Startup Extropic has published its first "litepaper" presenting an approach to overcome the limitations of conventional computer chips.
Startup Extropic provides a first look at the company's planned AI hardware. The Austin, Texas-based startup was founded in 2022 by former Google researchers Guillaume Verdon and Trevor McCourt. Both have experience in research and development; McCourt, for example, was part of the Quantum AI team at Google. The new hardware platform is designed to use thermal fluctuations in materials as a computing resource for generative AI. According to the company, this new approach makes it possible to develop AI accelerators that are orders of magnitude faster and more energy efficient than digital processors such as CPUs, GPUs, or TPUs.
"The demand for computing power in the AI era is increasing at an unprecedented exponential rate," the Extropic founders wrote in a blog post. However, the previous exponential growth in computing efficiency is reaching its physical limits: Transistors are approaching the size of atoms, where effects like thermal noise make reliable digital operation impossible.
As a result, the power requirements of modern AI systems are exploding. Large technology companies are already considering extreme measures, such as building nuclear power plants to power data centers for training large AI models. Extropic aims to solve this problem through a paradigm shift in computing architecture.
Randomness as a resource for probabilistic calculations
Biological systems serve as a model, according to Extropic, because they compute much more efficiently than anything mankind has ever built. In living cells, chemical reaction networks drive information processing. Because the number of reaction partners can be counted, the interactions are inherently discrete and random, the team said. The fewer molecules there are, the more these random fluctuations dominate the dynamics of the system.
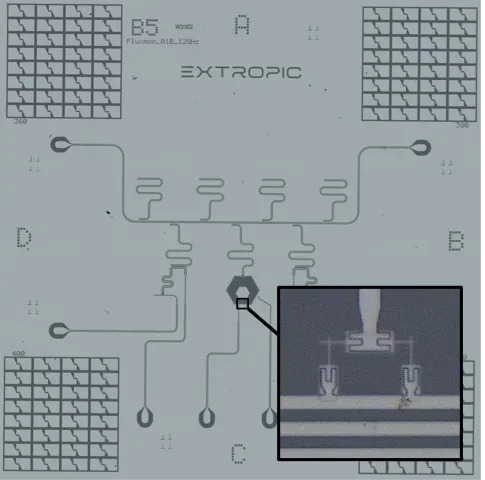
Extropic engineers want to harness this inherent randomness as a resource for probabilistic computation. To do this, they are developing a new generation of AI chips based on energy-based models (EBMs). These novel chips work with so-called "extropic accelerators," superconducting processors that operate at low temperatures and are nanofabricated from aluminum. The fundamental innovation is that the chips use the inherent thermal noise of the environment to generate random data, making them ideal for training and inferencing energy-based models (EBMs).
Energy-based models as the future of AI training
EBMs are a concept found in both thermodynamic physics and basic probabilistic machine learning. They form the basis of AI models that predict probabilities for different scenarios based on how much "energy" a particular scenario requires. The less energy, the more likely the scenario. EBMs are not limited to simple patterns or relationships. They can cover a wide range of possibilities, making them extremely versatile.
EBMs also have the advantage of requiring only a small amount of data to unambiguously determine the probability distribution, making them ideal for modeling borderline cases.
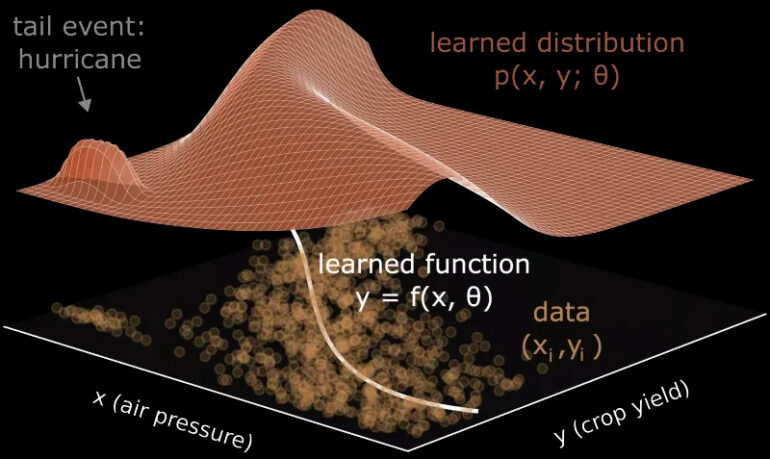
According to Extropic, EBMs accomplish this by filling in the gaps in the data with noise and trying to maximize its entropy while satisfying the statistics of the target distribution. This process, which hallucinates any possibility not present in a data set and heavily penalizes such occurrences, requires a high degree of randomness in both training and inference.
The major problem with energy-based models in the past has been the high computational cost of drawing a large number of random samples. This is very inefficient for digital computers. Extropic aims to solve this problem by implementing EBMs directly as parameterized stochastic analog circuits. This should improve runtime and power consumption by many orders of magnitude compared to digital hardware.
Another goal of Extropic is to make its technology widely available. They are therefore working on semiconductor devices that operate at room temperature and can be integrated into existing hardware setups such as GPU expansion cards. Extropic is also working on a software layer that translates the abstract specifications of EBMs into the appropriate hardware control language.