A team of researchers from various Chinese institutions has created Infinity-MM, one of the largest publicly available datasets for multimodal AI models, and trained a new top-performing model on it.
The Infinity-MM dataset consists of four main categories: 10 million image descriptions, 24.4 million general visual instruction data, 6 million selected high-quality instruction data, and 3 million data generated by GPT-4 and other AI models.
The team used existing open source AI models to create the data: The RAM++ model first analyses the images and extracts important information. This is then used to generate questions and answers. A special classification system with six main categories is used to ensure the quality and diversity of the data generated.
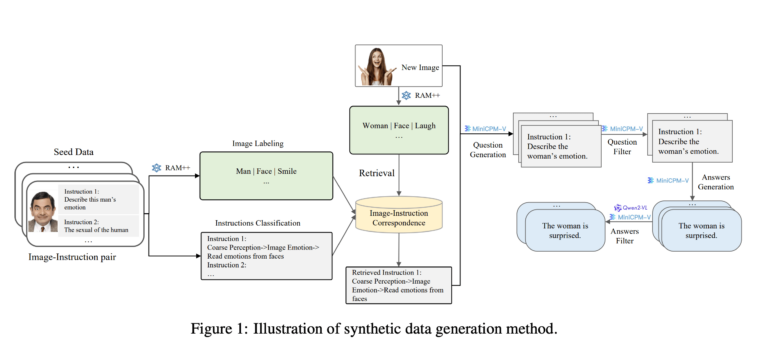
Four-stage training for better performance
The trained model, Aquila-VL-2B, is based on the LLaVA-OneVision architecture and uses Qwen-2.5 as a language model and SigLIP for image processing. Training was performed in four successive phases of increasing complexity.
In the first phase, the model learned basic image-text associations. Subsequent phases included general visual tasks, specific instructions and finally the integration of synthetically generated data. The maximum image resolution was also gradually increased.
New standards in benchmark tests
In extensive testing, Aquila-VL-2B achieved top scores despite its comparatively small size of only two billion parameters. In the MMStar benchmark for multimodal understanding, it scored 54.9% - the best for a model of this size.
According to the researchers, performance on mathematical tasks is particularly impressive, scoring 59% on the MathVista test, significantly outperforming comparable systems. The model also performed very well in general image understanding tests such as HallusionBench (43%) and MMBench (75.2%).
The researchers were also able to demonstrate that the integration of synthetically generated data significantly improved performance. Tests without this additional data resulted in an average performance drop of 2.4%.
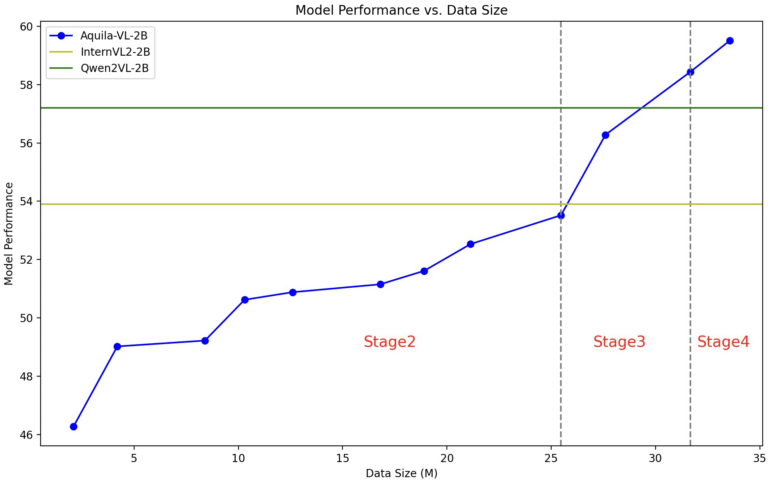
The team is making both the dataset and the model available to the research community. The model was trained on Nvidia A100 GPUs as well as Chinese chips.
Vision Language Models on the rise
The development of Aquila-VL-2B fits into a broader trend in AI research. While closed commercial systems such as GPT-4o have often shown better performance, open-source models are catching up. The use of synthetic training data is proving particularly promising.
For example, the open-source model Llava-1.5-7B was able to outperform even GPT-4V on certain tasks by training on over 62,000 synthetically generated examples. Meta also relies heavily on synthetic data with Llama models.
However, current tests also reveal the limitations of today's VLMs. Image processing is still inadequate in many areas, especially when it comes to extracting specific visual information from large amounts of data. The limited resolution of visual encoders is also a technical limitation.