Meta's AI researchers demonstrate a system that can predict which words a person has heard from MEG and EEG data.
More than 60 million people suffer traumatic brain injuries every year. Many of them can no longer communicate with their environment afterwards.
Numerous projects are looking for a way to help those affected. One approach is to decode brain activity into speech: Instead of speaking, typing, or using gestures, a measuring device reads brain activity and translates it into speech.
There are different invasive and non-invasive methods to do this. Stereoelectroence or electroencephalography provide more accurate readings, sometimes achieving over 90 percent accuracy in attributing individual letters. However, they require neurosurgical intervention.
Non-invasive methods based on electroencephalography (EEG) or magnetoencephalography (MEG) do not require dangerous procedures but provide less accurate data.
Meta researches similarities between AI and human brain
In a new research paper, a team from Meta now presents an AI system that can identify which words subjects have heard with a relatively high degree of accuracy using MEG data.
The work is part of a larger research effort by the company: Meta wants to learn more about the human brain to develop better artificial intelligence.
The project is led by Jean-Remi King, a CNRS researcher at the Ecole Normale Supérieure and a researcher at Meta AI. King's team showed late last year that it can predict human brain responses to language based on activations of a GPT-2 language model in response to the same stories.
Video: Meta
Then, in a paper published in June, King's team compared an AI model trained with speech recordings to fMRI recordings of more than 400 people listening to audiobooks. According to the researchers, most brain areas correlated significantly with the algorithm's activations in response to the same speech input. The AI algorithm learned brain-like representations, the team concluded.
Meta decodes MEG with artificial intelligence
In the work now published, King's team builds on work done in June: The Wav2Vec model trained there with 600 hours of speech recordings extracts a representation of a three-second speech recording and learns to map those representations to corresponding representations of brain activity from MEG and EEG.
The representations of brain activities are provided by a brain module trained with nearly 150 hours of MEG and EEG recordings from 169 people.
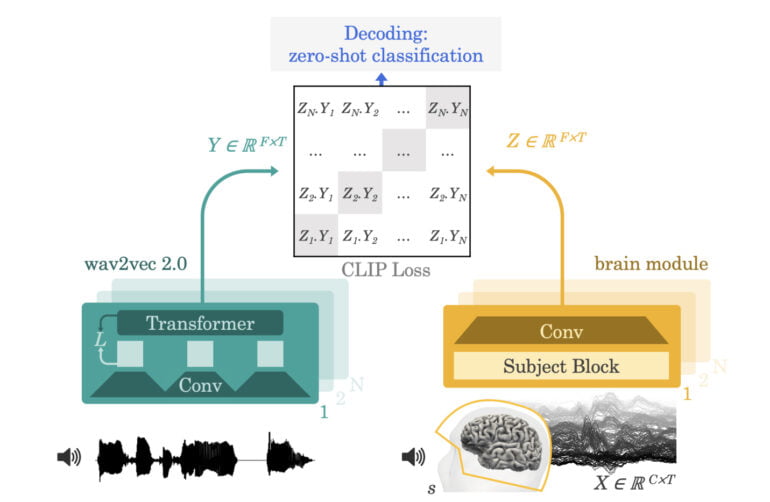
After training, the AI model can identify which clip from a large pool of audio clips the person was listening to based on a snippet of brain activity. To achieve this, the algorithm derives the words that the person was most likely to have heard.
Meta's AI system achieves a top-10 accuracy of up to 73 percent on 793 possible words for MEG recordings. So among ten suggested words, there is a 72 percent probability of the correct word. In up to 44 percent of the cases, the correct word was the first suggestion. In more than 50 percent of cases, it was among the first two suggestions.
Next step: brain to language - without intervention
Meta's research has numerous limitations. For example, the accuracy of more portable EEGs is less than 32 or even 20 percent. Also, Meta's team doesn't decode thoughts into language, but tries to identify what words a person has heard. That's not enough for solid communication.
Still, the research is an important step because it shows that AI can successfully learn to decode noisy, non-invasive recordings of brain activity during speech perception, Meta said.
Moreover, the system got better with more training data, and the accuracy achieved did not require time-consuming personalization for individuals. More data could add to this effect.
In the end, hardware requirements were not a problem either. The MEG devices used here are not portable, but wearable MEGs are currently being developed and some are already in use.
The next step, the researchers say, is now to extend the approach to decode speech directly from brain activity - without going through a pool of audio clips.