A microphone membrane as a computing unit? In the search for efficient AI hardware, researchers are turning to physical neural networks integrated into real objects.
The success of artificial intelligence over the past decade has been driven by the widespread adoption of artificial neural networks. This has been made possible by the realization that the backpropagation algorithm can be efficiently used on graphics cards to train neural networks for image analysis, for example. Since the ImageNet moment in 2012, ever larger networks have been trained on increasingly powerful hardware.
The energy hunger of these massive networks during training and use has led to the development of specialized AI accelerators, such as Google's TPUv3 or Cerebra's WSE2. Most of these chip alternatives primarily target more efficient usage, as this accounts for up to 90 percent of the energy cost of an AI network in commercial applications. The majority of these dedicated AI processors are still based on silicon chips, although in some cases optical chips are also used.
Hello, Echo: Canyons are passive processors
In the search for even more efficient alternatives, researchers led by physicist Logan Wright are turning to so-called physical neural networks (PNNs). “Everything can be a computer,” says Wright, who was a longtime researcher at Cornell University and is now at NTT Research. “We’re just finding a way to make the hardware physics do what we want.”
With that comes a new idea of what it means for something to "compute." Physical objects could compute passively, Wright and his team say. Canyons, for example, add an echo to voices without the need for a soundboard.
This processing of a signal by a static physical object that does not require any externally supplied energy to do so, nor does it need to execute an algorithm in the classical sense, is a model for Wright's team.
The goal is neural networks that are integrated into an object in physical form rather than running on silicon chips as is traditionally the case.
"Physics-aware training" for physical neural networks
In their new work, the researchers realized neural networks in three different physical systems: one mechanical, one optical and one analog-electronic. The mechanical system consists of a metal plate that is vibrated by a loudspeaker. The output signal is recorded by a microphone.
The optical system passes laser light through various crystals, and in the analog-electronic system, current flows through small circuits.
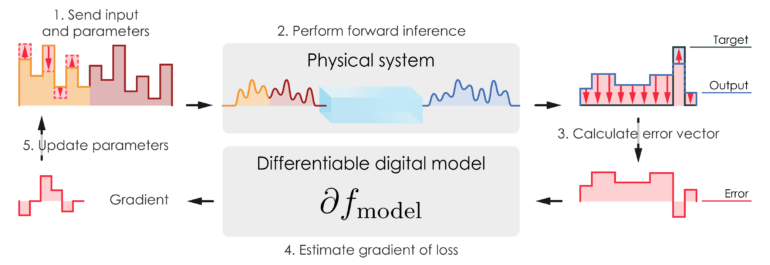
The researchers coded the input data for each network into sound, light, or voltage. Each system receives additional encoded parameters to help the physical network process the input data correctly.
These parameters are adjusted during training via the backpropagation algorithm until the algorithm finds the right combination of parameters and input signal. This approach is called physics-oriented training (PAT).
Physical neural networks achieve high accuracy with PAT
In tests, the physical networks then had to recognize handwritten numbers or one of seven tones, for example. The networks achieved an average accuracy of between 87 and 97 percent.
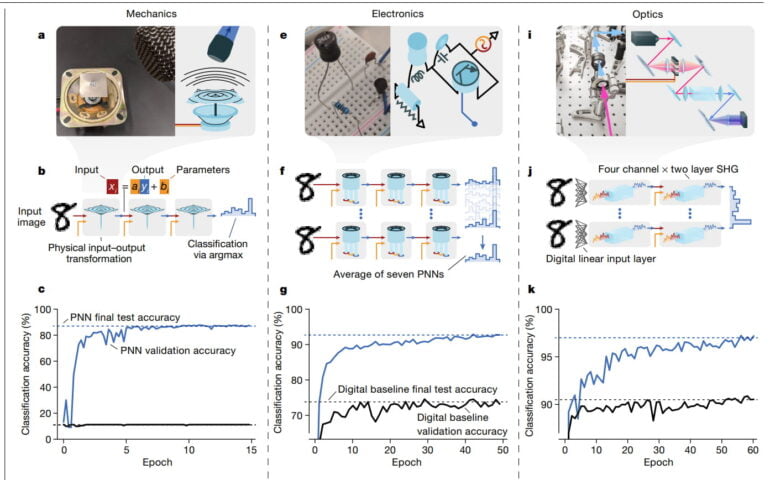
According to the researchers, the results show that controllable physical systems can be trained to perform AI calculations and are potentially several orders of magnitude faster and more energy efficient than silicon solutions.
Wright and his team next plan to develop systems in which they adjust physical objects during training, rather than relying on additional parameters to supplement input.
The physicist sees great potential for physical networks as smart sensors: the optics of a microscope, for example, could one day detect cancer cells even before the light is digitally processed. Or the microphone membrane of a smartphone could respond to a wake-up word.
In that case, people will probably think less about these being AI calculations, Wright said. Physical neural networks would then simply be "functional machines."