Googles new diffusion model generates 3D views from a single image. The authors see their work as an alternative to NeRFs.
Within the domain of generative AI systems, diffusion models have become pretty popular: AI systems such as DALL-E 2, Imagen, Midjourney or Stable Diffusion rely on the method to generate images. The video models Imagen Video, Make-a-Video and Phenaki generate videos, Motion Diffusion animations, and CLIP-Mesh 3D models with diffusion.
Now Google researchers are demonstrating "3D Diffusion Models" (3DiM), a diffusion model that generates new 3D views from a single image.
Google's 3DiM generates 3D view with one image
Google's 3DiM processes a single reference image with relative pose information for the 3D view and generates a new view via diffusion. Unlike similar AI systems, 3DiM uses these new images to generate subsequent views, rather than relying on only one single view for each newly generated view. Google researchers refer to this as stochastic conditioning.
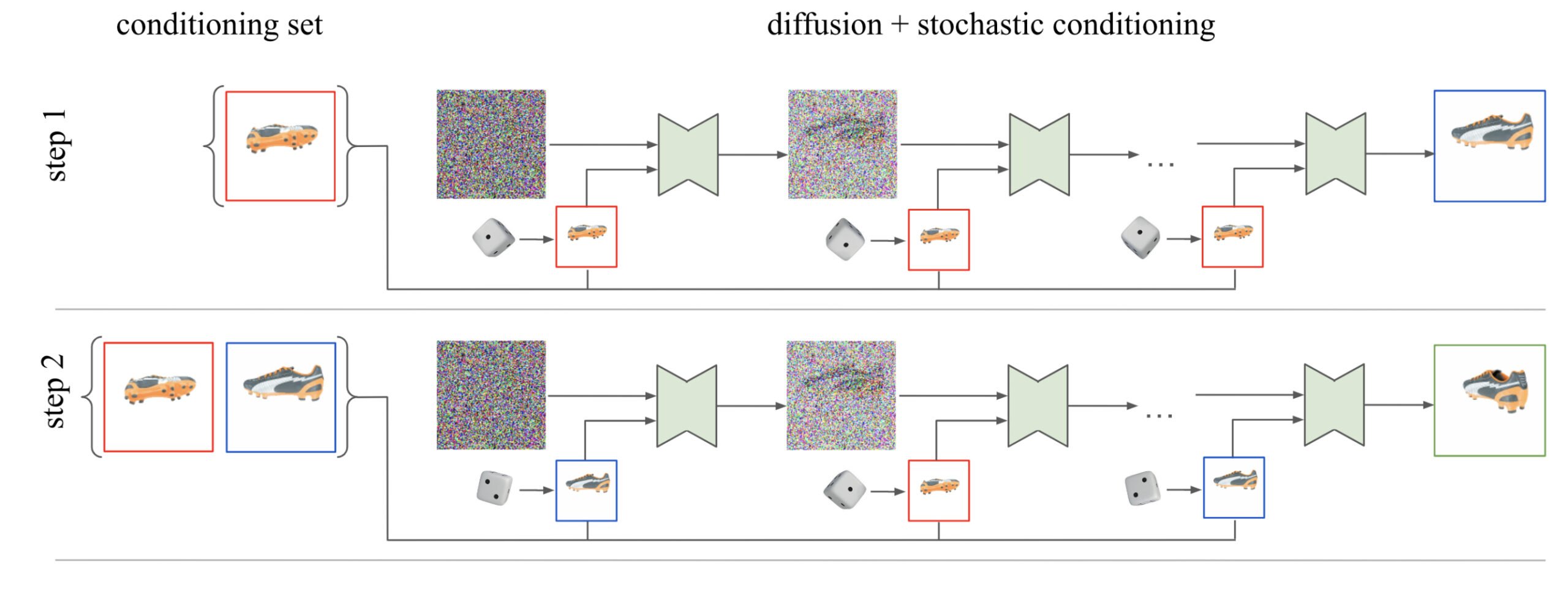
Specifically, during the reverse diffusion process of each image, the model selects a random conditioning image from the set of previous images at each denoising step.
This stochastic conditioning yields much more consistent 3D results, as shown in the generated videos, compared to the naive sampling method that only considers a single previous image, the Google team writes.
Video: Google
The team also trained a 471 million-parameter 3DiM model using the ShapeNet dataset. The model can then generate 3D views for all objects in the dataset.
3DiM uses architectural improvements, Google aims at real-world data use
In addition to stochastic conditioning, 3DiM benefits from some architectural changes to the classic image-to-image UNet architecture. The researchers propose X-UNet, a variant that shares weights between different images, as well as relies on cross-attention.
They show that better results are possible with this modification. 3D diffusion models can thus provide an alternative to other techniques such as NeRFs, which still face quality issues and high computational costs, according to the team.
Next, the team would like to apply the 3D diffusion models' ability to model entire datasets to the largest 3D datasets in the real world. However, more research is needed to overcome typical challenges of such datasets, such as noisy poses or varying focal lengths in the shots, they said.
More examples and information are available on the 3DiM Github page.