Deepmind's AdA shows that foundation models also enable generalist systems in reinforcement learning that learn new tasks quickly.
In AI research, the term foundation model is used by some scientists to refer to large pre-trained AI models, usually based on transformer architectures. One example is OpenAI's large language model GPT-3, which is trained to predict text tokens and can then perform various tasks through prompt engineering in a few-shot setting.
In short, a foundation model is a large AI model that, because of its generalist training with large datasets, can later perform many tasks for which it was not explicitly trained.
Deepmind's AdA learns in context
Previous foundation models rely primarily on self-supervised training. Deepmind now introduces an "Adaptive Agent" (AdA), a reinforcement learning agent that has the characteristics of a foundation model.
The Deepmind team has trained AdA in numerous runs in the XLand 3D environment, relying on the intelligent selection of each task: rather than randomly selecting challenges, AdA always trains on tasks that are just above the agent's current skill level. The tasks require skills such as experimentation, navigation, coordination, or the division of labor with other agents.
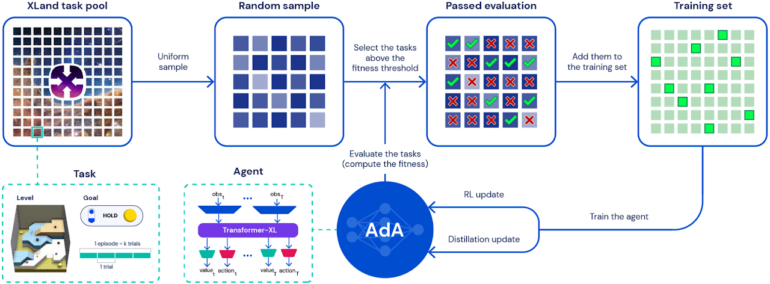
AdA relies on a custom Transformer architecture that allows the agent to store significantly more information, enabling efficient training, according to Deepmind.
In addition, the team is using distillation with a teacher-student approach to accelerate the learning process and train larger models. In the paper, the company trained a model with 265 million parameters and showed that 500 million parameters are possible with the method.
Deepmind AdA learns in XLand 2.0
Training the Transfomer model with millions of runs in the XLand environment produces a foundation RL model, Deepmind writes. AdA exhibits "hypothesis-driven exploration behavior" when exploring new tasks, using the information gained to refine strategies and achieve near-optimal performance.
The process takes only a few minutes, even for difficult tasks, making it human-level, according to Deepmind. Moreover, the whole process is possible without updating the weights in the network. Like GPT-3, AdA has few-shot capabilities, and the learning process takes place in the context window of the model.
In this paper, we demonstrate, for the first time to our knowledge, an agent trained with RL that is capable of rapid in-context adaptation across a vast, open-ended task space, at a timescale that is similar to that of human players. This Adaptive Agent (AdA) explores held out tasks in a structured way, refining its policy towards optimal behaviour given only a few interactions with the task.
From the paper
AdA is based on black-box meta-reinforcement learning and shows, contrary to previous assumptions, that the method is scalable, Deepmind says. Considering the scaling laws of language models or other foundation models, RL models like AdA could become the basis for useful RL models for real-world problems in the future.
More information and examples can be found on the AdA project page.