Google Deepmind researchers say the ability to act in an open-ended way is crucial for developing superintelligent AI.
Using ever-larger datasets is not enough to achieve ASI, the researchers say. They are referring to current scaling strategies, which tend to focus on using more computing power and more data. Instead, AI systems must be able to generate new knowledge and improve their learning capabilities on their own in an open-ended way.
The researchers define an AI system as "open-ended" if it constantly produces new and learnable artifacts that are difficult to predict, even if an observer has become better at predicting them by studying past artifacts.
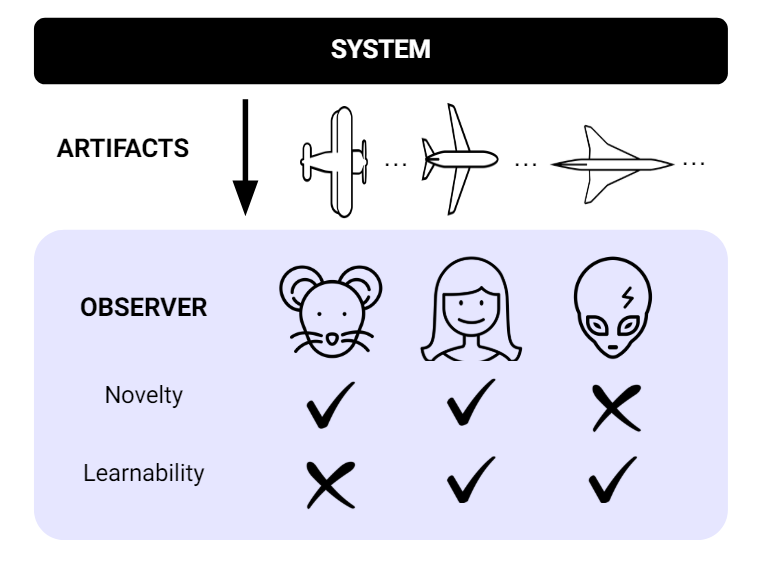
The researchers give some examples of open systems and their limitations. AlphaGo can constantly develop new strategies to beat learning humans - but only within the rules set by humans. Despite its openness, such a system is too narrow to discover new science or technology, the researchers say.
Another example is the "AdA" agent, which solves tasks in a 3D world with billions of possible variations. Through training, AdA gradually gains skills for increasingly complex and unfamiliar environments.
To human observers, this seems open-ended, as AdA keeps showing new abilities. However, the results suggest that the novelty reaches a limit over time and can only be reactivated by adding more content. So openness is limited in time.
The POET system trains agents that are each connected to an evolving environment. By switching between environments, the agents eventually solve very complex tasks that could not be solved by direct optimization.
However, this openness is limited by the settings of the environment itself: At some point POET, like AdA, reaches a limit.
Foundation models based on passive data will soon hit a wall
In contrast, current foundation models, such as large language models, are not "open-ended" because they are trained on static datasets; they would eventually stop producing new artifacts. They will "soon plateau," according to the paper.
However, they could lead the way to open systems if they were continually fed new data and combined with open-ended methods such as reinforcement learning, self-improvement, automatic task generation, and evolutionary algorithms.
Foundation models would bring together human knowledge and could guide the search for new relevant artifacts, the researchers say. This makes them a possible building block for AI's ability to generalize.
"Our position is that open-endedness is a property of any ASI, and that foundation models provide the missing ingredient required for domain-general open-endedness. Further, we believe that there may be only a few remaining steps required to achieve open-endedness with foundation models."
From the paper
The scientists also warn of major safety risks. The artifacts generated must remain understandable and controllable by humans. Safety must be built into the design of open-ended systems from the outset to prevent a superintelligent AI from getting out of control.
But if the challenges can be overcome, the researchers believe that open-ended foundation models could enable major breakthroughs in science and technology.
Deepmind co-founder Demis Hassabis hinted a year ago that Google Deepmind was testing combining the strengths of systems like AlphaGo with the knowledge of large models.
However, the Gemini model that Google has released so far does not yet offer such breakthrough innovations, and it can barely keep up with OpenAI's GPT-4.
OpenAI's Q* also likely aims to combine ideas like those known from Google Deepmind's AlphaZero with language models - similar to what Microsoft researchers recently showed with "Everything of Thought."