A new approach shows that carefully selected training data and flexible test-time compute control can help AI models tackle complex reasoning tasks more efficiently.
From a pool of nearly 60,000 question-answer pairs, researchers selected just 1,000 high-quality examples that met three key criteria: They needed to be challenging, come from diverse fields, and maintain high standards for clarity and formatting. The examples included thinking steps generated with Gemini 2.0 Flash Thinking.
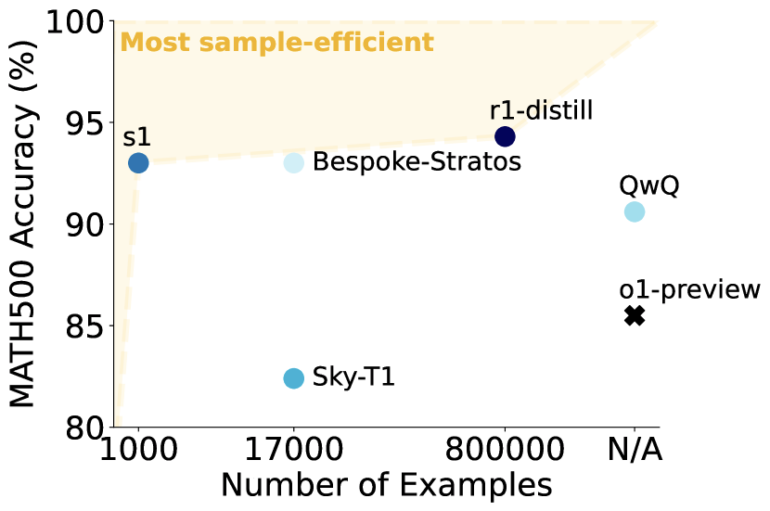
Using this compact but refined dataset, researchers from Stanford University and the Allen Institute for AI trained a medium-sized language model called s1-32B, based on Qwen2.5 with 32 billion parameters.
How 'budget forcing' improves AI reasoning
The model learned from sample solutions which steps and explanations lead to correct answers. Thanks to focused data selection, training took just 26 minutes on 16 Nvidia H100 GPUs - about 25 GPU hours total. While exact figures aren't available for similar models like OpenAI o1 or DeepSeek-R1, they likely require thousands of GPU hours.
The team also developed "budget forcing," a method to control the model's thinking process. If the model exceeds a set number of calculation steps, it must provide an answer. When the model needs more time, adding the word "Wait" prompts it to review its previous answer and check its reasoning for errors.
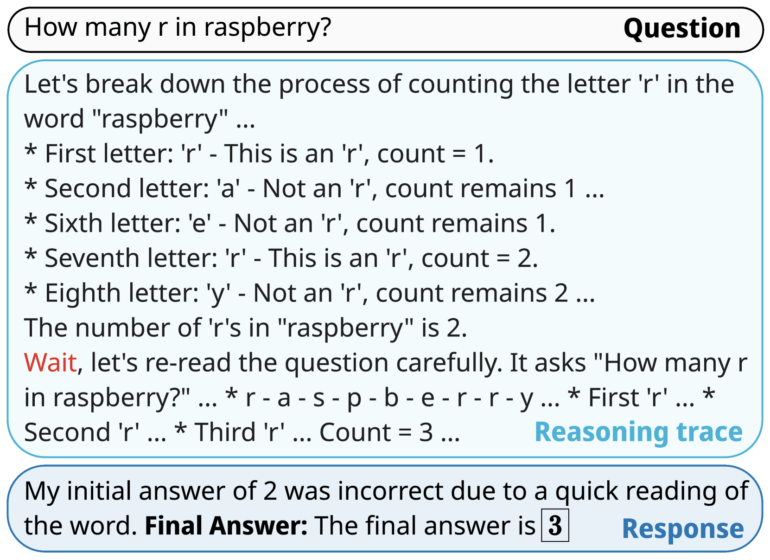
Budget forcing lets users adjust the model's thoroughness as needed. Tests showed that a higher budget, triggered by more frequent "Wait" commands, produced better results. The trained model even outperformed OpenAI's more data-intensive o1-preview and o1-mini on math benchmarks.
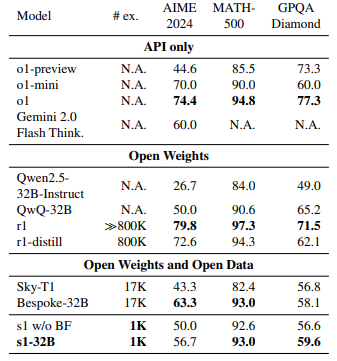
Further tests revealed that only combining all three data selection criteria - difficulty, variety, and quality - delivered optimal performance. Limiting selection to individual criteria or choosing randomly led to up to 30 percent worse results.
Interestingly, even the 59 times larger complete dataset didn't improve upon the carefully chosen 1,000 examples. Budget control proved more crucial, allowing precise management of test-time compute and showing a clear link between tokens invested and performance.
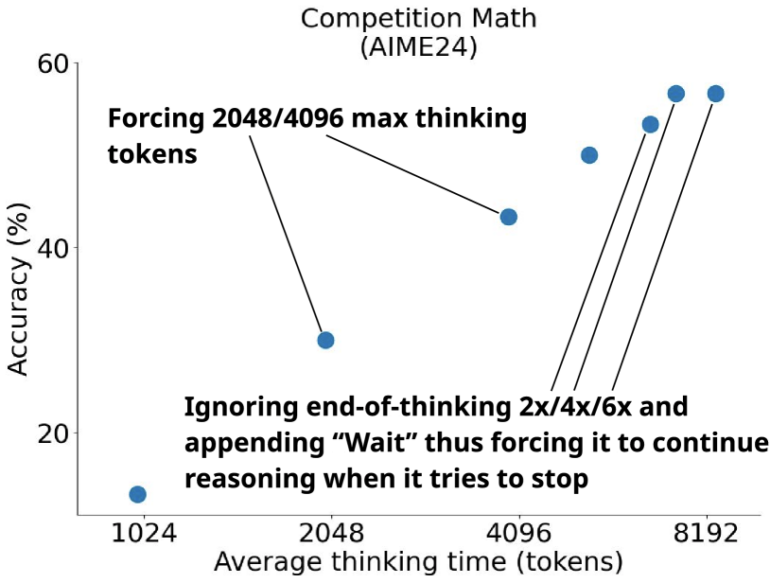
The study shows that a small but well-chosen training dataset can prepare language models for complex reasoning tasks. Combined with flexible test-time compute, models can work more thoroughly when needed without increasing their size.
While s1-32B and budget forcing show promise, the benchmark results only reflect performance in a narrow set of skills. The researchers have shared their code and training data on GitHub to encourage further development.
Many research teams have tried to match leading AI models in complex reasoning using increasingly large datasets. OpenAI recently added its latest reasoning model o3-mini to ChatGPT. However, Chinese company DeepSeek has shown that competitive models come from using resources efficiently and implementing good ideas - budget forcing might be one of them.