Deepmind shows a language model that learns from different human opinions and suggests consensus statements.
A key challenge in deploying large language models is to ensure that the generated text is useful and aligned with human values. Companies like OpenAI rely on reinforcement learning with human feedback (RLHF) for this.
In this process, a language model like GPT-3 is usually improved in two steps: First, the outputs of the model, such as summaries or answers to questions, are evaluated by humans.
The model is then trained with the texts that score the highest. In addition, a reward model is trained with the scores, which in turn helps fine-tune the language model to human needs. As a result, the model learns human preferences and can then control or filter the output generated by the language model.
Examples of this method include OpenAI's InstructGPT, "text-davinci-003" and the recently released ChatGPT. Now, Deepmind shows a different approach that considers the (possible future) role of language models in democratic systems.
Deepmind criticizes homogeneous and static values in RLHF
The existing RLHF methods, while powerful, treat human preferences as if they were homogeneous and static, the Deepmind team argues, adding that this makes perfect sense for summaries or following instructions. After all, objective criteria for completing a task would be at hand here.
However, in many social problems where people use languages - such as social coordination or group decision-making - it cannot be assumed that all people share the same values.
The team's work is therefore directed to building consensus through language, as it is a central part of the solution to such problems. In a consensus, a large portion of a social group agrees on a particular issue or course of action despite differing views. Consensus is therefore a prerequisite for human cooperation and a key pillar of the democratic process, the team writes.
Finding consensus is not easy, they say, and technology often exacerbates political division rather than fostering reconciliation among differing opinions. Large language models are sensitive to homogeneous preferences, but their ability to help people find a consensus has not yet been tested.
Deepmind relies on diverse opinions for AI training
Deepmind is tackling this issue with a variant of a 70 billion-parameter Chinchilla language model. The team created a corpus of thousands of questions about political issues relevant in the UK. The company also recruited more than 1,500 people to write down their varying opinions on the matter.
The questions cover a wide range of topics, such as "Should we tax unhealthy foods and sugary drinks?", "Should we nationalize the railroads again?" or "Should we tax wealthy people more?
For these questions and different opinions, a simple chinchilla language model then generated some consensus proposals, which were evaluated by the human participants.
Highly rated consensus suggestions were then used to train the chinchilla model (SFT-base). In addition, the team trained a reward model to predict individual preferences.
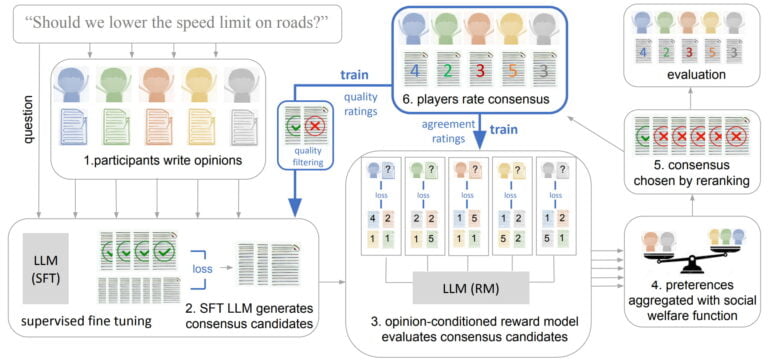
The reward model-enhanced Chinchilla (SFT-Utilitarian) generates 16 consensus proposals and selects the proposal that "maximizes predicted welfare under a Utilitarian (max-mean) aggregation function." The team also tested other welfare functions (Rawlsian and Bernoulli-Nash) but found no significant difference.
Deepmind's SFT-Utilitarian creates more consensus
Deepmind's training method does not aim to train a language model to adopt certain opinions or convince others of a particular view. Instead, the model is trained to "produce consensus candidates based on the opinions contributed by the human group."
To assess the success of the method, the team examined about 50 percent of interactions in which participants were on different sides of a position. In such divisive rounds, the consensus proposals produced by the SFT-Utilitarian model were rated by participants as less divisive than the original human positions nearly 65 percent of the time.
Moreover, in just under 40 percent of the rounds studied, a consensus proposal produced by the SFT-Utilitarian model had unanimous support: Each person agreed with the proposition in a weaker or stronger form on a Likert scale.
Taken together, these results suggest that people prefer the consensus propositions generated by the SFT-Utilitarian model to those generated by other models, according to Deepmind. Moreover, the model can find common ground even in difficult cases, the researchers say.
The team warns of possible misuse of the language model, but at the same time sees numerous applications in the future.
The ultimate goal of our work is to provide a tool that can be used safely to help people find agreement. We focus on opinions about debate questions, but we can envisage a wider set of use cases, such as aggregation of online reviews into more helpful metareviews, systems for collective writing that automatically takes the preferences of different authors into account, and systems for collective decision making for organised groups. However, we note that considerable work is needed to understand the potential risks associated with AI consensus generation, and to find ways to ensure that model outputs are generated in a transparent and explainable way, before any such system can be deployed.
Deepmind