Are giant AI language models like GPT-3 or PaLM under-trained? A Deepmind study shows that we can expect further leaps in performance.
Big language models like OpenAI's GPT-3, Deepmind's Gopher, or most recently Google's powerful PaLM rely on lots of data and gigantic neural networks with hundreds of billions of parameters. PaLM, with 540 billion parameters, is the largest language model to date.
The trend toward more and more parameters stems from the previous finding that the capabilities of large AI models scale with their size. In some cases, the giant networks can solve tasks that their developers did not anticipate.
Google PaLM can explain jokes, for example, and has a rudimentary ability to think logically: Based on a few examples in the prompt (so-called "few-shot" learning), the model actually learns to explain its answer in a logical way. The researchers call this process "chain-of-thought prompting".
Shortly before PaLM was unveiled, Deepmind researchers presented the Chinchilla language model. The team studied the interaction between the model size in parameters and the amount of text data measured in the smallest processed unit, tokens.
Deepmind's Chinchilla shows: more data, better performance
While in recent years the focus of AI researchers has been on more parameters for better performance, Deepmind reduced the size of the network in Chinchilla and instead significantly increased the amount of training data. Since in AI training, the computational power required depends on the model size and training tokens, it remained on par with the AI language model Gopher, also from Deepmind.
Gopher has 280 billion parameters and was trained with 300 billion tokens. Chinchilla is four times smaller with only 70 billion parameters, but was trained with about four times more data - 1.3 trillion tokens.
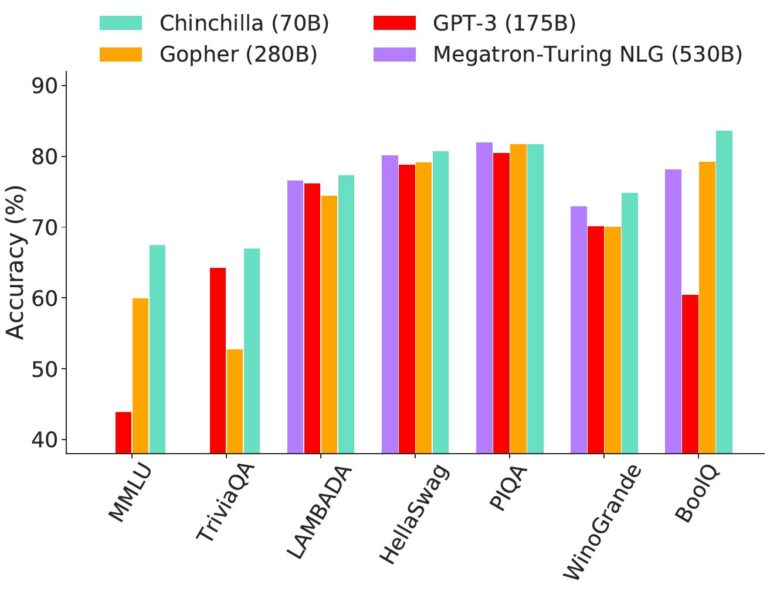
Despite the same training costs for Chinchilla and Gopher, the "tiny" AI model performs better than its predecessor in almost every speech task. Chinchilla even beats significantly larger language models like GPT-3 or the huge Megatron-Turing NLG model from Nvidia and Microsoft with 530 billion parameters. Only Google's PaLM with its 540 billion parameters and 768 billion training tokens performs better than Chinchilla.
Google's PaLM is massively under-trained
Deepmind's Chinchilla shows that giant AI language models are under-trained and that smaller AI models trained with lots of data can also achieve high performance. Smaller models like Chinchilla are also cheaper to run and can be optimized for specific use cases with little additional data.
With this approach, a "small" PaLM variant with 140 billion parameters could achieve the same performance as the large PaLM version with 540 billion parameters, according to Deepmind researchers. However: mini PaLM would require much more training data - a whopping three trillion training tokens instead of just 768 billion tokens.
Or, and this is likely already on Google's research agenda: Google accepts the higher training costs and trains the largest version of PaLM with significantly more data. Since the scaling curve of PaLM is similar to that of older language models such as GPT-3, the Deepmind research team assumes that the performance increase through scaling has not yet come to an end.
However, such a comprehensive language model requires more than ten trillion tokens for AI training, according to Deepmind - more than ten times the largest training dataset for language models to date.