- Added current results from the LMarena.ai standard model benchmark
- Included statements from LMarena.ai and Artificial Analysis
Update April 12, 2025:
The "standard" Maverick model from Llama 4 currently ranks 32nd on LMarena, well behind the top-performing models. But the results need context: even older systems like Qwen 2.5 appear above widely used Anthropic models such as Sonnet 3.7 and 3.5. The score differences among models are often marginal.
LMarena shows how arbitrary benchmarks can be when they're not tied to clearly defined tasks—and how easily they can be manipulated, as seen with Meta’s experimental Maverick model discussed below. Ultimately, the most useful benchmark is how well a model performs on the tasks you care about, and whether it offers a good balance of cost and performance.
Update April 9, 2025:
LMArena has released evidence addressing concerns about Meta's "experimental" AI model. The platform published over 2,000 head-to-head comparisons, including user prompts, model responses, and user preferences.
"Meta's interpretation of our policy did not match what we expect from model providers," LMArena stated. "Meta should have made it clearer that 'Llama-4-Maverick-03-26-Experimental' was a customized model to optimize for human preference."
The data shows Llama 4 consistently produces longer, more formatted responses with frequent emoticon use - suggesting Meta specifically tuned the model for benchmark performance. LMArena plans to test the standard version of Llama-4-Maverick and publish those results shortly.
Benchmark scores rise after revised methodology
Artificial Analysis has revised its evaluation criteria and updated Llama 4's scores. By accepting responses formatted as "The best answer is A" for multiple-choice questions, the platform recorded significant changes in MMLU Pro and GPQA Diamond benchmarks. Scout's Intelligence Index increased from 36 to 43, while Maverick moved from 49 to 50, demonstrating how scoring methods can impact benchmark results.
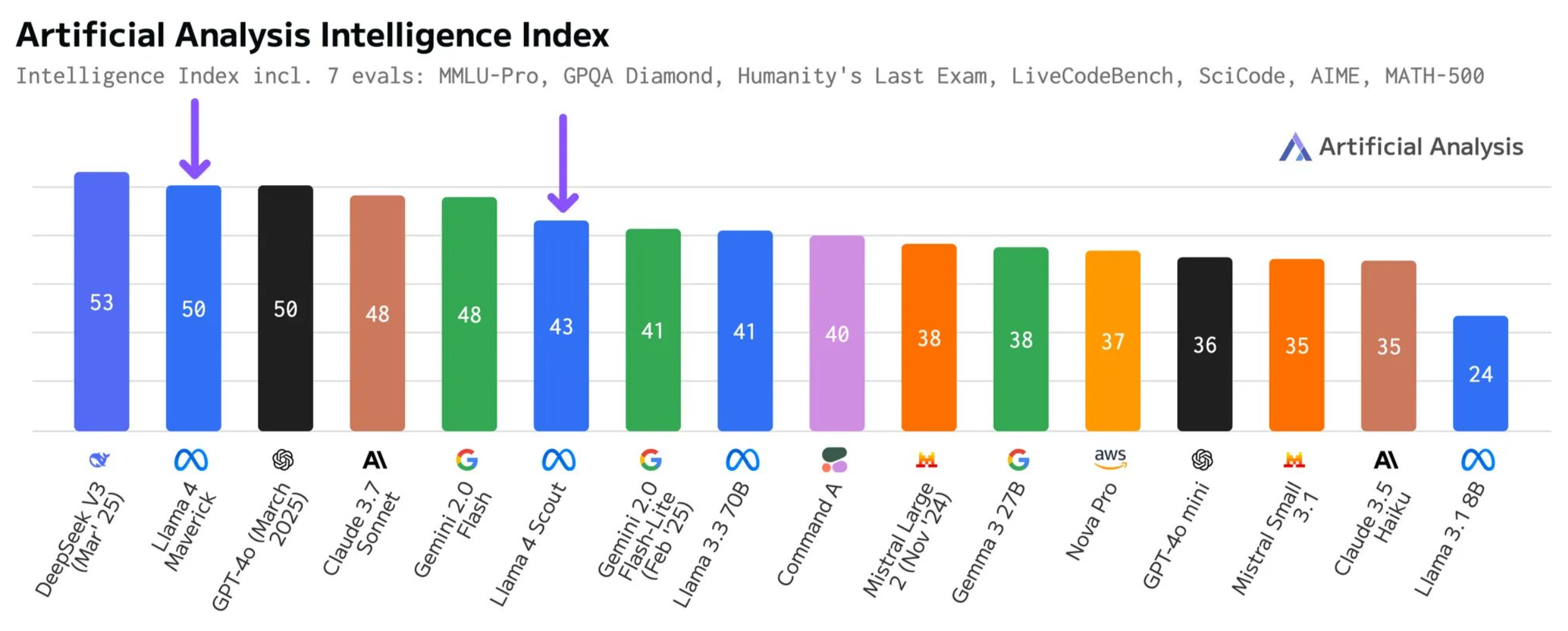
The new data highlights Maverick's efficient design - it achieves these scores with 17 billion active parameters, compared to Deepseek V3's 37 billion. In total parameters, Maverick uses 402 billion versus Deepseek V3's 671 billion, while also supporting image processing capabilities.
Original article from April 7, 2025:
New independent evaluations reveal that Meta's latest Llama 4 models - Maverick and Scout - perform well in standard tests but struggle with complex long-context tasks.
According to the aggregated "Intelligence Index" from Artificial Analysis, Meta's Llama 4 Maverick scored 49 points while Scout reached 36. This places Maverick ahead of Claude 3.7 Sonnet but behind Deepseek's V3 0324. Scout performs on par with GPT-4o-mini and outperforms both Claude 3.5 Sonnet and Mistral Small 3.1.
Both models demonstrated consistent capabilities across general reasoning, coding, and mathematical tasks, without showing significant weaknesses in any particular area.
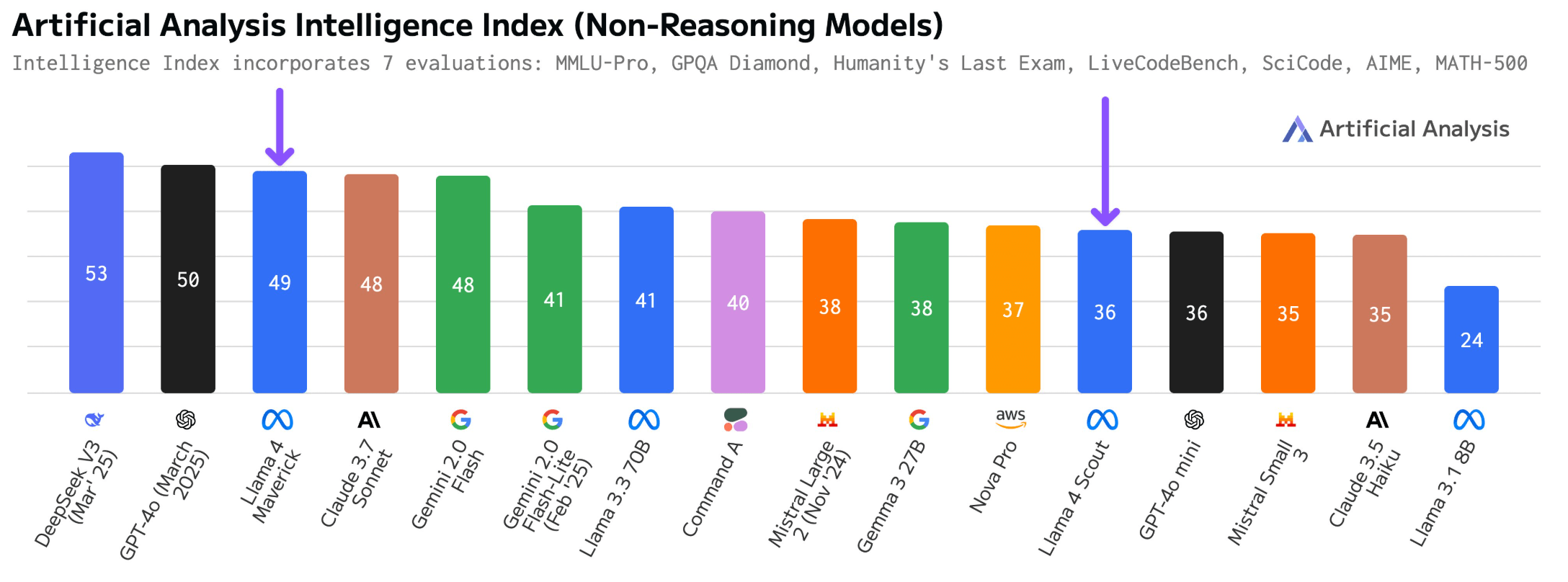
Maverick's architecture shows some efficiency, using just half the active parameters of Deepseek V3 (17 billion versus 37 billion) and about 60 percent of the total parameters (402 billion versus 671 billion). Unlike Deepseek V3, which only processes text, Maverick can also handle images.
Artificial Analysis reports median prices of $0.24/$0.77 per million input/output tokens for Maverick and $0.15/$0.4 for Scout. These rates undercut even the budget-friendly Deepseek-V3 and cost up to ten times less than OpenAI's GPT-4o.
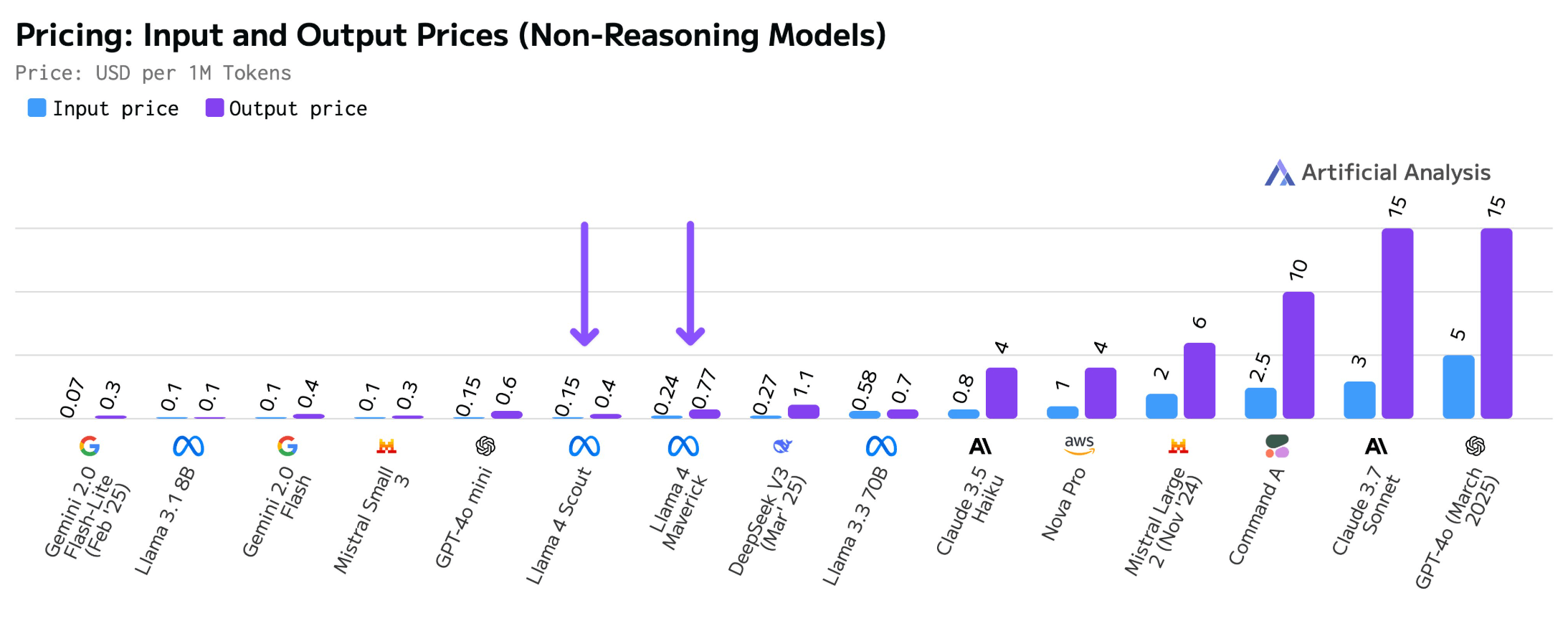
Questions arise over LMArena results
The Llama 4 launch hasn't been without controversy. Multiple testers report significant performance differences between LMArena - a benchmark Meta heavily promotes - and the model's performance on other platforms, even when using Meta's recommended system prompt.
Meta acknowledged using an "experimental chat version" of Maverick for this benchmark, suggesting possible optimization for human evaluators through detailed, well-structured responses with clear formatting.
In fact, when LMArena's "Style Control" is activated - a method that separates content quality from presentation style - Llama 4 drops from second to fifth place. This system attempts to isolate content quality by accounting for factors like response length and formatting. It's worth noting that other AI model developers likely employ similar benchmark optimization strategies.
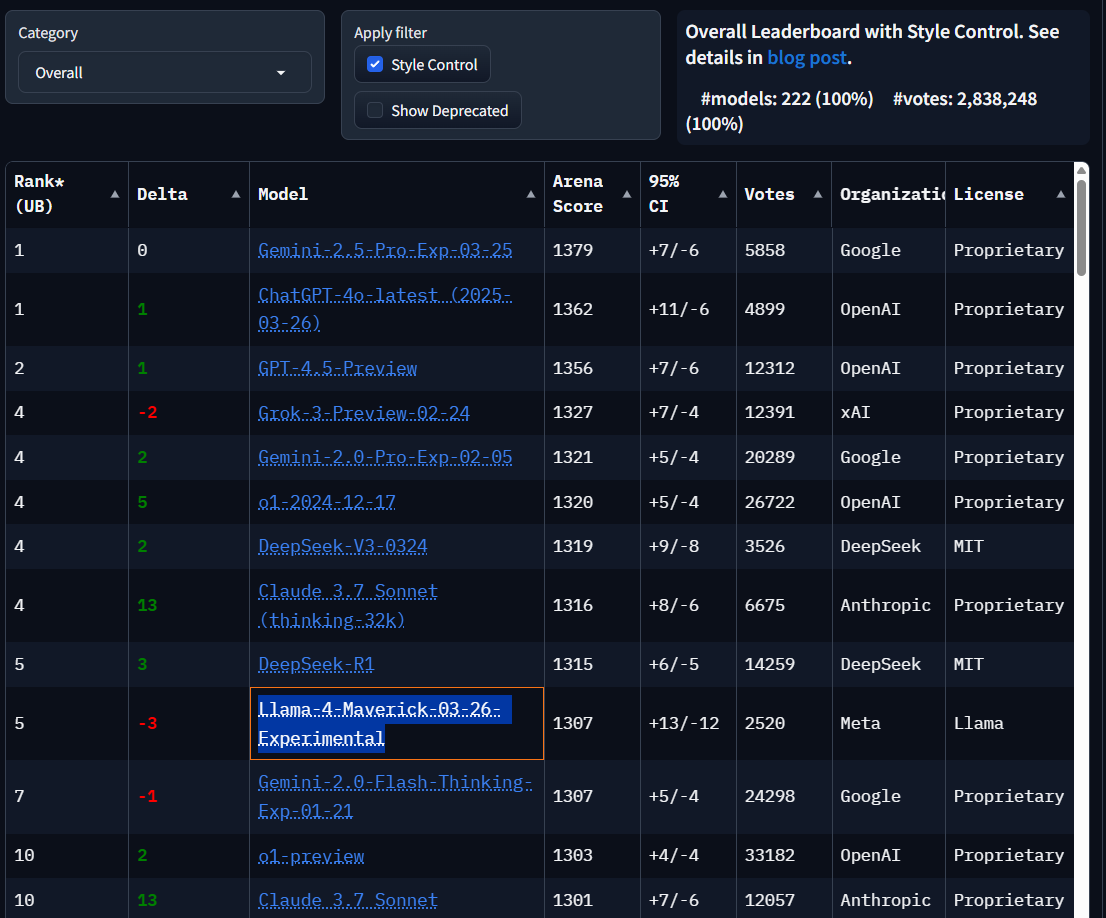
Long-context performance falls short
The most significant issues emerged in tests by Fiction.live, which evaluate complex long-text comprehension through multi-layered narratives.
Fiction.live argues their tests better reflect real-world use cases by measuring actual understanding rather than just search capabilities. Models must track temporal changes, make logical predictions based on established information, and distinguish between reader knowledge and character knowledge.
Llama 4's performance disappointed in these challenging tests. Maverick showed no improvement over Llama 3.3 70B, while Scout performed "downright atrocious."
The contrast is stark: while Gemini 2.5 Pro maintains 90.6 percent accuracy with 120,000 tokens, Maverick achieves only 28.1 percent and Scout drops to 15.6 percent.
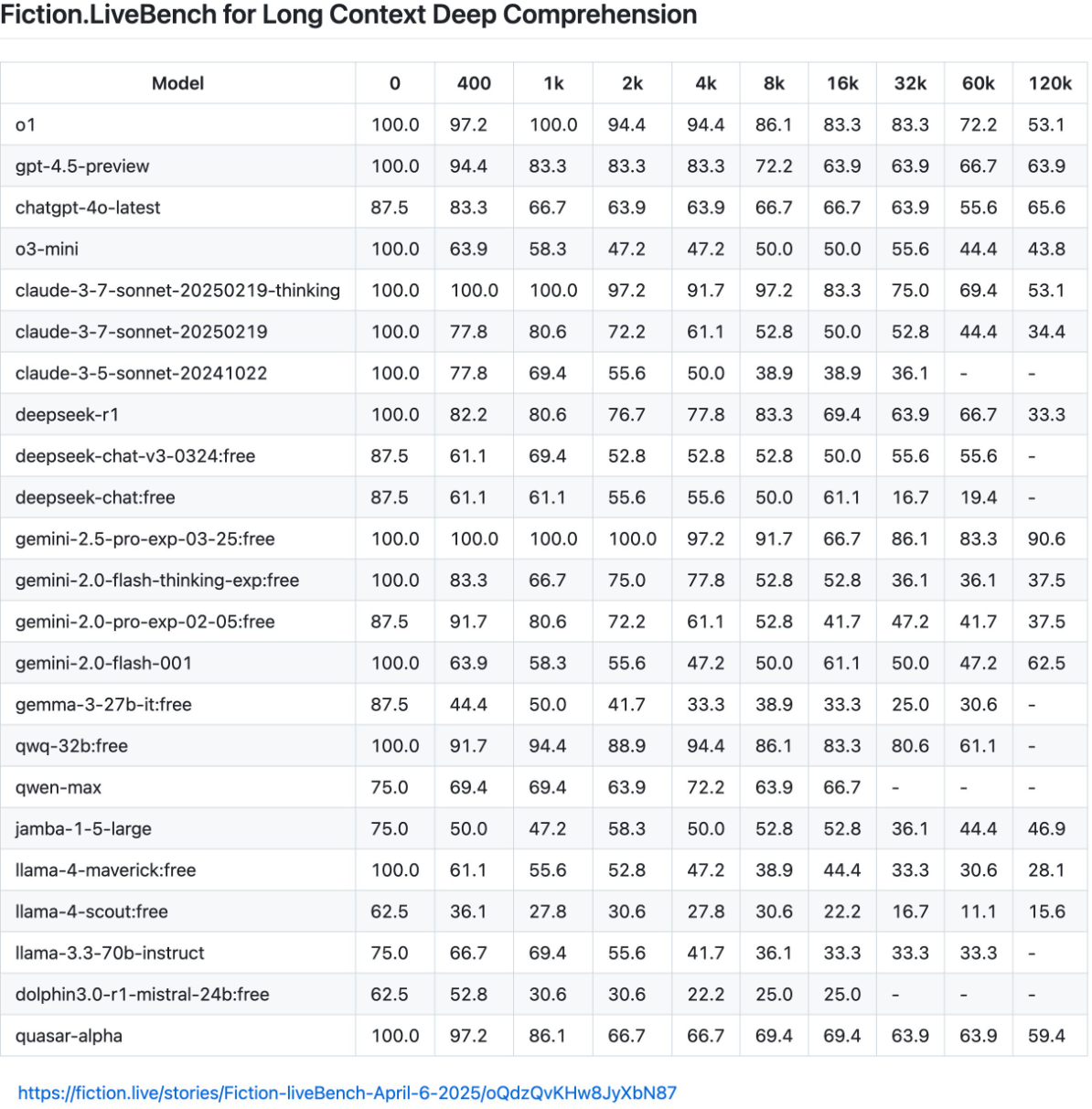
These results challenge Meta's claims about long-context capabilities. Scout, advertised to handle 10 million tokens, struggles with just 128,000. Maverick also fails to consistently process documents within 128,000 tokens but claims a one-million token context window.
Research increasingly shows that large context windows offer fewer benefits than expected, as models struggle to evaluate all available information equally. Working with smaller contexts up to 128K often proves more effective, and users typically achieve even better results by breaking larger documents into chapters rather than processing them all at once.
Meta responds to mixed reception
In response to mixed performance reports, Meta's head of generative AI Ahmad Al-Dahle explains that early inconsistencies reflect temporary implementation challenges rather than limitations of the models themselves.
"Since we dropped the models as soon as they were ready, we expect it'll take several days for all the public implementations to get dialed in," Al-Dahle writes. He strongly denies allegations about test set training, stating "that's simply not true and we would never do that."
"Our best understanding is that the variable quality people are seeing is due to needing to stabilize implementations," Al-Dahle says, emphasizing that various services are still optimizing their Llama 4 deployments.