Benchmarks are an important way to measure progress in AI research - but artificial intelligence is constantly achieving new bests. Are we running out of AI benchmarks?
Artificial Intelligence benchmarks are closely linked to AI research: They create measurability and comparability, and often even become a research goal. AI benchmarks thus steer - at least in part - AI progress.
The successes of artificial neural networks in the ImageNet benchmark, for example, are considered to have triggered the AI boom of recent years: the results demonstrated the potential of the technology and led to increased investment in further research. They are consequently a key element for the further development of AI.
What to do when we run out of benchmarks?
Today, the ImageNet benchmark still plays a central role in research: New models, such as Google's Vision Transformer, which rely on Transformer architectures and self-supervised learning for image analysis, are compared with ImageNet methods.
This reliance on benchmarks to determine progress becomes a problem when there are consistently top scores in a benchmark and there is no high-quality successor benchmark.
An example of the rapid pace of AI research came from Google and Microsoft in early 2021: researchers from Deepmind and Facebook, among others, unveiled the SuperGLUE language benchmark in August 2019 to replace the already outdated GLUE benchmark.
Less than a year later, AI systems from Google and Microsoft achieved top scores that even surpassed human benchmarks. The SuperGLUE benchmark has thus become obsolete.
33 percent of AI benchmarks are not being used
Researchers at the Medical University of Vienna and the University of Oxford now show in a meta-study of AI benchmarks that saturated or stagnant benchmarks are common. The researchers examined 1,688 benchmarks with 406 tasks in computer vision and natural language processing since 2013, and draw the following conclusions:
- In some cases, there would be continuous growth, such as in the ImageNet benchmark.
- However, a majority of all benchmarks quickly reach technological stagnation or saturation.
- In some cases, a lack of research interest is also a cause of stagnation. The researchers cite the UCF101 action recognition benchmark as an example of saturation.
- However, the dynamics of performance improvement do not follow a clearly discernible pattern: in some cases, phases of stagnation are followed by unpredictable leaps. This is what happened in the PROTEINS benchmark.
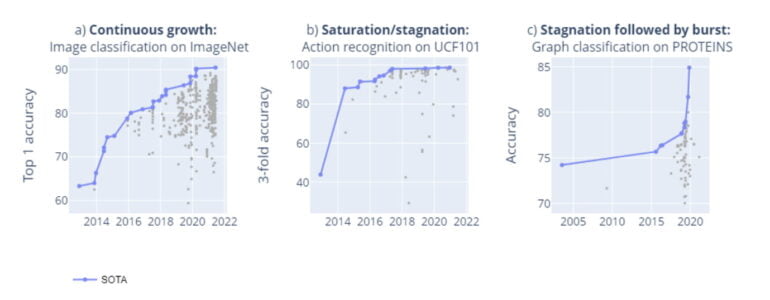
Moreover, of the 1,688 benchmarks, only 66 percent have more than three results at different points in time - so in practice, 33 percent of all AI benchmarks are not used and therefore useless. This points to the trend in recent years that benchmarks tend to be dominated by datasets from established institutions and companies, the researchers say.
Quality over quantity for AI benchmarks
While benchmark successes for computer vision dominated the first half of the last decade, the second half saw a boom in natural language machine processing, according to the researchers.
In 2020, the number of new benchmarks declined and new tests increasingly focused on tasks with a higher level of difficulty, e.g., those that test reasoning. Examples of such benchmarks are Google's BIG-bench and FAIR's NetHack Challenge.
On the one hand, the trend toward benchmarks from established institutions, including industry, raises concerns about the bias and representativeness of benchmarks. On the other hand, criticism of the validity of many benchmarks for capturing the performance of AI systems under real-world conditions suggests that the development of fewer but quality-assured benchmarks covering multiple AI capabilities may be desirable.
In the future, new benchmarks should be developed by large, collaborative teams from many institutions, knowledge domains, and cultures to ensure high-quality benchmarks and avoid fragmentation of the benchmark landscape, the researchers conclude.