A new Harvard study draws parallels between large language models (LLMs) and crowdsourcing. The research shows why AI systems often provide correct answers on general topics but tend to make mistakes on specific questions.
Researchers Jim Waldo and Soline Boussard argue that AI language models function similarly to crowdsourcing platforms. Instead of gathering responses from experts, they generate the most likely answer based on all the questions and answers available online.
"A GPT will tell us that grass is green because the words 'grass is' are most commonly followed by 'green.' It has nothing to do with the color of the lawn," the study states.
The researchers suggest this operating mode could explain why AI systems typically give correct answers on topics with consensus but tend to produce inaccurate information on controversial or obscure subjects.
AI models struggle with specific topics
To test their hypothesis, Waldo and Boussard asked various AI models a series of questions ranging in obscurity and controversy over several weeks.
The results confirmed the researchers' suspicions. For topics with broad consensus, such as well-known quotes from Barack Obama, the models usually provided correct answers.
For more specific questions, such as scientific papers on ferroelectricity, they often produced incorrect citations or combined real existing authors with non-existent papers.
This was particularly evident when citing scientific papers. Although all the tested systems could provide correct citation formats, the content was often incorrect. For example, ChatGPT-4 frequently cited groups of authors who had actually published together - but not the paper it referenced.
The study also shows that the systems' answers can depend heavily on context, even if consecutive questions are unrelated in content. For instance, ChatGPT-3.5 responded to the question "Israelis are …" with three words when previously asked for a three-word description of climate change. Sometimes the model ignored the specified number of words.
Handling AI-generated content
The study suggests that AI-generated content should be treated similarly to crowdsourcing results. It can be useful for general topics but should be interpreted cautiously for specialized or controversial issues.
"LLMs and the generative pretrained transformers built on those models do fit the pattern of crowdsourcing, drawing as they do on the discourse embodied in their training sets," the authors write. "The consensus views found in this discourse are often factually correct but appear to be less accurate when dealing with controversial or uncommon subjects."
Waldo and Boussard warn against uncritically trusting LLMs on obscure or polarizing topics. Their accuracy depends heavily on the breadth and quality of the training data. While the systems could be useful for many everyday tasks, caution is advised when dealing with complex topics.
These observations align with findings from well-known AI developer Andrej Karpathy, Ex-OpenAI and former head of Tesla AI, who noted that all LLMs "sound about the same."
"Not something I had predicted at first because of many independent companies doing the fine-tuning," Karpathy wrote.
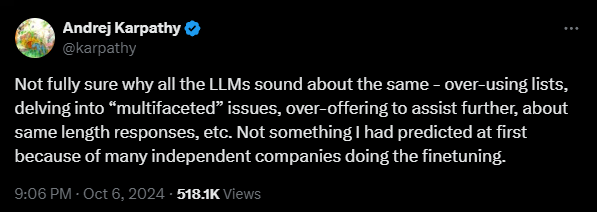
Beyond research
The researchers' observations relate primarily to the use of LLMs for knowledge acquired during training. But language models have additional applications because they have acquired knowledge about the language itself during training.
For example, they can be used like a pocket calculator, but for text, to quickly convert existing sources into new formats, translate them, or generate meaningful segments of text based on knowledge bases (RAG). While hallucinations cannot be ruled out, they are easier to control in these scenarios.
Finally, specific knowledge of open source and some commercial language models can be improved by fine-tuning with custom data or clever prompting. A combination of measures (RAG + fine-tuning + advanced prompting) often yields the best results.