Deepmind introduces a new research framework for AI agents in simulated environments such as video games that can interact more flexibly and naturally with humans.
AI systems have achieved great success in video games such as Dota or Starcraft, defeating human professional players. This is made possible by precise reward functions that are tuned to optimize game outcomes: Agents were trained using unique wins and losses calculated by computer code. Where such reward functions are possible, AI agents can sometimes achieve superhuman performance.
But often - especially for everyday human behaviors with open-ended outcomes - there is no such precise reward function.
For an AI agent to be able to "put a cup down nearby," for example, it would need to be able to deal with a variety of possibilities. There are different linguistic formulations of the same request, language-intrinsic ambiguities (what is "nearby"?), irrelevant factors (for example, the color of the cup), and many ways to fulfill the request.
Deepmind strives for more natural interaction between humans and AI
Deepmind researchers are now introducing a new learning and training paradigm to develop agents that can naturally interact with and learn from humans.
To achieve this, Deepmind relies on humans to come up with tasks that AI agents must perform in simulated environments. Deepmind uses the resulting data to optimize the agents. These agents, trained with human feedback, can better understand "fuzzy human concepts" and have "grounded and open-ended" interactions with humans, according to Deepmind.
While still in its infancy, this paradigm creates agents that can listen, talk, ask questions, navigate, search and retrieve, manipulate objects, and perform many other activities in real-time.
Deepmind
AI agents learn from humans in an interactive playhouse
For its new research framework, Deepmind developed an interactive 3D playhouse in which AI agents and human users can move freely as avatars and interact and cooperate with each other.
Any exchange between humans and avatars took place via natural language in a chat. Here, humans set the context by asking the agents tasks or questions. The following video shows some of these tasks and how (already trained) AI agents solve them.
In the Playhouse environment, the research team also collected interaction data for training with reinforcement learning. According to Deepmind, the dataset generated includes 25 years of real-time interactions between agents and hundreds of humans.
Learning from humans means learning for humans
For the advanced AI agents, Deepmind first cloned the behavior and interactions of human users in the playhouse. Without this prior, the AI agents would otherwise just act randomly and in ways that humans could not understand, Deepmind writes.
This initial behavior was then optimized by human feedback with reinforcement learning according to the classical trial-and-error principle. However, the reward or punishment was not based on a score, but humans evaluated whether the actions contributed to achieving the goal or not.
Based on these interactions, Deepmind then trained a reward model that could predict human preferences. This reward model served as a feedback mechanism for further optimization of the agents through reinforcement learning.
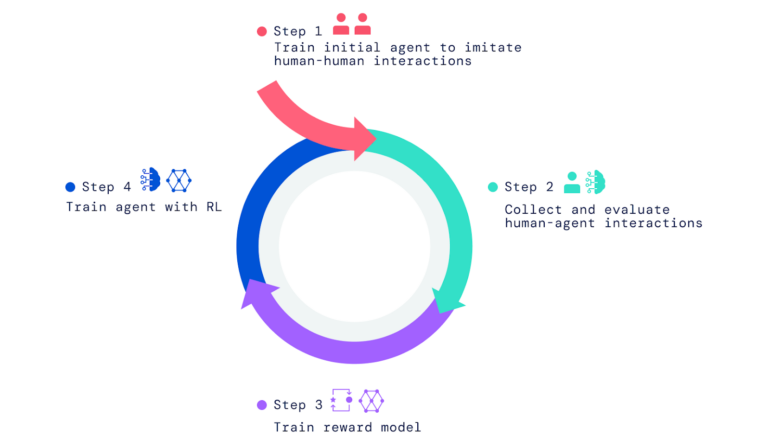
The tasks and questions for the learning process came from humans as well as from agents that mimicked human tasks and questions: one agent asked questions and tasks, the other responded.
Diverse and surprising behaviors
According to Deepmind, agents trained with human feedback can solve a variety of tasks that the team had not previously anticipated. For example, they arranged objects based on two alternating colors or brought users an object similar to the one they were currently holding.
These surprises emerge because language permits a nearly endless set of tasks and questions via the composition of simple meanings. Also, as researchers, we do not specify the details of agent behaviour. Instead, the hundreds of humans who engage in interactions came up with tasks and questions during the course of these interactions.
Deepmind
When evaluated with human users, AI agents trained with imitation learning and reinforcement learning performed significantly better than agents that only mastered imitation.
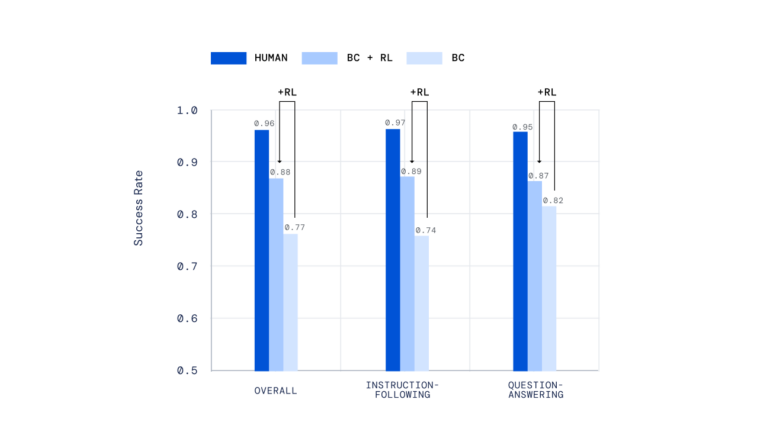
The training process could also be run multiple times to further optimize the agents with an updated reward model, the researchers say. Agents trained in this way were even able to outperform human users on average for some complex instructions.
Deepmind sees the presented framework as contributing to the development of AI agents for video games that can interact more naturally with humans, rather than just acting according to pre-programmed behaviors. The framework could also help in the development of digital or robotic assistants suitable for everyday use.
Humans as a benchmark for machine behavior
The integration of human feedback into the training of AI systems is seen by various institutions as a way to better adapt AI to the needs of humans. Deepmind, for example, has already presented a chatbot that incorporates human feedback into the training process. The research lab CarperAI plans to publish a corresponding open-source speech AI.
OpenAI sees human feedback in AI development as a central component of positive AI alignment. With the Instruct GPT models, OpenAI has already optimized large language models with human feedback that can generate human-preferred text despite having fewer parameters.