The leap from 2D to 3D poses challenges for existing diffusion methods. RenderDiffusion seems promising because it can render a 3D scene based on a single 2D image.
For 2D images, diffusion methods have made great progress over the past few months. Gradually, researchers on this path are also seeing success for 3D objects. Google, for example, recently demonstrated 3DiM, which can generate 3D views from 2D images.
Diffusion models currently achieve the best performance in both conditional and unconditional image generation, according to researchers at several UK universities and Adobe Research. So far, however, these models have not supported consistent 3D generation or reconstruction of objects from a single perspective.
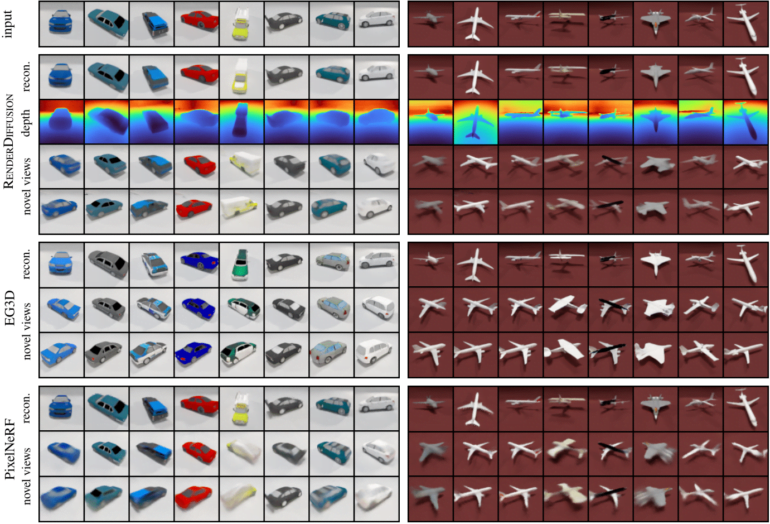
3D denoising
In their paper, the researchers present RenderDiffusion. This, they say, is the first diffusion model for 3D generation and inference that can be trained using only monocular 2D supervision. The model can generate a 3D scene from a single 2D image end-to-end without relying on multiview data such as Gaudi.
At the heart of the method is a customized architecture for denoising the original image. At each step, the method generates a three-dimensional, volumetric 3D representation of a scene. The resulting 3D representation can be rendered from any viewpoint. This diffusion-based approach also allows the use of 2D inpainting to modify the generated 3D scenes.
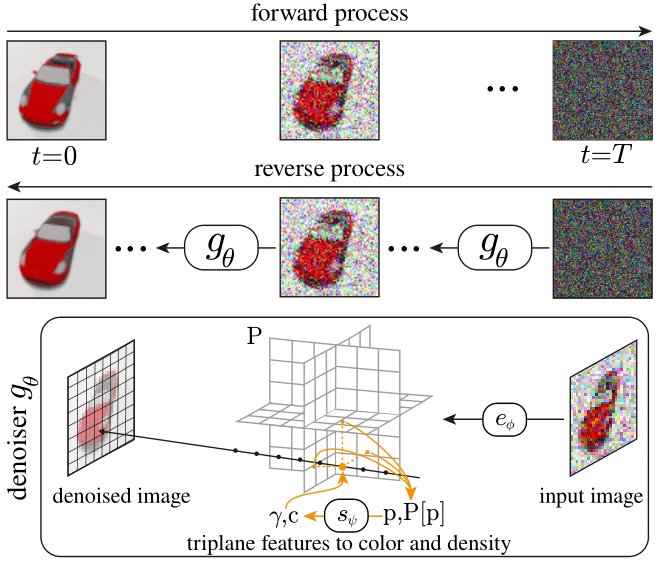
Compared to similar generative 3D models such as the GAN-based EG3D and PixelNeRF, which uses multiview images of 2D input images, RenderDiffusion produces more faithful 3D objects of the input image that are also sharper and more detailed, the researchers write.
A major disadvantage of RenderDiffusion is that training images must be labeled with camera parameters. In addition, the generation across different object categories is difficult.
These limitations could be overcome by estimating camera parameters and object bounding boxes, and by using an object-centric coordinate system. In this way, the system could also generate scenes with multiple objects, the researchers write.
RenderDiffussion could enable "full 3D generation at scale"
The researchers see their paper as a significant contribution to the 3D industry: "We believe that our work promises to enable full 3D generation at scale when trained on massive image collections, thus circumventing the need to have large-scale 3D model collections for supervision."
Future work could enable object and material editing to enable an "expressive 3D-aware 2D image editing workflow."
The researchers plan to publish their code and datasets on Github soon.