Nvidia, Evozyne, InstaDeep, and researchers from TU Munich show new advances in the use of AI in biology at the JP Morgan Healthcare Conference.
Advances in generative AI models for language and images are transforming the market for natural language processing (NLP), art, and design. But underlying technologies such as transformers, diffusion models, or variational autoencoders (VAE), and methods such as unsupervised learning with gigantic amounts of data, are proving themselves outside these domains as well.
One promising application area is bioinformatics, where models such as Deepmind's AlphaFold 2 or Meta's ESMFold predict the structure of proteins, or diffusion models are expected to start a new era in protein design. In 2022 alone, nearly 1,000 scientific papers were published on Arxiv on the use of AI in biology. By 2025, more than 30 percent of new drugs and materials could be discovered systematically using generative AI techniques, according to the Gartner report "Innovation Insight for Generative AI," for example.
Nvidia partners with startups and researchers for advances in bioinformatics
At this year's JP Morgan Healthcare conference, Nvidia is now showcasing the results of two collaborations with startups and researchers: the genomics language model Nucleotide Transformer and the generative protein model ProT-VAE.
The Nucleotide Transformer was created in a collaboration between InstaDeep, recently acquired by Biontech, the Technical University of Munich, and Nvidia. The team trained different model sizes with data from up to 174 billion nucleotides of different species on Nvidia's Cambridge-1 supercomputer, following the recipe for success of large language models such as GPT-3: large models, a gigantic data set, and lots of computing power.
As hoped, the performance of the Nucleotide Transformer increased with model size and data volume. The team tested the model in 19 benchmarks and in 15 achieved equivalent or better performance than other models trained specifically for these tasks. In the future, the transformer is expected to help translate DNA sequences into RNA and proteins, for example.
"We believe these are the first results that clearly demonstrate the feasibility of developing foundation models in genomics that truly generalize across tasks," said Karim Beguir, InstaDeep’s CEO. "In many ways, these results mirror what we have seen in the development of adaptable foundation models in natural language processing over the last few years, and it’s incredibly exciting to see this now applied to such challenging problems in drug discovery and human health."
AI model ProT-VAE generates new proteins
Researchers at the startup Evozyne went a step further: Using Nvidia's BioNeMo platform, they created the generative model ProT-VAE to generate new proteins. While models such as AlphaFold or ESMFold predict the structure of protein sequences, ProT-VAE is designed to derive functions directly from the sequences and thus generates new proteins that perform a specific function.
The ability to engineer proteins with predetermined functions is a central goal of synthetic biology and has the potential to revolutionize fields like medicine, biochemical engineering or the energy sector. The problem: With the naturally occurring amino acids alone, there are significantly more possible proteins than protons in the visible universe.
Evozyne sees the solution in "machine learning guided protein engineering" with ProT-VAE. The model sandwiches a VAE network between an Nvidia pre-trained protein transformer encoder and decoder. The VAE network is then trained for a specific protein family in which new proteins are to be generated. In the generative process, however, the model can further benefit from the comprehensive representations of the ProtT5 transformer, which has processed sequences of amino acids into millions of proteins during Nvidia's training.
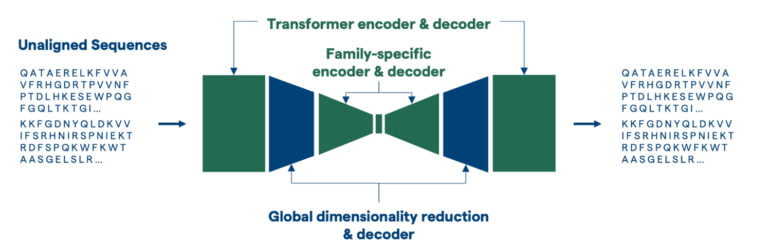
To test their model, the team engineered, among other things, a variant of the human PAH protein. Mutations of the PAH gene can limit its activity and lead to metabolic disorders, such as disrupting mental development and leading to epilepsy. According to the researchers, ProT-VAE designed a variant with 51 mutations, 85 percent sequence similarity, and 2.5-fold improved function.
We anticipate that the model can offer an extensible and generic platform for machine learning-guided directed evolution campaigns for the datadriven design of novel synthetic proteins with “super-natural” function.
From the paper.
Until recently, this process had taken months to years. With ProT-VAE that time was reduced to a matter of weeks.