Neural Radiance Fields (NeRFs) are a promising graphics technology that can transform the real world into 3D relatively quickly and with high quality. LERF (Language Embedded Radiance Fields) integrates the capabilities of large language models into NeRFs. This enables accurate 3D object recognition without additional training.
UC Berkeley researchers present LERF, which volumetrically integrates CLIP vectors into the 3D environment of a NeRF. LERF extracts 3D relevance maps from the NeRF environment. These maps can then be searched using natural language.
For example, a user can search for a specific book title in the NeRF environment of a bookstore. LERF can identify and tag that book in the environment with pixel accuracy on the first try (zero shot). According to the researchers, the technology requires no region proposals, masks, or fine tuning.
A NeRF of a bookstore becomes searchable via natural language thanks to LERF. | Video: Kerr et al.
Google, for example, is working on integrating NeRFs of real places like restaurants or stores into Google Maps. Using LERF technology, these scanned real-world locations could be searched virtually at lightning speed.
However, LERFs are still static, so for a real-time search for the nearest supermarket, a multimodal search using normal 2D webcam images would be more appropriate. For a guided VR tour of a real store, however, the combination of LERF and NeRF would be sufficient. In addition to Google, Meta is also researching NeRFs to allow users to bring real objects into digital worlds using smartphone scans.
Large language models interact with the digitized real world through NeRFs
LERF bridges the gap between large language models and digital worlds that, in the case of NeRFS, can be very close to reality.
In one experiment, the research team used ChatGPT to generate a list of tasks for cleaning up a kitchen where coffee had been spilled. All of the actions suggested by ChatGPT could be mapped to areas and objects relevant to the steps in a kitchen NeRF using LERF's 3D relevance map.
For example, LERF detects the paper towel over the sink (center), which ChatGPT thinks should be used first to wipe up as much of the spilled coffee as possible. The spilled coffee (far right) is also marked in the image, but with less relevance when searching for the paper towel.
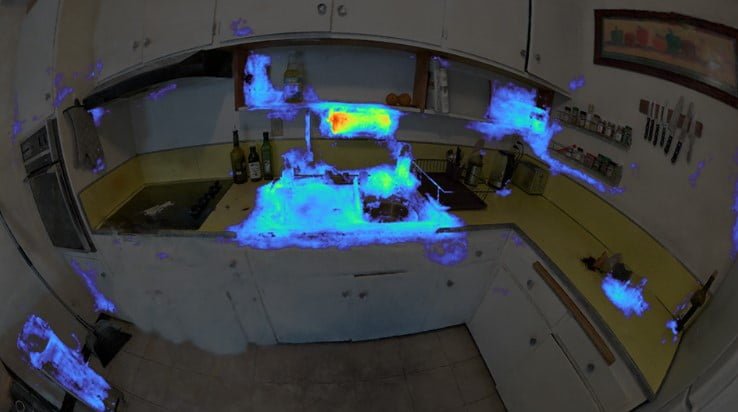
What's special about using natural language is that you can search a 3D scene for many different features: Color, shape, function, name of an object, or even brands. The system can even distinguish between different types of donuts, such as chocolate and blueberry.
Video: Kerr et al.
The research team sees potential applications in robotics, such as visual robot training in simulations, better understanding of the capabilities of visual-language models, and interacting with and in 3D worlds.
The team plans to integrate LERF into the open source NeRF software "Nerfstudio". More information and examples are available on the project page lerf.io.