A study by OpenAI, OpenResearch, and the University of Pennsylvania examines the potential impact of large language models on the labor market.
According to the study, large language models will impact at least 10 percent of the work of about 80 percent of U.S. workers. For 19 percent of workers, language models are expected to influence at least 50 percent of tasks.
The researchers based their findings on an analysis of 19,265 tasks and 2,087 work processes described in the O*NET 27.2 database. The database includes 1,016 occupations with detailed descriptions of their tasks and work processes, called detailed work activities (DWAs), such as "study scripts to determine project requirements."
As a proxy for the potential economic impact on jobs, the researchers defined the exposure to the language models in relation to the amount of time it takes to complete a task.
If a GPT model or access to a GPT-based system can reduce that time by 50 percent, the researchers consider that task to be directly exposed to AI language models. They do not distinguish between effects that augment work and effects that replace work.
The researchers justify the "somewhat arbitrary" choice of the 50 percent threshold by pointing out that acceptance of the technology is likely to be highest in areas with significant productivity gains. In addition, they say, the threshold makes "interpretation by annotators" easier.
To apply the exposure definition to labor force data in the U.S. economy, the team used human raters as well as GPT-4 itself. Both the capabilities of current models and those of anticipated GPT-based software were considered.
Higher-paying jobs more affected by language models
One finding of the study is that, overall, higher-paying jobs are more affected by AI changes. Jobs that rely heavily on science and critical thinking are less at risk. In contrast, programming and writing jobs are particularly impacted by the adoption of large language models.
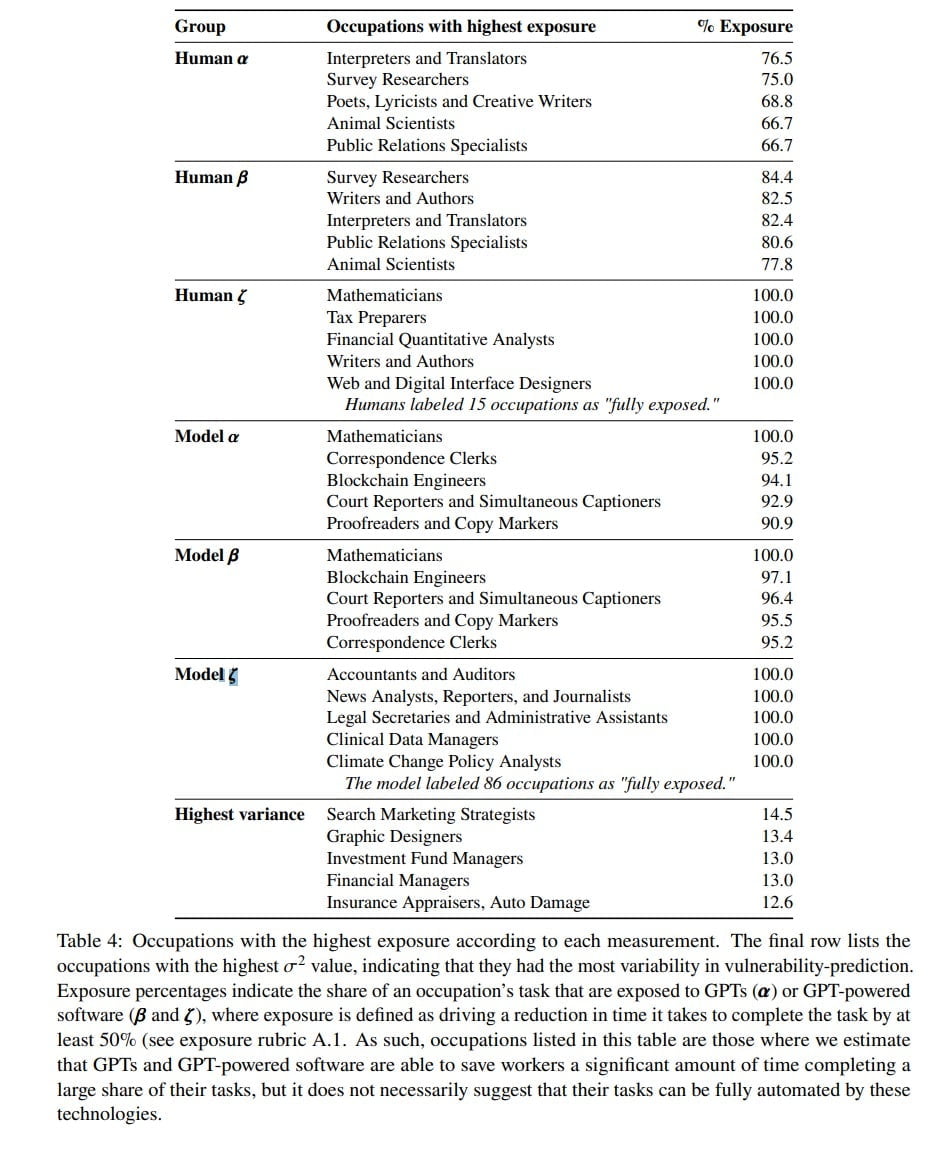
Another observation of the study is that occupations with high barriers to entry are more likely to be affected by large language models than occupations with low barriers to entry. This is also evident when looking at occupations without any exposure to large language models: Here we find mainly manual occupations such as stonemason or cook. These occupations can usually be learned after a shorter period of schooling and do not require many years of study up front.
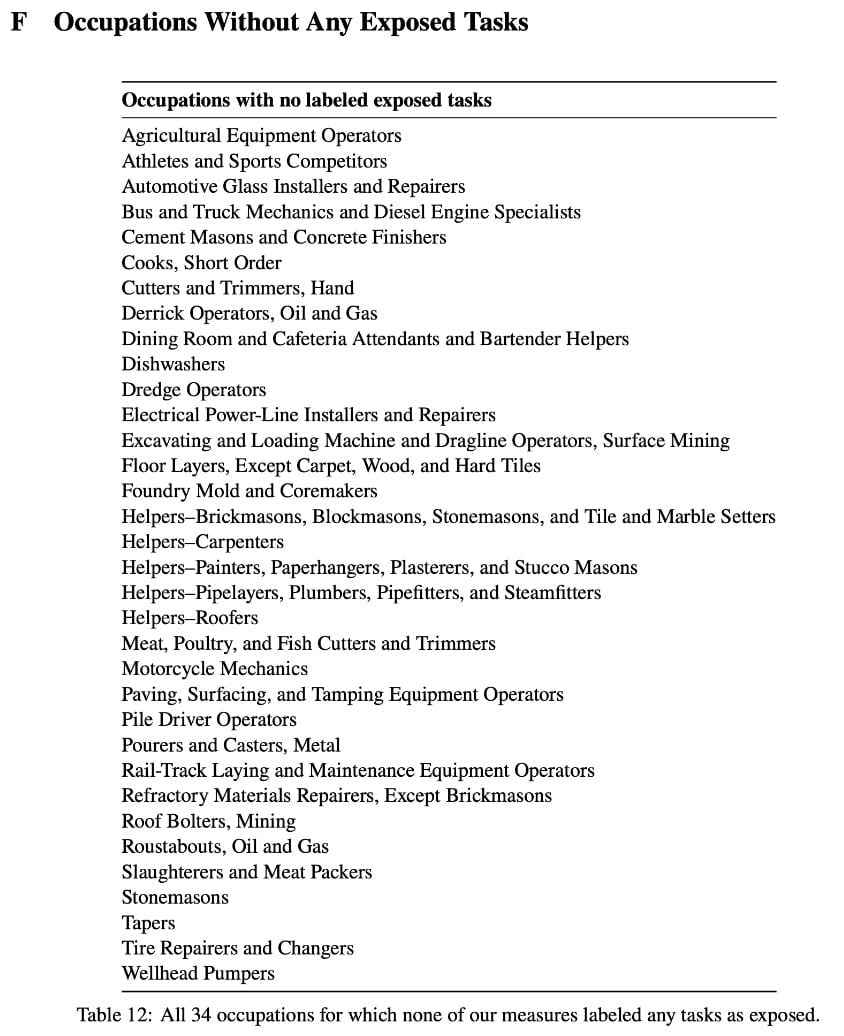
But it is reasonable to expect at least indirect effects on these occupations: If large language models make many office jobs obsolete, craft occupations could become more crowded, which in turn could increase competitive and performance pressures in these segments.
"GPTs are GPTs"
The researchers conclude their study with a comparison: GPTs are GPTs. By this they mean that the "Generative Pre-trained Transformers", the neural networks of GPT-4 and co, happen to have the same acronym as "General-Purpose Technologies" such as electricity, fire or mobility, which have a major impact on the entire economy. They hypothesize that the language GPTs studied have characteristics of these general-purpose technologies.
While LLMs have consistently improved in capabilities over time, their growing economic effect is expected to persist and increase even if we halt the development of new capabilities today. We also find that the potential impact of LLMs expands significantly when we take into account the development of complementary technologies.
Eloundou et al.
Further research is needed to examine the broader implications of lifelong learning, including the potential to augment or replace human labor, the impact on quality of work, inequalities, skills development, and many other areas, the research team said. This work could help policymakers and stakeholders make more informed decisions about the "role of AI in the future of work."