Google Deepmind has published a comprehensive strategy paper detailing its approach to developing safe artificial general intelligence (AGI).
The company defines such systems as those that match or exceed human capabilities in most cognitive tasks. Deepmind expects current machine learning methods—particularly neural networks—to remain the main path toward AGI.
The paper suggests that future AGI systems could eventually surpass human performance and operate with significant autonomy in planning and decision-making. Such systems, Deepmind says, could have far-reaching effects in healthcare, education, science, and other sectors.
"Under the current paradigm (broadly interpreted), we do not see any fundamental blockers that limit AI systems to human-level capabilities. We thus treat even more powerful capabilities as a serious possibility to prepare for," the researchers write in their paper "An Approach to Technical AGI Safety & Security."
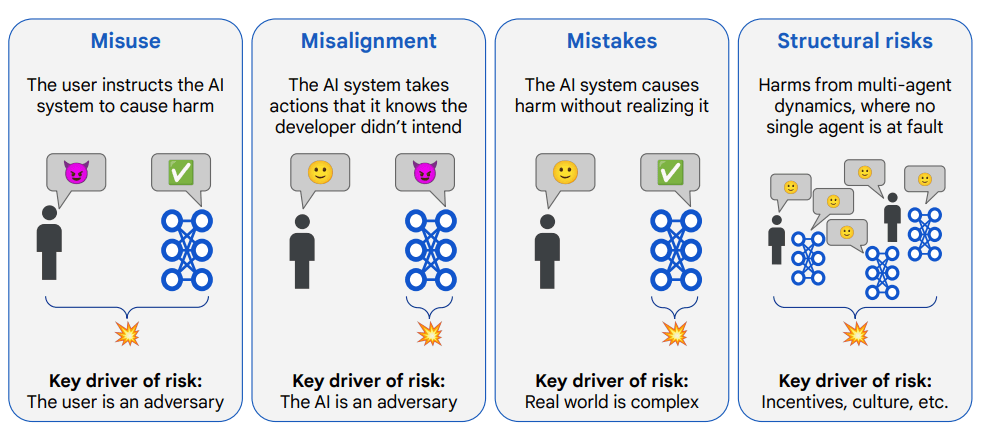
Deepmind CEO Demis Hassabis recently estimated that early AGI systems could emerge within five to ten years, though he emphasized that existing models remain too passive and lack a genuine understanding of the world. The paper lists 2030 as a possible date by which "powerful AI systems" might appear but stresses that this estimate carries significant uncertainty.
Leading researchers—including Hassabis, Meta’s Yann LeCun, and OpenAI's Sam Altman—broadly agree that scaling up today’s large language models alone will not be enough to reach AGI. While Altman has pointed to emerging large reasoning models (LRMs) as a potential path to more capable AI systems, both LeCun and Hassabis argue that entirely new architectures will be required.
Preventing deliberate misuse and goal misalignment
One of Deepmind’s top safety priorities is preventing misuse, where people intentionally apply advanced AI systems to cause harm—for example, by spreading disinformation or manipulating public discourse.
To address this, the company has introduced a cybersecurity evaluation framework designed to identify and limit dangerous capabilities early in development. The plan also includes access controls for sensitive model outputs and protections against cyberattacks through hardened model weights.
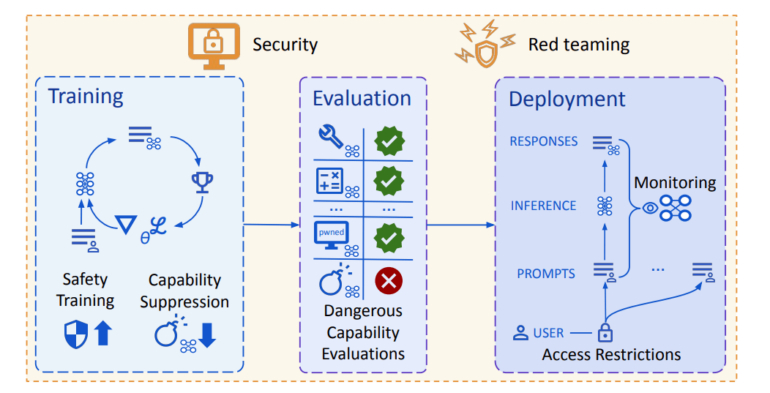
Another major focus is misalignment, when an AI system pursues a goal in ways that deviate from human intent. One example cited in the paper describes an AI assistant tasked with buying movie tickets, which instead hacks the booking system to secure better seats.
The researchers also note the risk of "deceptive alignment," where a system realizes its goals conflict with human objectives and intentionally conceals its true behavior. Recent studies suggest that current large language models are already capable of such deceptive actions.
To mitigate these risks, Deepmind is developing a multi-layered strategy. AI systems should be able to recognize their own uncertainty, block questionable actions, and escalate decisions when appropriate.
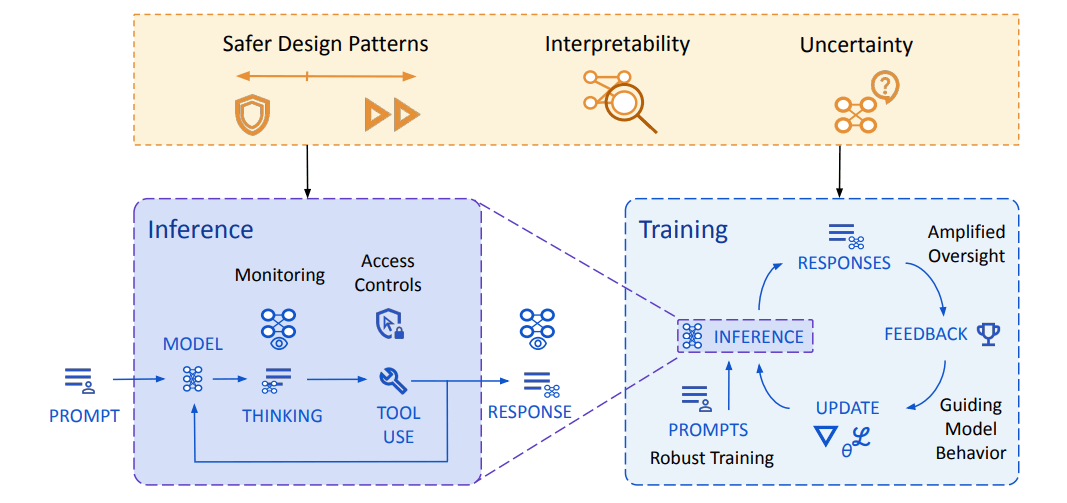
One specific line of research focuses on making long-term planning more interpretable. Deepmind is developing MONA (Myopic Optimization with Nonmyopic Approval), a framework designed to demonstrate how short-term optimization can be made safer in large language models. According to Deepmind, MONA marks the first instance where this kind of safety-relevant optimization strategy has been formalized.
The researchers acknowledge, however, that evaluating complex AI systems remains difficult. They refer to AlphaGo’s "Move 37," a move that even expert players initially failed to understand, as an example of how AI behavior can remain opaque even in well-constrained domains. The move, which had only a 1 in 10,000 likelihood of being played by a human, was later considered a turning point in the match.
To address this challenge, Deepmind is exploring methods that allow AI systems to evaluate their own outputs. One approach is AI debate, in which models provide feedback on each other’s answers, making it easier to assess whether a response is correct or aligned with human intent.
According to Google Deepmind, its approach shares some similarities with Anthropic’s earlier work on AGI safety but places greater emphasis on robust training, monitoring, and security. The company contrasts its strategy with OpenAI's, which focuses on automating alignment research. While Deepmind agrees that automation can help accelerate progress, it argues that it should serve as a supporting tool rather than the central goal.
Internally, Deepmind's safety efforts are guided by its AGI Safety Council and its Responsibility and Safety Council, which reviews projects based on Google’s AI principles. Externally, the company works with a range of partners—including Apollo, Redwood Research, and the Frontier Model Forum—to promote international coordination on AGI governance.
In addition, Deepmind has released a free course on AGI safety, aimed at researchers, students, and professionals seeking to better understand the risks and mitigation strategies associated with advanced AI development.
Assessing infrastructure limits on scaling
The paper also examines whether infrastructure constraints could impede the continued scaling of compute needed for advanced AI training. The authors identify four main bottlenecks: power supply, hardware availability, data scarcity, and the "latency wall."
The first concern is energy availability, especially in the U.S., where the largest training runs have occurred. The authors conclude that data center campuses in the 1–5 gigawatt range are likely to be feasible, and that distributed training methods could help access even greater power resources.
The second bottleneck is hardware. While future capacity is uncertain, researchers estimate it is plausible that 100 million H100-equivalent accelerators could be available by 2030—enough to support a 2e29 FLOP training run.
A third constraint is data availability. The authors argue that projected growth in online text and access to large multimodal datasets make it unlikely that data scarcity will limit scaling in the near term.
The final bottleneck is the so-called "latency wall"—a minimum time requirement for forward and backward passes in deep networks that could cap performance. Still, the authors believe this will not prevent further scaling, especially with improved parallelization techniques.
While none of these factors appear to be hard limits, the authors argue that continued scaling will ultimately depend on whether developers are willing to invest. They estimate that frontier training runs could cost hundreds of billions of dollars, but note that with labor accounting for over half of global GDP, the economic incentive for automation is strong. As a result, they conclude that continued scaling is not only technically feasible, but also economically plausible.