AudioGen is the next text-to-X project: The AI system generates audio that matches text input.
Researchers from Meta AI and the Hebrew University of Jerusalem introduce AudioGen: a Transformer-based generative AI model that can generate audio from scratch to match text input or extend existing audio input.
Whistling in the woods - while birds sing and dogs bark
According to the researchers, the AI model solves complex audio problems. For example, it can distinguish between different objects and separate them acoustically, such as when several people are speaking at the same time. It can also simulate background noise such as reverberation.
To train the model in these skills, the researchers used an augmentation technique in the learning phase that mixes different audio samples. In this way, the model learned to separate multiple sources. In total, the researchers assembled ten datasets of audio and matching text annotations.
Subsequently, AudioGen can generate new audio compositions that were not part of the training dataset in this compilation, such as a person walking through the forest whistling while birds chirp in the background. All that is needed is a text prompt, as the following video shows.
Video: Kreuk et al.
The system can also generate music and even sing, according to first author Felix Kreuk, but it is not designed to do so and currently offers few control options.
The researchers want to publish their model
The research team had humans evaluate AudioGen's results via Amazon's Mechanical Turk platform. They evaluated audio quality as well as how well the text and audio fit together, i.e., relevance. More than 85 percent of the 100 randomly selected audio samples contained at least two concepts, for example, "A dog barks while a bird sings."
The testers rated the audio samples on a scale of 1 to 100. In total, the research team had four models evaluated, including the CLIP-based DiffSound with 400 million parameters and three T5-based AudioGen with 285 million to one billion parameters.
The largest AudioGen model scored highest in quality (around 72 points) and relevance (around 68 points) with a clear gap to Diffsound (around 66 / 55 points).
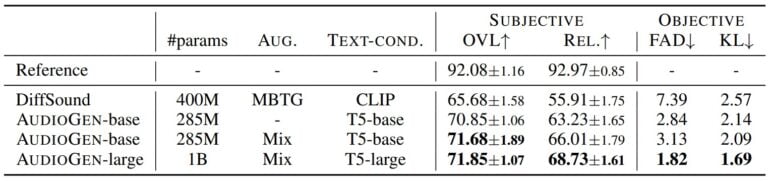
The research team sees AudioGen as the first step toward better text-to-audio models in the future. The technology could also enable semantic audio processing or support the separation of audio sources from discrete units, they say. A release of the model is in the works, according to Kreuk.
In mid-September, Google introduced AudioLM, which also uses techniques of large language models to complete spoken sentences, for example, and generate entirely new audio.