OpenAI's DALL-E 2 relies on a whole set of security measures to prevent possible misuse. Now OpenAI gives a deep insight into the training process.
In April, OpenAI first shared insights into DALL-E 2, the company's new image-generating AI model. Since then, a closed beta test has been underway with impressive results. They raise questions about DALL-E 2's role in the future of creative work or make photographers fear the death of photography.
A central goal of the closed beta phase is to prepare DALL-E 2 for use as a freely available product. To this end, OpenAI wants to ensure that DALL-E 2 does not generate any violent and sexual images in particular. So far, DALL-E 2 has pretty much followed the rules.
The company has taken a number of measures to achieve this, such as input and upload filters for the system's input screen, limits on the number of images that can be generated at any one time, a comprehensive content policy, and active control of generated content, including human reviews of questionable content.
OpenAI filters training data automatically
Beyond these measures, OpenAI focuses on mitigating potentially dangerous content in the training dataset. For the training of DALL-E 2, OpenAI collected hundreds of millions of images and their captions from the Internet. In the process, numerous images with unwanted content were found in the automatically collected dataset.
To identify and remove this content, OpenAI uses a semi-automatic process: using a few hundred images that have been manually classified as problematic, a neural network is trained to classify images.
Another algorithm then uses this classifier to find some images in the main dataset that could improve the classifier's performance. These images are then processed by humans and, if suitable, used to further train the classifier. This process is performed for several specialized classifiers.
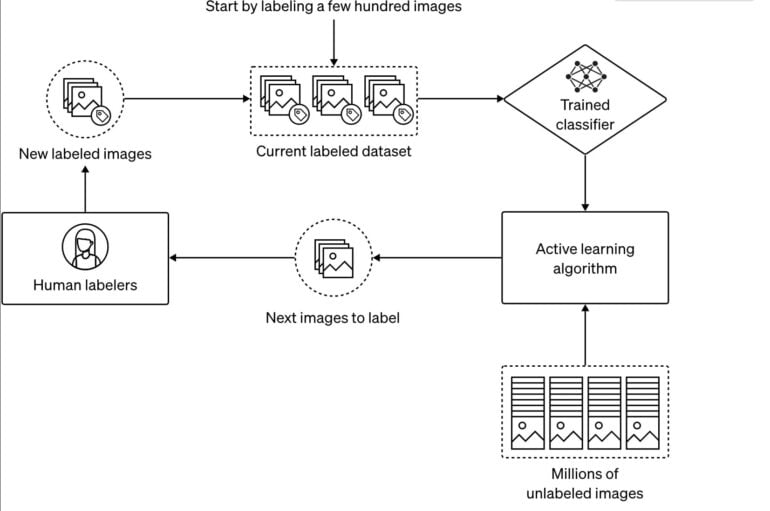
The trained classifier can then automatically filter problematic images from the hundreds of millions of images. In the process, filtering out problematic data takes precedence over preserving unproblematic data, OpenAI writes. It is significantly easier to refine a model later with more data than to make the model forget something it has already learned, according to the company.
The filtering process, which is so cautious, eliminated about five percent of the total training data set, including many images that did not show problematic content, the company said. Better classifiers in the future could recover some of this lost data and further improve DALL-E 2's performance.
To test the efficiency of its approach, OpenAI trained two GLIDE models, one filtered and one unfiltered. GLIDE is a direct predecessor of DALL-E 2, and as expected, the filtered model generated significantly less graphic and explicit content.
Data filter increases bias in AI model
However, the successful filtering process has an unexpected side effect: it creates or increases the model's bias toward certain demographic groups. This bias is a major challenge even as it is, but the filtering process, which is actually positive, exacerbates the problem, OpenAI said.
The company cites the input "a CEO" as an example: The unfiltered model tends to produce more images of men than women - much of this bias is due to the training data.
However, this effect was exacerbated in the filtered model. It showed images of men almost exclusively. Compared to the unfiltered model, the frequency of the word "woman" in the data set was reduced by 14 percent, while that of the word "man" was only six percent.
There are probably two reasons for this: Although men and women are roughly equally represented in the original dataset, women may appear more frequently in sexualized contexts. Therefore, the classifiers remove more images of women, reinforcing the imbalance.
In addition, the classifiers themselves might be biased by certain class definitions or implementations and remove more images of women.
OpenAI fixes bias with reweighting of training data
However, the OpenAI team was able to significantly reduce this effect by reweighting the remaining training data for the model, such as making the less common images of women more influential in training the model. For the words tested, such as "woman" and "man," the frequency values had dropped to about one and minus one percent instead of the 14 and six percent.
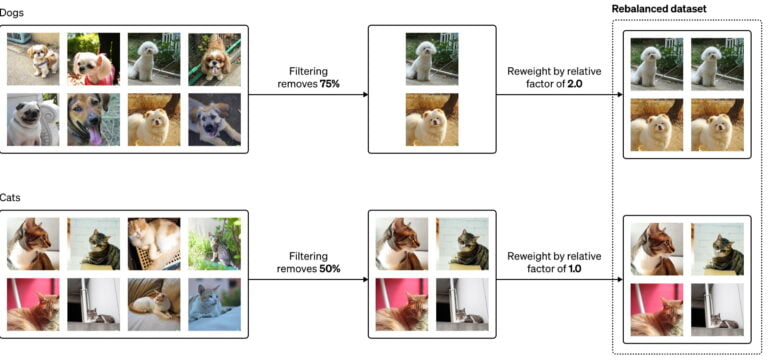
In a blog post, OpenAI also shows that models like GLIDE and DALL-E 2 sometimes learn by memorization, reproducing training images instead of creating new ones. The company identified the cause as images that are frequently repeated in the training dataset. The problem can be removed by removing visually similar images.
Next, OpenAI wants to further improve the filters for training, further combat the bias in DALL-E 2, and better understand the observed effect of memorization.