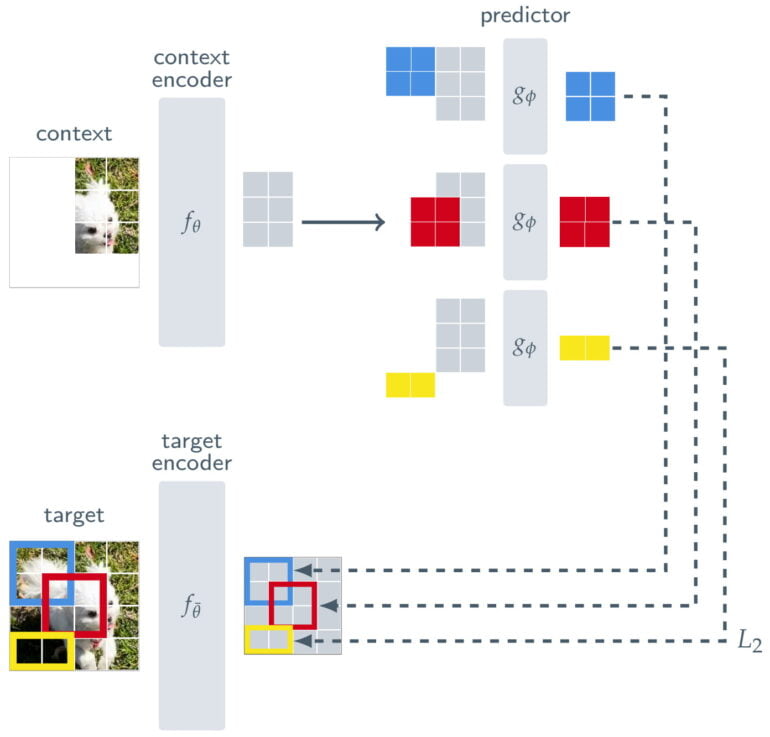
Thus, I-JEPA's prediction is not done at the pixel level, but at the level of abstract representations as the image is processed by the target encoder. With this the model uses "abstract prediction targets for which unnecessary pixel-level details are potentially eliminated," Meta says, thereby leading the model to learn more semantic features.
I-JEPA shines in ImageNet
The learned representations can then be reused for different tasks, allowing I-JEPA to achieve great results in ImageNet with only 12 labeled examples per class. The 632 million parameter model was trained on 16 Nvidia A100 GPUs in less than 72 hours. Other methods typically require two to ten times as many GPU hours and achieve worse error rates when trained on the same amount of data.
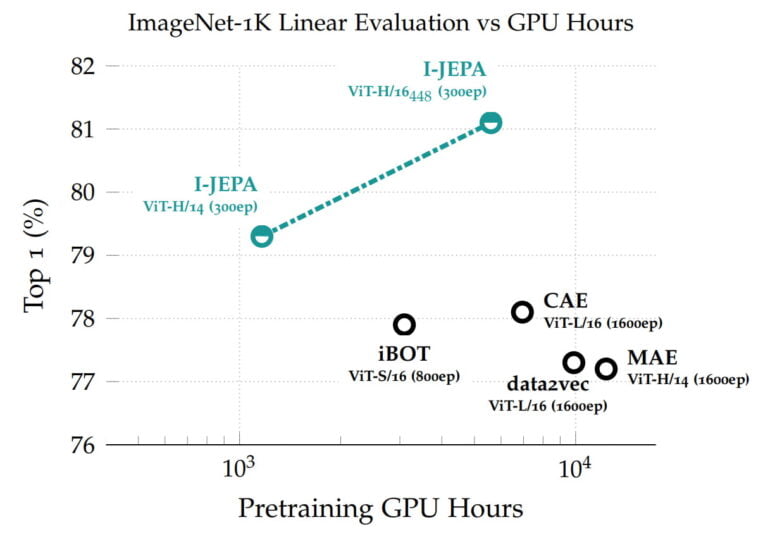
In an experiment, the team uses a generative AI model to visualise I-JEPA's representations and shows that the model learns as expected.
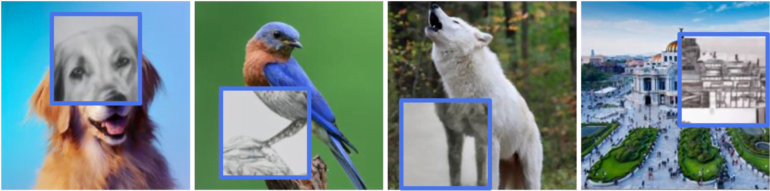
I-JEPA is a proof of concept for the proposed architecture, whose core element is a kind of filter between prediction and training data, which in turn enables abstract representations. According to LeCun, such abstractions could allow AI models to more closely resemble human learning, make logical inferences, and solve the hallucination problem in generative AI.
JEPA could enable world models
The overall goal of JEPA models is not to recognize objects or generate text - LeCun wants to enable comprehensive world models that function as part of autonomous artificial intelligence. To achieve this, he proposes to stack JEPA hierarchically to enable predictions at a higher level of abstraction based on predictions from lower modules.
"It would be particularly interesting to advance JEPAs to learn more general world models from richer modalities, e.g., enabling one to make long-range spatial and temporal predictions about future events in a video from a short context, and conditioning these predictions on audio or textual prompts," Meta said.
JEPA will therefore be applied to other domains such as image-text pairs or video data. "This is an important step towards applying and scaling self-supervised methods for learning a general model of the world," the blog states.
LeCun provides more insights into the motivation, development, and operation of JEPA in a talk at Northeastern University's Institute for Experiential AI.
More information is available on the I-JEPA meta-blog. The model and code are available on GitHub.