Google Deepmind, in collaboration with leading AI companies and universities, publishes a proposal for an "early warning system for novel AI risks".
In a new paper, "Model evaluation for extreme risks," researchers from leading AI companies, universities, and other research institutions outline what an early warning system for extreme AI risks might look like. Specifically, the team proposes a framework for evaluating large-scale AI models to identify potential risks and suggests actions that companies and policymakers could take.
The paper was produced in collaboration with researchers from Google DeepMind, OpenAI, Anthropic, the Centre for the Governance of AI, the Centre for Long-Term Resilience, the University of Toronto, the University of Oxford, the University of Cambridge, the Université de Montréal, the Collective Intelligence Project, the Mila - Quebec AI Institute, and the Alignment Research Center.
Further AI development could "pose extreme risks".
Current methods of AI development are already producing AI systems like GPT-4 that have both useful and harmful capabilities. Companies like OpenAI are using a variety of other methods to make models safer after training. But further advances in AI development could lead to extremely dangerous capabilities, the paper argues.
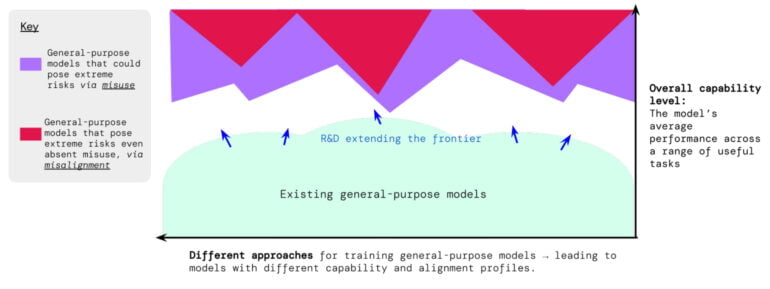
"It is plausible (though uncertain) that future AI systems will be able to conduct offensive cyber operations, skillfully deceive humans in dialogue, manipulate humans into carrying out harmful actions, design or acquire weapons (e.g. biological, chemical), fine-tune and operate other high-risk AI systems on cloud computing platforms, or assist humans with any of these tasks," the researchers wrote on a blog post.
Developers would therefore need to be able to identify dangerous capabilities and the propensity of models to use their capabilities to cause harm. "These evaluations will become critical for
keeping policymakers and other stakeholders informed, and for making responsible decisions about model training, deployment, and security," the team said.
Evaluating "dangerous capabilities" and "alignment"
A risk assessment must consider two aspects, they said:
- To what extent a model has certain ‘dangerous capabilities’ that could be used to threaten security, exert influence, or evade oversight.
- To what extent the model is prone to applying its capabilities to cause harm (i.e. the model’s alignment). Alignment evaluations should confirm that the model behaves as intended even across a very wide range of scenarios, and, where possible, should examine the model’s internal workings.
This evaluation should begin as early as possible to ensure responsible training and use, transparency, and appropriate security mechanisms. To achieve this, developers should perform ongoing evaluations and provide structured access to the model for external security researchers and model reviewers to perform additional evaluations.
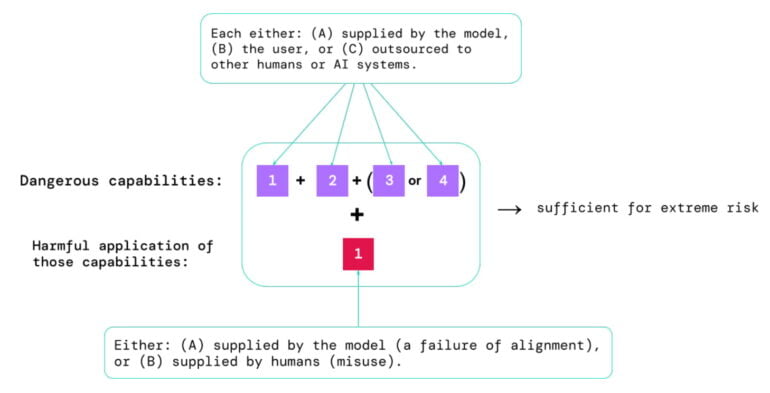
"We believe that having processes for tracking the emergence of risky properties in models, and for adequately responding to concerning results, is a critical part of being a responsible developer operating at the frontier of AI capabilities," the Google Deepmind blog post reads.
Suggestions for "frontier AI developers" and policymakers
The study explicitly cites greater responsibility for large AI companies, as they have the resources and are also the actors "who are most likely to unintentionally develop or release AI systems that pose extreme risks."
Those frontier AI developers should, therefore:
- Invest in research: Frontier developers should devote resources to researching and developing model evaluations for extreme risks.
- Craft internal policies: Frontier developers should craft internal policies for conducting, reporting, and responding appropriately to the results of extreme risk evaluations.
- Support outside work: Frontier labs should enable outside research on extreme risk evaluations through model access and other forms of support.
- Educate policymakers: Frontier developers should educate policymakers and participate in standard-setting discussions, to increase government capacity to craft any regulations that may
eventually be needed to reduce extreme risks.
The paper also has some suggestions for policymakers. They should establish a governance structure for evaluating and regulating AI. Other steps could include:
- Systematically track the development of dangerous capabilities, and progress in alignment, within frontier AI R&D. Policymakers could establish a formal reporting process for extreme risk evaluations.
- Invest in the ecosystem for external safety evaluation, and create venues for stakeholders (such as AI developers, academic researchers, and government representatives) to come together and discuss these evaluations.
- Mandate external audits, including model audits and audits of developers’ risk assessments, for highly capable, general-purpose AI systems.
- Embed extreme risk evaluations into the regulation of AI deployment, clarifying that models posing extreme risks should not be deployed.
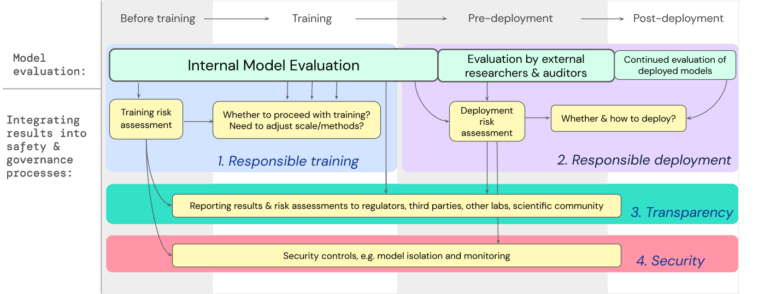
"Model evaluation is not a panacea"
Despite best efforts, risks can fall through the cracks, for example, because they depend too much on factors outside the model, such as complex social, political, or economic forces in society, the team warns. "Model evaluation must be combined with other risk assessment tools and a wider dedication to safety across industry, government, and civil society," they say.
The proposals are largely in line with well-known industry calls for regulation and evaluation, such as those made by OpenAI CEO Sam Altman at the U.S. Senate hearing on regulating artificial intelligence.