Bloomberg developed a language model specifically for the financial sector. To train the AI, the company used its own financial data and augmented it with online text data. This demonstrates how companies can develop domain-specific language models that are more useful for their industry than generic models.
Bloomberg's AI teams first built a dataset of English-language financial documents: 363 billion financial-specific tokens came from its own data assets, and another 345 billion generic tokens came from online text datasets The Pile, C4, and Wikipedia.
Using 569 billion tokens from this dataset, the team trained the domain-specific "BloombergGPT," a 50-billion-parameter decoder-only language model optimized for financial tasks. The Bloomberg team used the open source Bloom language model as its base architecture.
For finance-specific tasks, BloombergGPT outperforms popular open-source language models such as GPT-NeoX, OPT, and Bloom. But it also outperforms these models on generic language tasks such as summaries, in some cases significantly so, and is almost on par with GPT-3 according to Bloomberg's benchmarks.
"The quality of machine learning and NLP models comes down to the data you put into them," explained Gideon Mann, Head of Bloomberg’s ML Product and Research team.
BloombergGPT illustrates the value of domain-specific language models
According to Bloomberg, language models can be used in many areas of financial technology, from sentiment analysis in articles, such as those related to individual companies, to automatic entity recognition, to answering financial questions. For example, Bloomberg's news division can use the model to automatically generate headlines for newsletters.
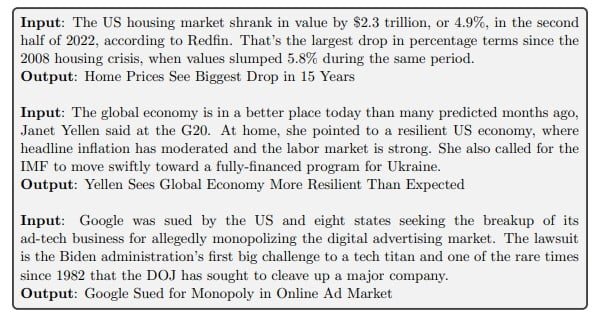
In addition, the model needed only a few examples to formulate queries in Bloomberg's own query language (BQL) to extract data from a database. You can use natural language to tell the model what data you need, and it'll generate the appropriate BQL.
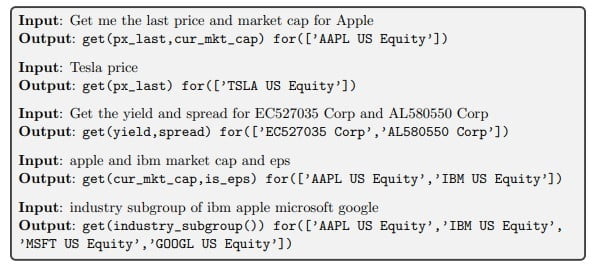
"For all the reasons generative LLMs are attractive – few-shot learning, text generation, conversational systems, etc. – we see tremendous value in having developed the first LLM focused on the financial domain," said Shawn Edwards, Bloomberg’s Chief Technology Officer.
The domain-specific language model, he said, allows Bloomberg to develop many new types of applications and achieve much higher performance than with custom models for each application - all with a faster time to market.