Nvidia dominates the sixth round of the established AI benchmark MLPerf with its two-year-old A100 GPU. However, the competition does not sleep.
The MLPerf artificial intelligence benchmark has been conducted by MLCommons since 2018. The test is supposed to enable a transparent comparison of different chip architectures and system variants in AI calculations.
Participating companies include chip manufacturers such as Nvidia, Google, Graphcore or Intel's Habana Labs, as well as server manufacturers such as Inspur, Fujitsu or Lenovo. A total of 24 companies participated with their products in this year's MLPerf Training Benchmark 2.0.
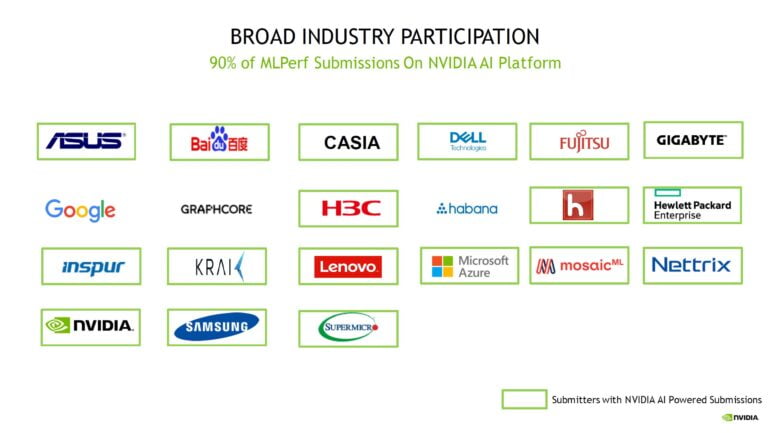
Last year, a Graphcore IPU-POD16 with 16 MK2000 chips beat an Nvidia DGX A100 640 system in training a ResNet 50 model for the first time - by 60 seconds. However, Nvidia considered the comparison inappropriate, since its system only has eight chips installed. Thus, Nvidia showed the best per-chip performance in MLPerf Training 1.1.
MLPerf Training 2.0: Nvidia owns 90 percent
Nvidia's systems dominate this year's benchmark as well: of all the submissions in the benchmark, 90 percent are built on Nvidia's AI hardware. The remaining three participants are Google's TPUv4, Graphcore's new BOW IPU, and Intel Habana Labs' Gaudi 2 chip.
All Nvidia systems rely on the two-year-old Nvidia A100 Tensor Core GPU in the 80-gigabyte variant and participate in all eight training benchmarks in the closed competition. Google only participates in the RetinaNet and Mask R-CNN benchmarks, Graphcore and Habana Labs only in the BERT and RestNet-50 benchmarks.
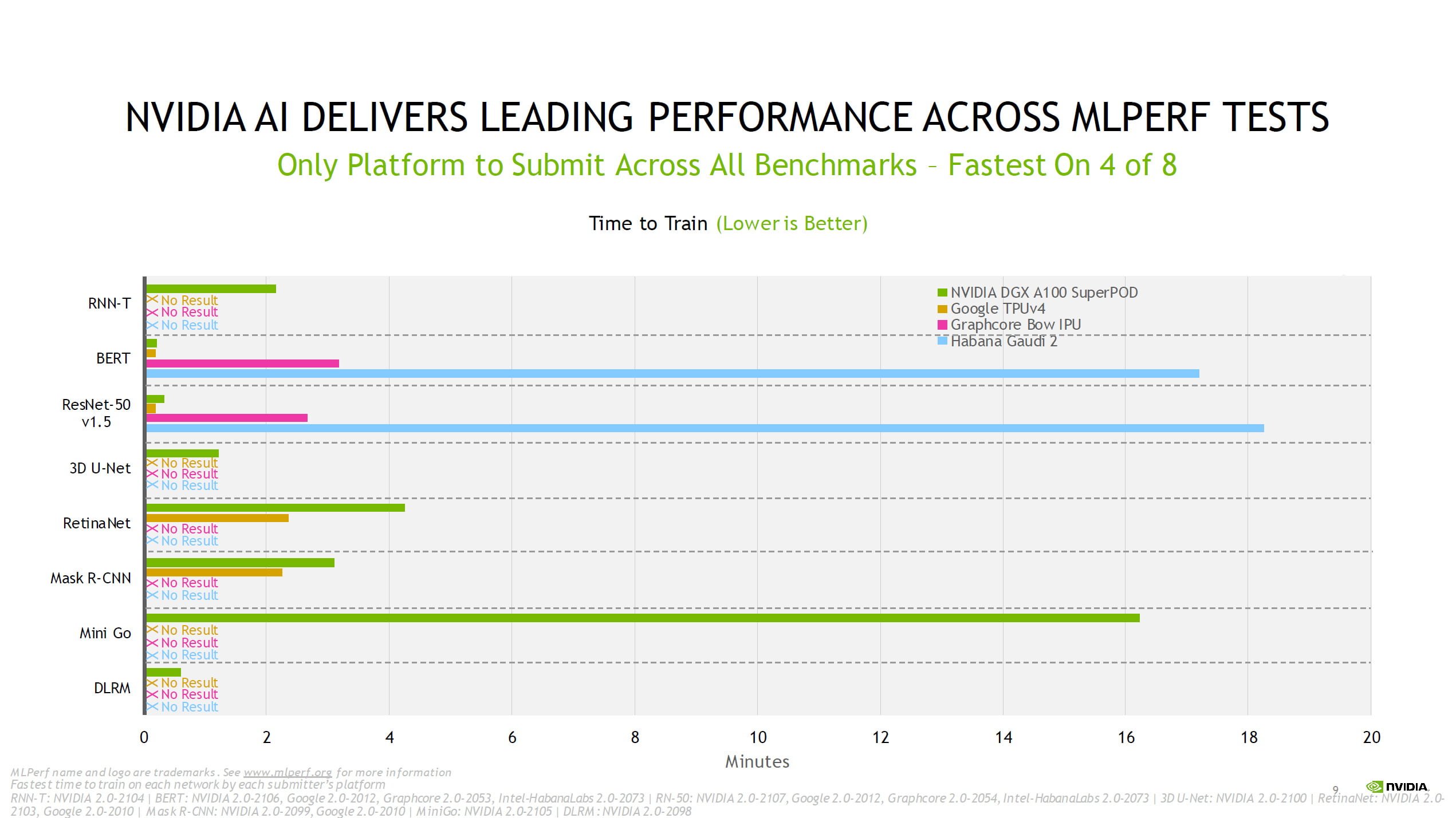
According to Nvidia, the A100 also retains its leading position in a performance-per-chip comparison and is the fastest in six of the eight tests.
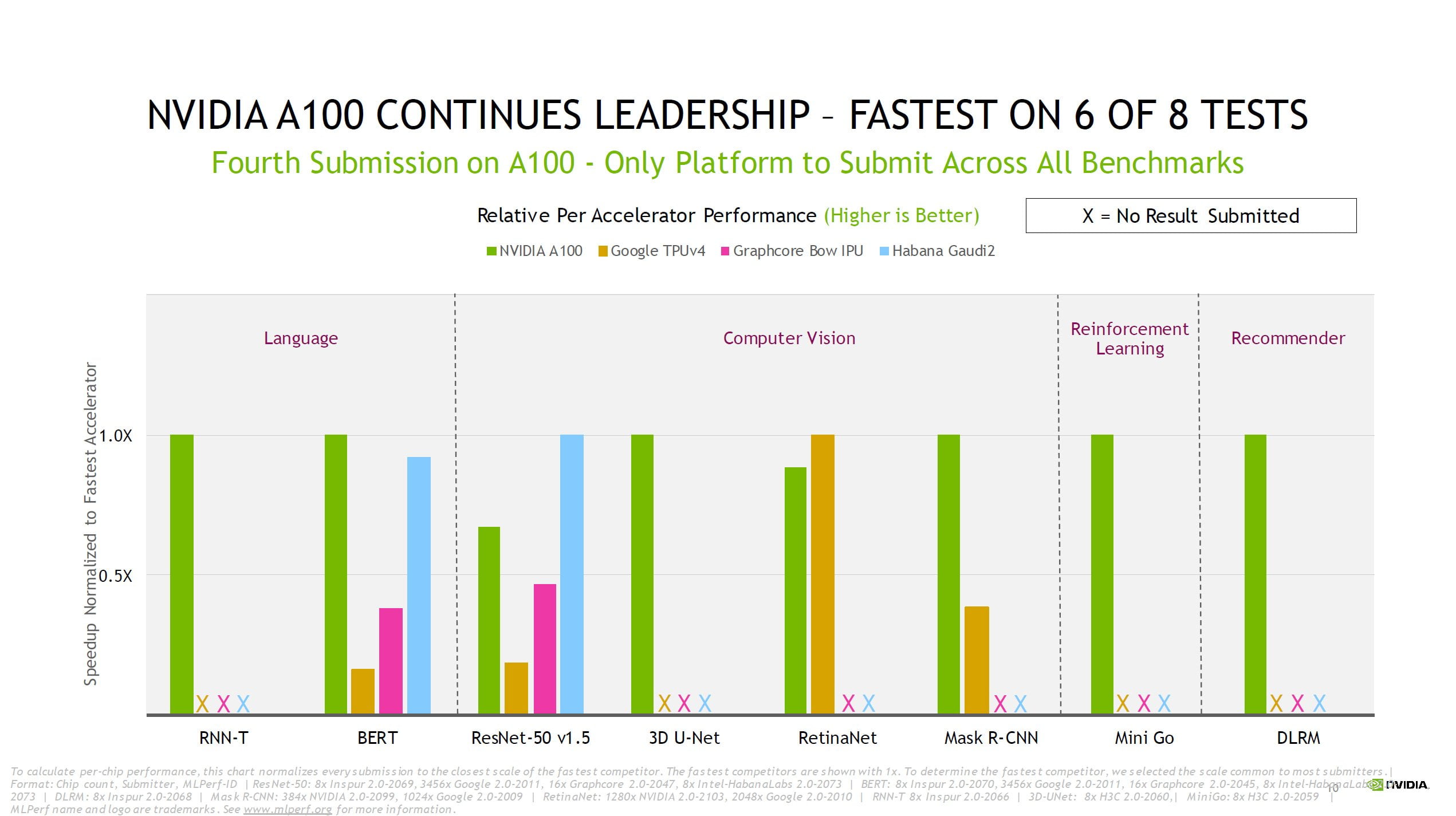
Since the first tests began in 2018, Nvidia's AI platform has increased training performance by a factor of 23 thanks to the jump from V100 to A100 as well as numerous software improvements, the company says.
Nvidia sees one of the biggest advantages of its platform in its versatility: Even relatively simple AI applications, such as asking questions about an image via voice input, require multiple AI models.
Developers need to be able to design, train, use, and optimize these models quickly and flexibly. Therefore, AI hardware diversity - the ability to run any model in MLPerf and beyond - and high performance are critical for real-world AI product development.
Nvidia also highlights being the only company able to show real-world performance in supercomputer configurations. This, it says, is important for training large AI models such as GPT-3 or Megatron Turing NLG.
Graphcore shows performance leap and willingness to cooperate
British chipmaker Graphcore is entering the race for the first time with the new BOW IPU. With the better hardware and software improvements, Graphcore achieves a 26 to 31 percent faster training time in the ResNet-50 benchmark, and in the BERT benchmark it is 36 to 37 percent on average - depending on the system.
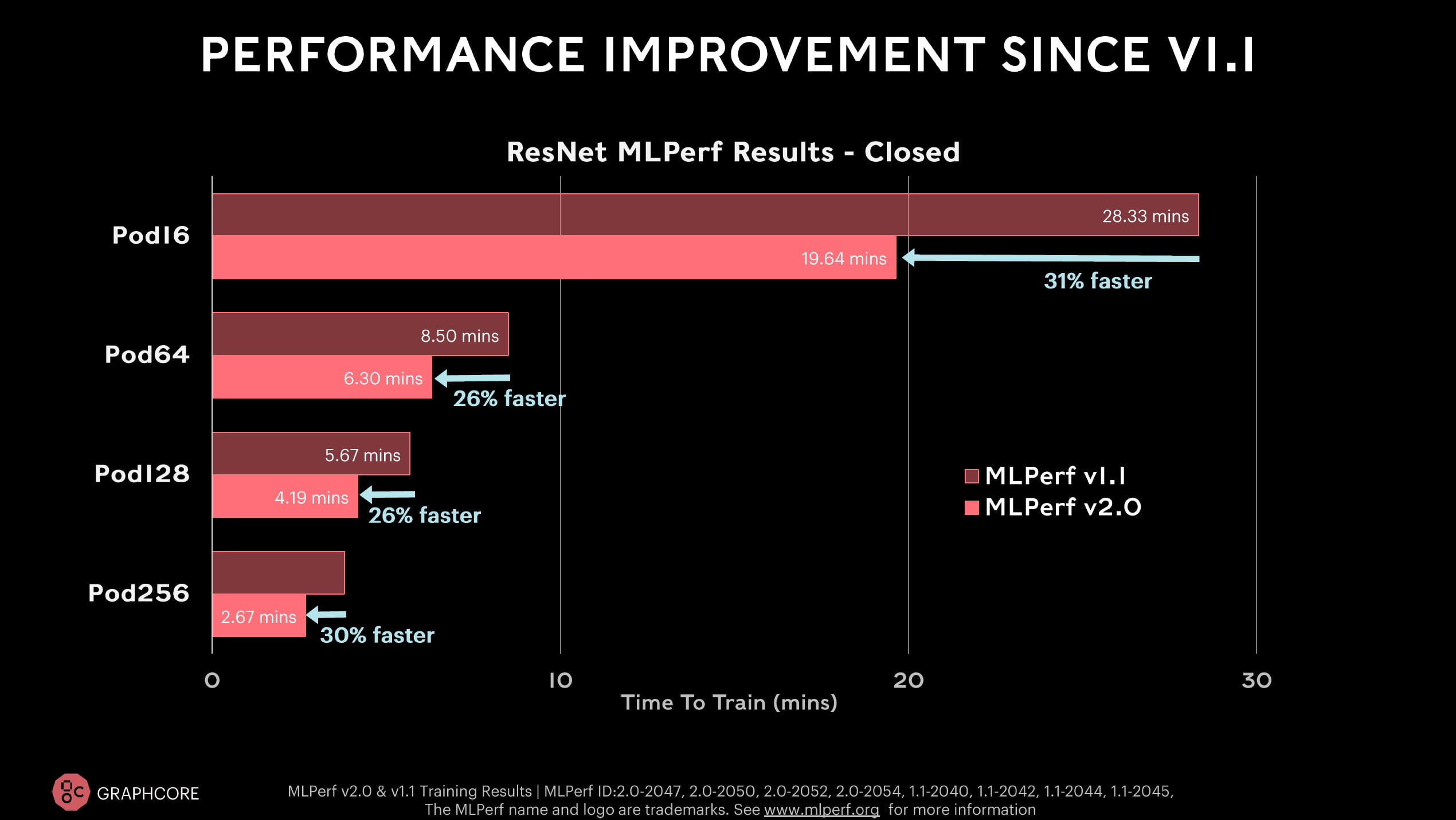
For the first time, an external company with a Graphcore system also participates in the benchmark. Baidu submits BERT values for a Bow-Pod16 and a Bow-Pod64 and uses the AI framework PaddlePaddle, which is widely used in China.
The values achieved in training are on par with Graphcore's submissions in the internal PopART framework. For Graphcore, this is a sign that its chips can also achieve good results in other frameworks.
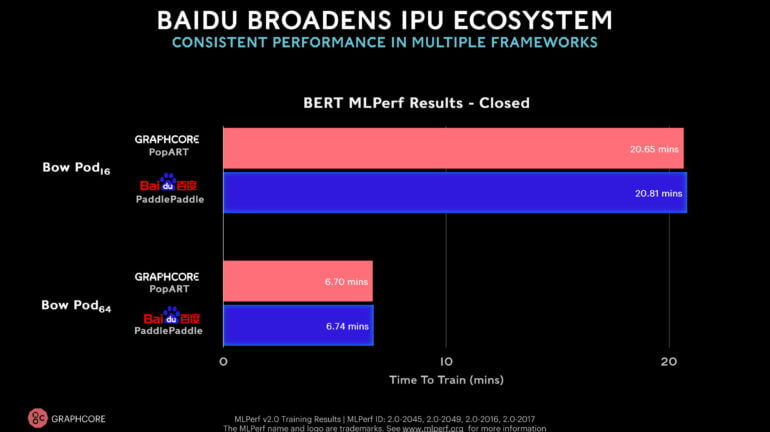
According to Graphcore, the new Bow-Pod16 is clearly ahead of Nvidia's DGX-A100 server in the ResNet-50 benchmark and offers competitive prices.
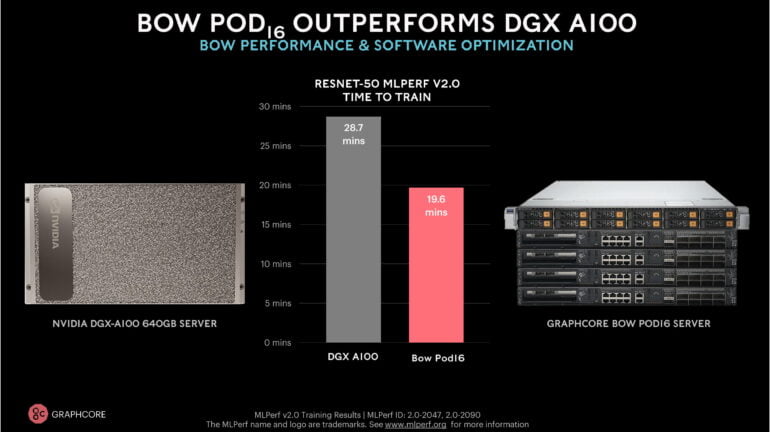
Graphcore does not want to compete directly with Nvidia
In a press briefing on the MLPerf results, Graphcore points out the different architecture of its products: Nvidia, Google and Intel produce similar vector processors, whereas Graphcore's IPU is a graph processor.
The participation in the MLPerf benchmark should therefore primarily show that Graphcore's IPU can deliver comparable performance. However, the hardware offers more.
"For us, if all we do is just copy Nvidia and build Nvidia products, that is very difficult because Nvidia already builds the best GPUs. Anybody who builds something similar is going to have a really hard time differentiating against it. So we're doing something different," Graphcore said.
The company works with a variety of customers using a variety of architectures and models - including ones that other MLPerf participants would still call experimental, such as Vision Transformers. Those don't show up in the benchmark.
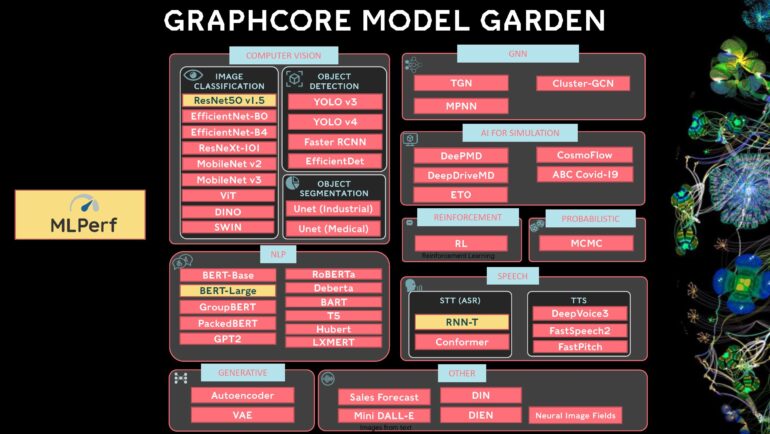
Similarly, in the open MLPerf benchmark, Graphcore submitted results of a modified RNN-T model that was developed in cooperation with a company. However, this differs from the RNN-T model in the closed competition, according to Graphcore. The AI benchmark, designed as an industry standard, is probably not yet flexible enough, at least for the UK company.
Another key differentiator for Graphcore compared to Nvidia is its current significantly better performance on graph neural networks that use small lot sizes and require dynamic memory usage. The EfficientNet model also benefits from Graphcore's IPUs. In both cases, the company sees the advantage over GPUs in the different chip architecture of its products.
Graphcore also recently announced a cooperation with the German AI startup Aleph Alpha. All results and more information are available on the MLCommons MLPerf benchmark website.