MIT economist Aidan Toner-Rodgers conducted a large, controlled study examining how an AI research tool affected productivity at an unnamed US company with over 1,000 researchers. The company develops inorganic materials like molecular compounds, crystal structures, glasses, and metal alloys for healthcare, optics, and industrial manufacturing.
Research teams randomly selected to use the customized AI tool discovered 44% more new materials and filed 39% more patent applications compared to teams that stuck with standard workflows. The AI system combines graph neural networks with reinforcement learning, pre-trained on data from extensive databases like the Materials Project for crystal structures and the Alexandria Materials Database for molecular structures.
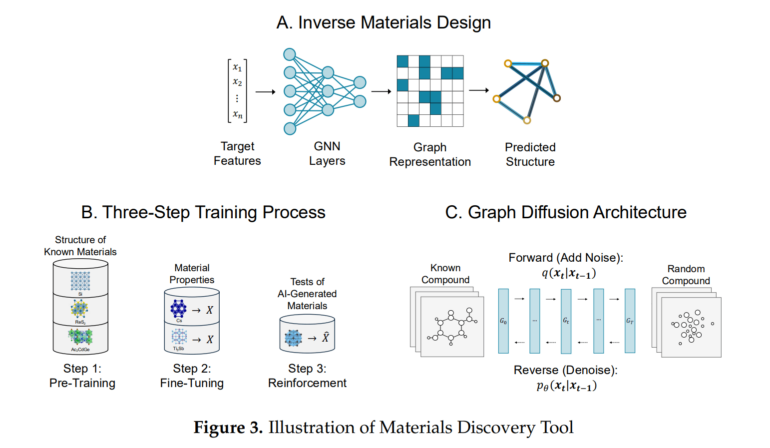
Researchers input desired material properties into the neural network, which then suggests new structures that might have those properties. Teams filter out likely failures, attempt to synthesize promising structures, test them in experiments and even in product prototypes. Results feed back into the neural network to improve its predictive capabilities.
Top performers see biggest gains
Interestingly, the company's highest-performing researchers benefited most from the AI assistance, while lower-performing scientists saw little advantage. Toner-Rodgers suggests top researchers use their expertise to prioritize promising AI suggestions, while others waste resources testing false positives.
In a follow-up survey, researchers using the AI reported lower job satisfaction, as the tool took over some of the more creative steps in their work. Scientists mostly just had to select which suggested materials to advance to the next phase.