With a new dataset, Deepmind has partnered with many other institutions to fill the data gap in robot training and enable generalization of robot capabilities across robot types. Early results are promising.
Google Deepmind, in collaboration with 33 academic labs, has released a new dataset and models aimed at promoting generalized learning in robotics across different types of robots.
The data comes from 22 different types of robots. The goal is to develop robot models that can better generalize their capabilities across different types of robots.
Toward a general-purpose robot
Until now, a separate robot model had to be trained for each task, each robot, and each environment. This places high demands on data collection. In addition, the slightest change in a variable meant that the process had to start all over again, according to Deepmind.
The goal of the Open-X initiative is to find a way to pool knowledge about different robots (embodiments) and train a universal robot. This idea led to the development of the Open-X embodiment dataset and RT-1-X, a robot transformer model derived from RT-1 (Robotic Transformer-1) and trained on the new dataset.
Tests in five different research labs showed an average 50 percent increase in task completion success when RT-1-X took control of five common robots compared to the robot-specific control models.
A dataset for general-purpose robot training
The Open X Embodiment dataset was developed in collaboration with academic research labs from more than 20 institutions. It aggregates data from 22 robots representing more than 500 capabilities and 150,000 tasks in more than one million workflows.
The dataset is an important tool for training a generalist model that can control many types of robots, interpret different instructions, make basic inferences about complex tasks, and generalize efficiently, according to Deepmind.
Emergent capabilities in robot model
The RT-2 version of the visual language action model RT-2-X, introduced this summer, tripled its capabilities as a potential real-world robot after being trained with the Open-X dataset. The experiments showed that co-training with data from other platforms gave RT-2-X additional capabilities not present in the original RT-2 dataset.
The RT-2 model uses large language models for reasoning and as the basis for its actions. For example, it can reason why a rock is a better improvised hammer than a piece of paper, and apply this ability to different application scenarios.
After training on the X dataset, it was able to improve these skills. For example, RT-2-X showed a better understanding of spatial relationships between objects, being able to distinguish fine gradations such as "put the apple on the cloth" or "put the apple near the cloth".
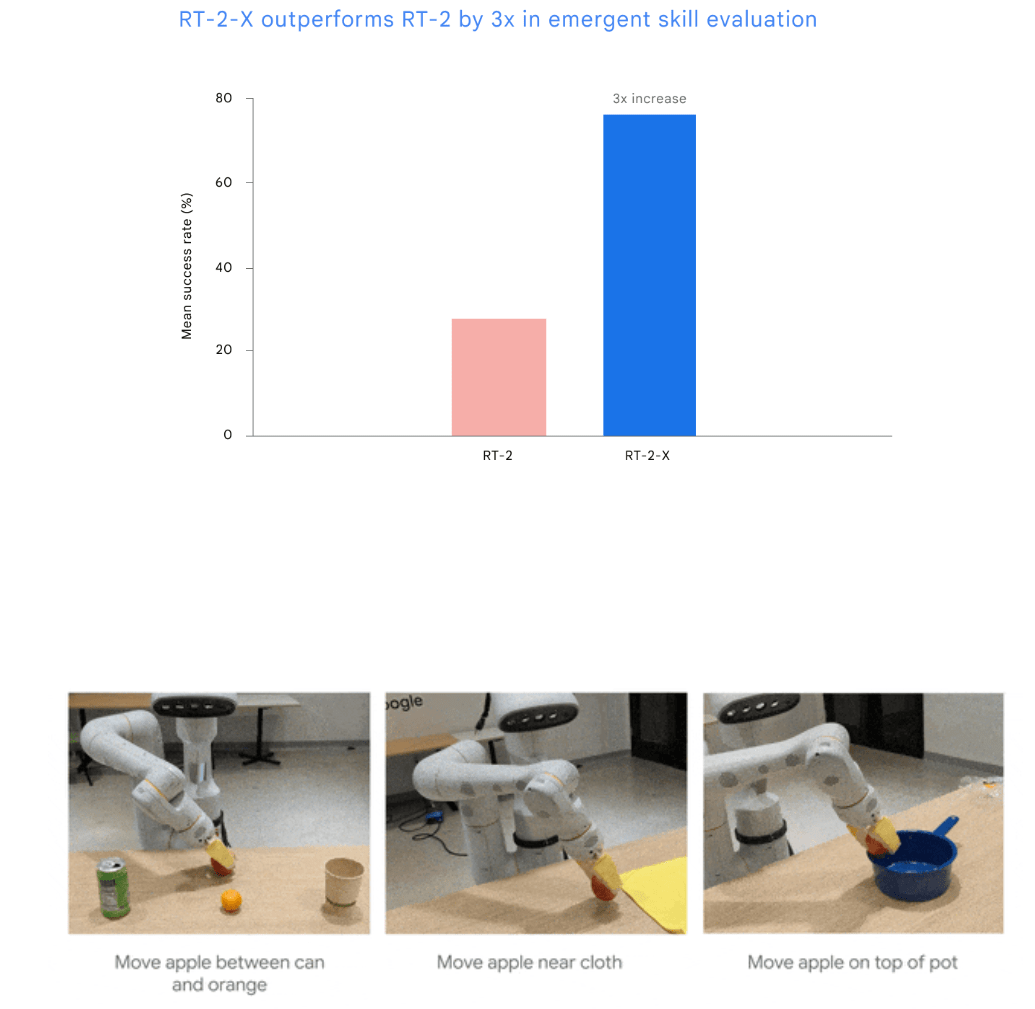
RT-2-X shows that training with data from other robots can also improve already capable robots that have been trained with lots of data, Deepmind writes.
The research team's conclusion is similar: scaling robot capabilities using data from different types of robots works, they say, and yields "dramatic performance improvements." Future research could look at how robot models can better learn from experience to improve themselves.