Vision-Robotics Bridge learns the affordances of environments to accelerate robot learning.
Several research projects are investigating how robots can learn from videos because there is not enough robot training data - one reason why OpenAI, for example, has stopped its own robot research.
Comprehensive robot training data would require many robots performing actions in the real world, but they would need to be trained on them beforehand - a chicken-and-egg problem. Video training is seen as a possible solution, as AI models could learn how humans interact with their environment from video data and then transfer these skills to robots.
Vision-Robotics Bridge develops an affordance model for robots
he term affordance, coined by the American psychologist James J. Gibson, refers to the fact that living things do not see objects and features in their environment in terms of their qualities, but rather primarily as an offer to the individual. For example, living beings do not perceive a river as simply flowing water but as an opportunity to drink.
The team from Carnegie Mellon University and Meta AI is guided by this concept and defines affordance in the context of robotics as the sum of contact point and post-contact trajectories. The AI model learns from video to identify objects with possible actions, as well as possible movement patterns after grasping an object.
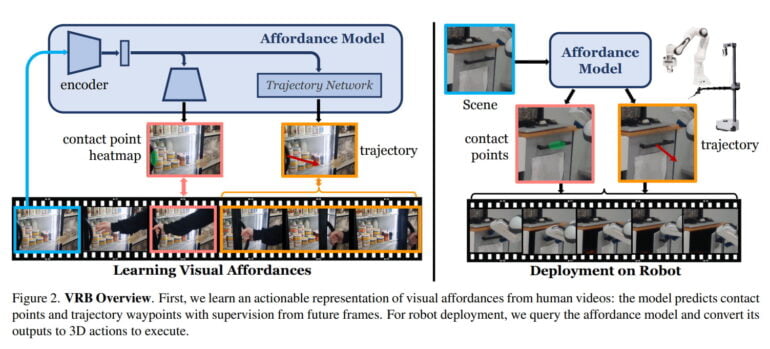
For example, it learns that a fridge is opened by pulling the handle and in which direction a person pulls it. In the case of a drawer, it recognizes the handle and learns the only correct direction of movement to open the drawer.
Video: CMU / Meta
Video: CMU / Meta
VRB proves itself in 200 hours of real-world testing
In robotics, VRB aims to provide a robot with contextualized perception to help it learn its tasks faster. The team shows that VRB is compatible with four different learning paradigms, and applies VRB in four real-world environments on more than ten different tasks using two different robot platforms.
In extensive experiments lasting more than 200 hours, the team demonstrated that VRB is far superior to previous approaches. In the future, the researchers plan to apply their method to more complex, multistep tasks, incorporate physical concepts such as force and tactile information, and investigate the visual representations learned from VRB.
More information is available on the VRB project page. The code and dataset should also be available there soon.