ImageReward is designed to improve the results of generative AI models like Stable Diffusion and has been trained with human feedback.
Generative AI models for text-to-image have developed rapidly, with paid services like Midjourney or open-source models like Stable Diffusion leading the way. Central to this boom was OpenAI's first DALL-E model, which served as a blueprint for models to come: a generative AI model produces images, and another AI model evaluates how close those images are to the text description.
This task was performed by OpenAI's CLIP, variants of which are still used in current AI systems based on diffusion models. In a new paper, researchers now demonstrate a text-image scoring method inspired by reinforcement learning with human feedback that relies on alternatives such as CLIP, Aesthetic, or BLIP for better image synthesis.
ImageReward improves Stable Diffusion image quality
The team from Tsinghua University and Beijing University of Posts and Telecommunications trained the ImageReward reward model using human feedback. The text-image scoring method learned from 137,000 examples and 8,878 prompts and outperformed CLIP, Aesthetic, or BLIP by 30 to nearly 40 percent on various benchmarks.
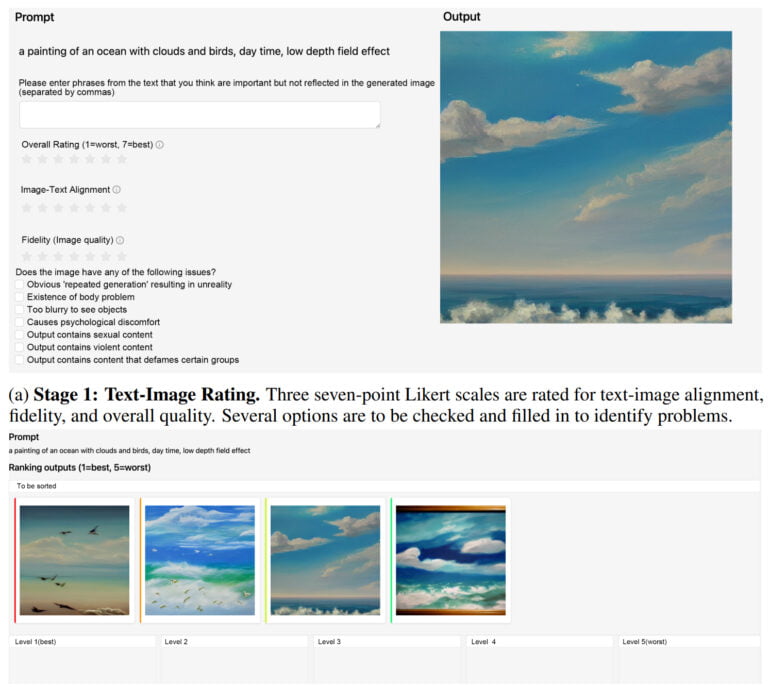
In practice, ImageReward achieves better alignment of text and images, reduces distorted renderings of bodies, better matches human aesthetic preferences, and reduces toxicity and bias. The team shows how ImageReward affects image quality in a few examples, where they let the different text-image scorers let select the Top-1 images out of 64 generations.
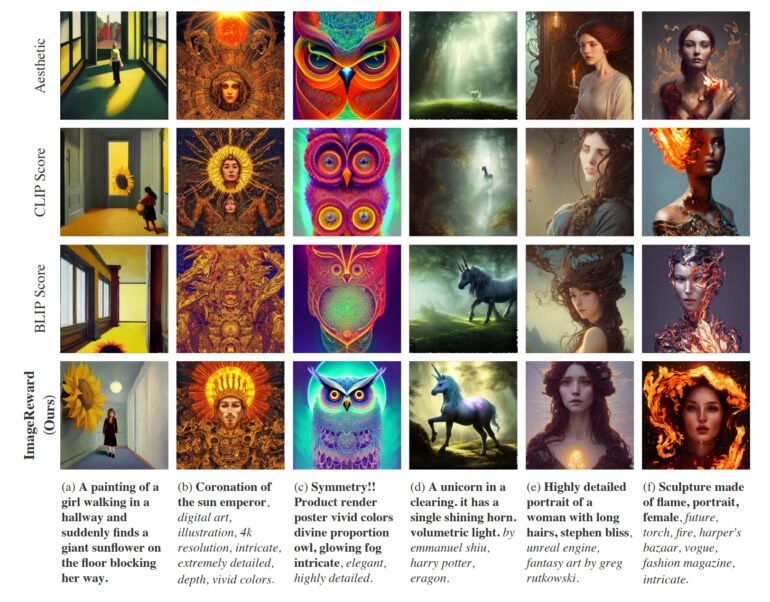
ImageReward available for Stable Diffusion WebUI
According to the team, future work will require a larger dataset to better train the reward model and more varied prompts to reflect the diverse needs of human users. In addition, ImageReward can currently only be used after the fact as a filter for images that have already been generated - similar to CLIP in the first DALL-E model. The widely used diffusion models do not appear to be inherently compatible with current RLHF methods, according to the team.
However, they hope to work with the research community in the future to find ways to use ImageReward as a true reward model in RLHF for text-to-image models.
ImageReward is available from GitHub. There are also instructions there on how to integrate ImageReward into the Stable Diffusion WebUI.