Deepmind shows an AI system that learns intuitive physics. The team was inspired by insights from developmental psychology.
Artificial intelligence has cracked numerous benchmarks and conquered domains of human intelligence recently thanks to advances in hardware, network architectures and methods. However, despite successes such as AlphaGo, AlphaFold, GPT-3, and DALL-E 2, there is still a lack of what is often referred to as common sense.
There is a lively discussion in the AI research community about possible pathways to these capabilities, for example recently particularly prominent on Twitter between Meta's AI chief Yann LeCun and Gary Marcus.
A team at Deepmind is now turning its attention to a particular aspect of this debate, intuitive physics, in new research. In developmental psychology, intuitive physics often refers to the network of concepts that underlies our thinking about the properties and interactions of macroscopic objects.
This physical understanding is fundamental to embodied intelligence, as it is fundamental to all action in the environment. It also forms the basis for conceptual knowledge and compositional representations in general.
Deepmind's PLATO and the violation of expectation paradigm
In its new work, the team draws on key insights and methods from developmental psychology on intuitive physics: for example, physics is understood at the level of discrete objects and their interactions, the researchers write in their paper.
These allow for the formation of five concepts:
- continuity of objects,
- object permanence,
- solidity,
- immutability,
- and inertia in changes of direction.
If these concepts are present, a living being has formed an intuitive understanding of physics. In developmental psychology, these concepts are studied using what is known as the violation-of-expectation (VoE) paradigm.
The paradigm states that a person who possesses one of the concepts listed above has a set of expectations about the behavior of objects. For example, the concept of object permanence states that objects do not cease to exist when they disappear out of view.
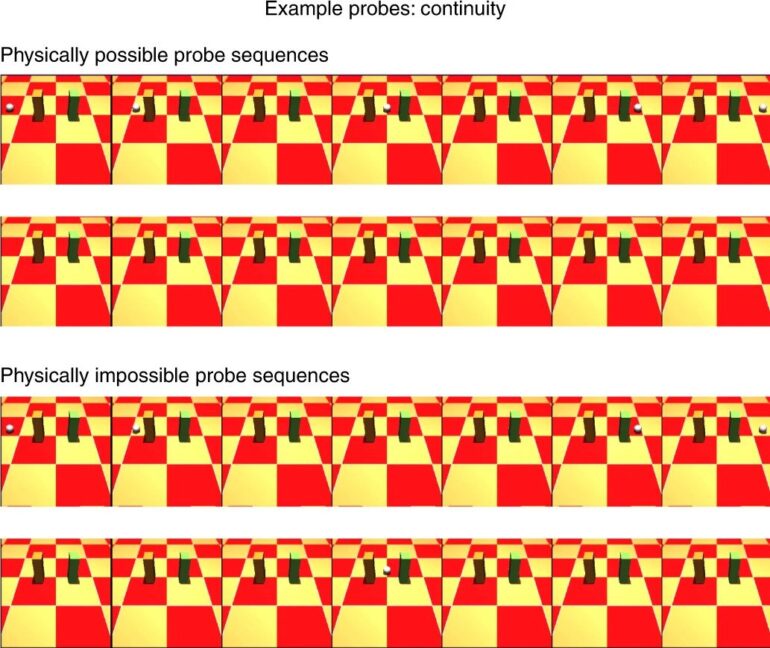
In experiments with infants or toddlers, for example, these expectations are broken in videos to determine whether the children are surprised. For example, if a toddler looks longer at an object after breaking the laws of physics, this is an indication of an expectation violation and thus of an existing concept of intuitive physics.
Deepmind's researchers trained PLATO (Physics Learning through Auto-encoding and Tracking Objects), a deep learning system that predicts the behavior of simple physical objects in videos. Deepmind then tested it with the expectation violation paradigm on the five previously mentioned concepts.
Deepmind generates 300,000 video clips of physical objects
To train PLATO, Deepmind created the Physical Concepts dataset, which consists of 300,000 short videos of simple, animated 3D objects, such as a ball rolling past behind an obstacle and reappearing on the other side.
PLATO consists of two components: a perception module that converts individual images into a series of object codes, and a dynamics predictor that predicts future images using the perception module's object codes.
The object codes here correspond to the representations of discrete objects known from developmental psychology, which serve as the basis of intuitive physics.
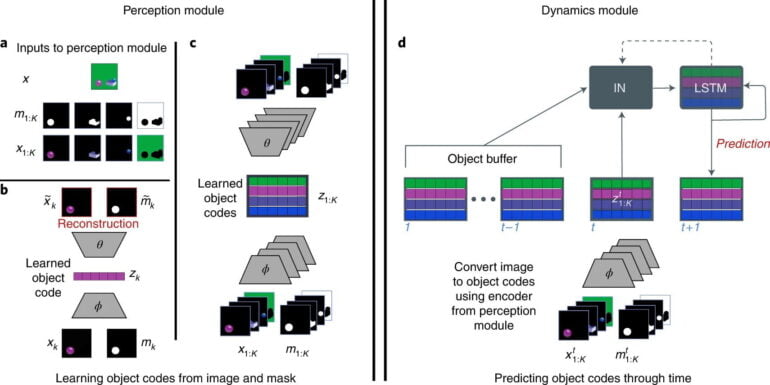
Thus equipped, PLATO could learn intuitive physics and at least some of the five concepts, the team surmised. After training, the researchers therefore tested PLATO with different VoE videos, i.e., short clips that verify certain concepts, such as when an object teleports in the field of view.
PLATO is based on physical objects
Each video that showed a violation of physical principles was contrasted with a corresponding video that was physically correct. This allowed the researchers to compare predictions. The team additionally trained variants of a second object-agnostic AI model that also predicts future images of the videos but does not use object codes.
In the tests, PLATO showed significant VoE effects in all five concept samples-the AI completions were strongly biased toward physical concepts and therefore did not match the video's progression in the VoE cases. In contrast, the alternative object-agnostic models without object codes did not produce results beyond chance.
In another experiment, the team also showed that similar results can already be achieved by training models with just 50,000 videos - equivalent to 28 hours of visual data. A possible indication that the human brain could also learn such concepts with visual observation.
Lead author Luis Piloto stresses, however, that PLATO was not designed as a model for infant behavior. It could, however, be a first step for an AI system that could test hypotheses about how human infants learn.