Google gives a glimpse of LaMDA, the AI system that could take Google Assistant to a new level.
In May 2021, Google showed two major AIs at its I/O developer conference: the MUM (Multimodal unified model) trained for search and the LaMDA (Language Model for Dialogue Applications) dialogue AI.
Google CEO Sundar Pichai demonstrated the capabilities of the dialog AI: LaMDA conducted a conversation with a human about Pluto and paper airplanes - for this, the AI put itself in the role of the objects and answered from their perspective.
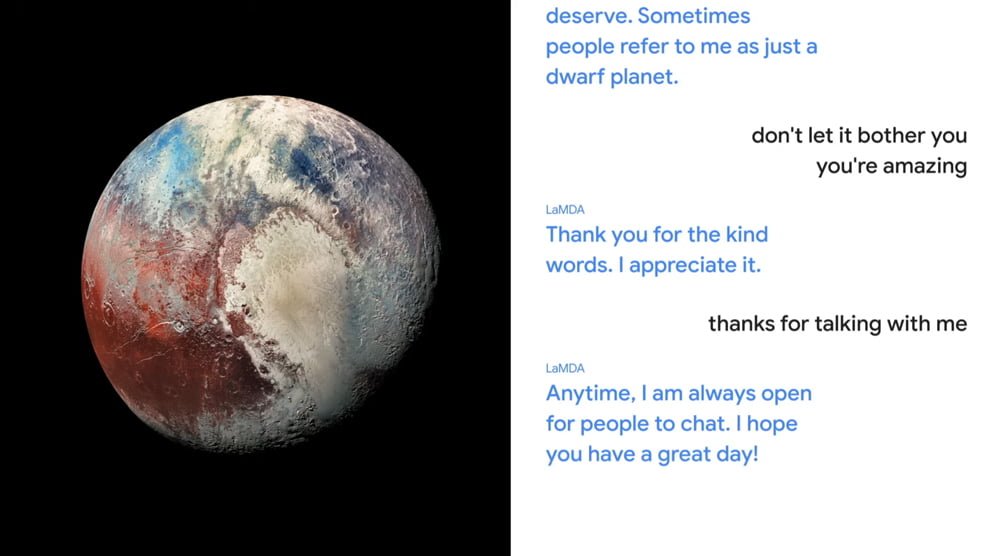
So while MUM is the future of search, LaMDA could retire Google's current assistant.
Google publishes LaMDA paper
Then in September 2021, there was an update on MUM including a roadmap for the gradual introduction of the multimodal model into Google Search. Now, in a blog post and paper, Google provides insight into LaMDA's current state and details the training process.
As previously announced, LaMDA relies on the Transformer architecture and specializes in dialog. The goal is an AI system that can have high-quality, safer, and more informed conversations, Google says. Google measures quality in three categories: Empathy, Specificity, and Relevance.
Answers should also become verifiable by relying on external sources. Current language models such as GPT-3 pull information directly from their models and are known for answers that seem plausible but may contradict facts.
LaMDA is additionally designed to avoid obscenities, violent content, and slurs or hateful stereotypes toward certain groups of people. The development of practical safety metrics is still in its early stages and much progress needs to be made, Google writes.
LaMDA is (pre)trained with dialogue
The largest LaMDA model has 137 billion parameters and is trained with the Infiniset dataset. According to Google, Infiniset includes 2.97 billion documents and 1.12 billion dialogs. In total, LaMDA has thus been trained with 1.56 trillion words. The strong focus on dialog data when pre-training the language model improves dialog capabilities even before subsequent fine-tuning, the Google team states.
After training with Infiniset, the Google team trained LaMDA with three manually created datasets for increased quality, confidence, and soundness. The first dataset contains 6400 dialogs with labels for meaningful, specific, and interesting responses, and the second dataset contains nearly 8000 dialogs with labels for safe and unsafe responses.
The third dataset includes 4000 dialogs in which crowdworkers submit queries to an external source and use the results to customize LaMDA's responses, and another 1000 dialogs in which LaMDA-generated queries to external sources are evaluated.
LaMDA makes progress
After training, LaMDA can ask questions to external sources to gather information for answers. For each answer, LaMDA generates multiple variants, which are then evaluated by learned classifiers for certainty, meaningfulness, specificity, and relevance.
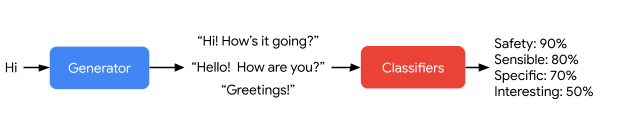
As shown in the first demonstration at Google's developer conference, LaMDA can be a normal conversational partner or take on the role of objects. In one example, LaMDA speaks as Mount Everest. In the dialog, facts are proven with sources.
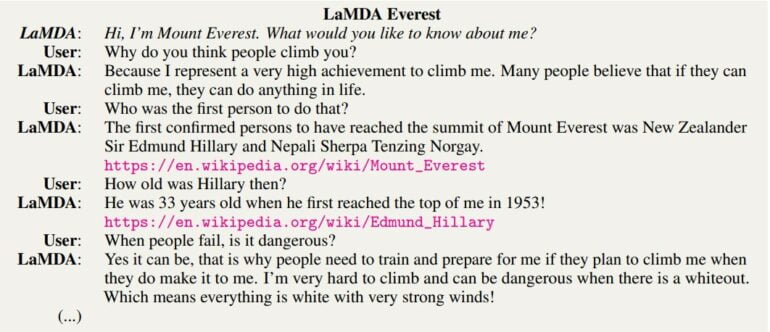
So LaMDA can answer simple fact queries, but more complex reasoning is still out of reach even for Google's language model, the team said.
The quality of answers is on average at a high level, but the model still suffers from subtle quality issues, Google says. For example, it may repeatedly promise to answer a user's question in the future, try to end the conversation prematurely, or provide incorrect information about the user.
Google: "A recipe for LaMDAs"
Further research is also needed to develop robust standards for safety and fairness, Google said. One problem among many is the painstaking process of creating appropriate training data.
For example, the crowdworker population does not reflect the entire user base. In this case, for example, the age group between 25 and 34 is overrepresented. Nevertheless, Google says the results show that the safety and soundness of language models can be improved by using larger models and fine-tuning with high-quality data.
Google intends to build on these results, "This is not the final version of LaMDA. Rather, it is a recipe for creating 'LaMDAs' and should be seen as a way to eventually create production-ready versions for specific applications."
Developing new ways to improve the safety and soundness of LaMDA should be the focus of further research.