The new AI benchmark ARC-AGI-2 significantly raises the bar for AI tests. While humans can easily solve the tasks, even highly developed AI systems such as OpenAI o3 clearly fail.
François Chollet and his team have released ARC-AGI-2, a new version of their AI benchmark. While following the same format as ARC-AGI-1, the new test provides what the team says is a stronger signal for measuring genuine system intelligence.
"It's an AI benchmark designed to measure general fluid intelligence, not memorized skills – a set of never-seen-before tasks that humans find easy, but current AI struggles with," Chollet explained on X. The benchmark focuses on capabilities current AI systems still lack: symbol interpretation, multi-step compositional thinking, and context-dependent rule application.
The benchmark has been fully calibrated against human performance. In live testing sessions with 400 participants, only tasks that multiple people could reliably solve were kept. Average test takers achieve 60 percent without prior training, while a panel of 10 experts reached 100 percent.
Today, we're releasing ARC-AGI-2. It's an AI benchmark designed to measure general fluid intelligence, not memorized skills - a set of never-seen-before tasks that humans find easy, but current AI struggles with.
It keeps the same format as ARC-AGI-1, while significantly... pic.twitter.com/9mDyu48znp
- François Chollet (@fchollet) March 24, 2025
Current AI models struggle with new benchmark
Initial testing results paint a sobering picture. Even the most advanced systems perform poorly. Pure language models like GPT-4.5, Claude 3.7 Sonnet, and Gemini 2 score zero percent. Models with basic chain-of-thought reasoning like Claude 3.7 Sonnet Thinking, R1, and o3-mini manage only zero to one percent.
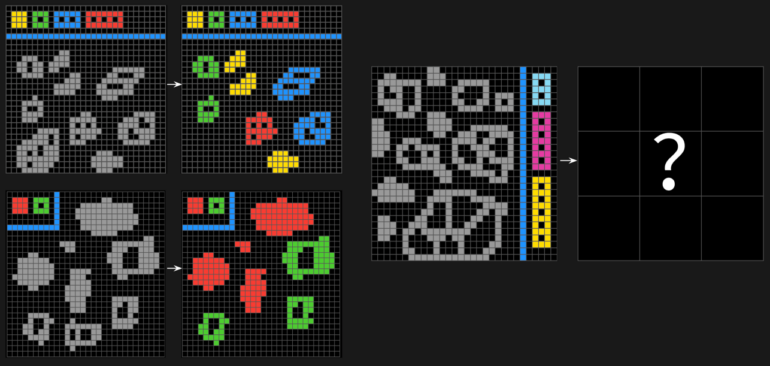
OpenAI's o3-low model showed a particularly notable performance drop, falling from 75.7 percent on ARC-AGI-1 to roughly 4 percent on ARC-AGI-2. The ARC Prize 2024 winners, Team ARChitects, experienced a similar decline from 53.5 percent to 3 percent.
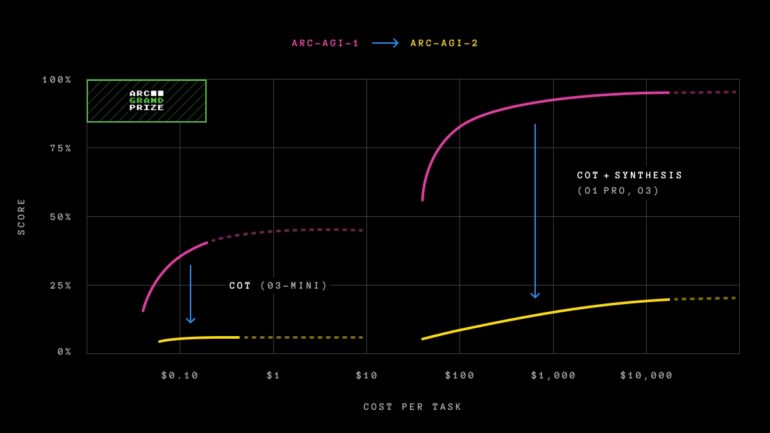
Some models, particularly o3-high, still lack extensive testing or rely on projections, meaning their actual performance could be higher.
Efficiency becomes crucial metric
ARC-AGI-2 introduces a new efficiency metric. The benchmark now evaluates not just problem-solving ability but also how efficiently that ability is deployed. Cost serves as the initial metric, enabling direct comparisons between human and AI performance.
"We know that brute-force search could eventually solve ARC-AGI (given unlimited resources and time to search). This would not represent true intelligence," the ARC Prize Foundation explains. "Intelligence is about finding the solution efficiently, not exhaustively."
The efficiency gap between human and artificial intelligence is stark. While a human expert panel solves 100 percent of tasks at around $17 per task, OpenAI's o3-low model spends approximately $200 per task to achieve just four percent accuracy.
ARC Prize 2025 launches with million-dollar prize pool
The ARC Prize 2025 competition launches alongside ARC-AGI-2, offering $1 million in total prizes. The main prize of $700,000 requires achieving 85 percent accuracy on the private evaluation set. Additional rewards include $125,000 in guaranteed progress prizes and $175,000 in yet-to-be-announced prizes.
The competition runs on Kaggle from March through November 2025. Unlike the public leaderboard on arcprize.org, Kaggle's rules limit participants to about $50 in computing power per submission and prohibit using internet APIs.
While the original ARC-AGI-1 benchmark from 2019 was considered one of the toughest tests of AI and signaled the rise of reasoning models, neither version claims to indicate the achievement of AGI. According to the development team, both benchmarks can be solved without reaching artificial general intelligence.