3D-LLM integrates understanding of 3D environments into large language models, taking chatbots from the two-dimensional world to the three-dimensional world.
Large language models and multimodal language models can handle speech and 2D images, examples include ChatGPT, GPT-4, and Flamingo. However, these models lack a true understanding of 3D environments and physical spaces. Researchers have now proposed a new approach called 3D LLMs to solve this problem.
3D LLMs are designed to give the AI an idea of 3D spaces by using 3D data such as point clouds as input. In this way, multimodal language models should understand concepts such as spatial relationships, physics, and affordances that are difficult to grasp with 2D images alone. 3D LLMs could enable AI assistants to better navigate, plan, and act in 3D worlds, for example in robotics and embodied AI.
The relationship between the 3D world and language
To train the models, the team needed to collect a sufficient number of 3D and natural language data pairs - such data sets are limited compared to image-text pairs on the Web. Therefore, the team developed prompting techniques for ChatGPT to generate different 3D descriptions and dialogs.
The result is a dataset of over 300,000 3D text examples covering tasks such as 3D labeling, answering visual questions, task decomposition, and navigation. For example, ChatGPT was asked to describe a 3D bedroom scene by asking questions about objects visible from different angles.
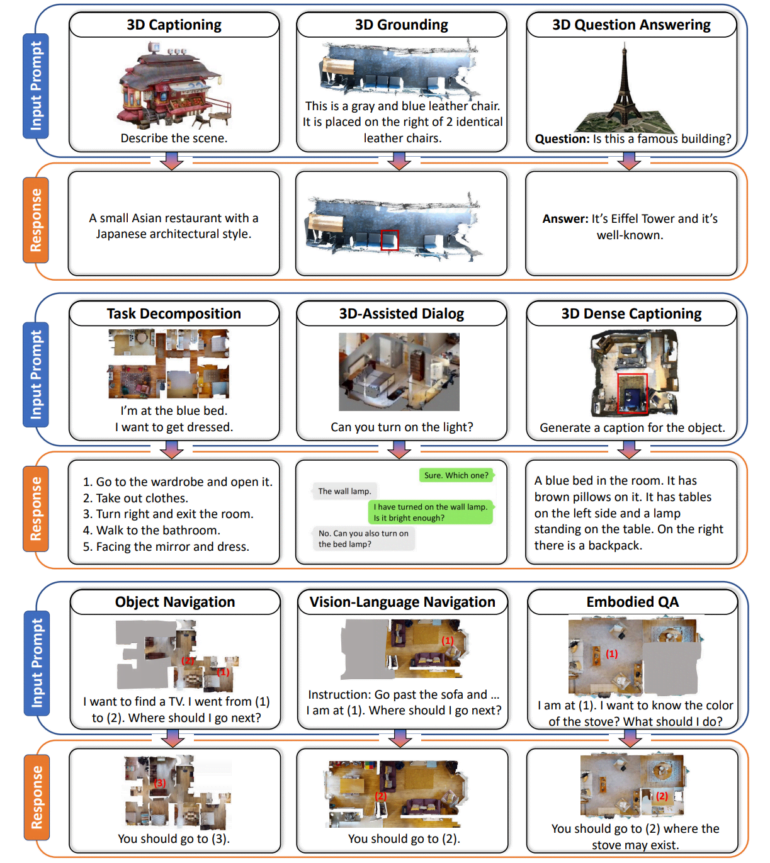
Team connects text descriptions to points in 3D space
The team then developed 3D feature extractors to convert 3D data into a format compatible with pre-trained 2D vision language models such as BLIP-2 and Flamingo.
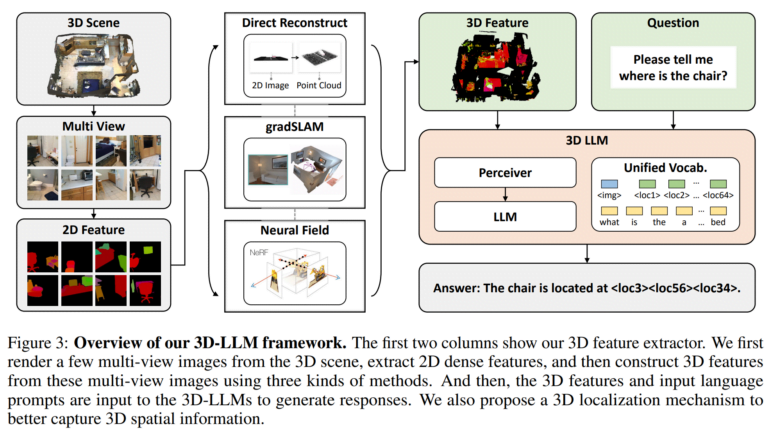
In addition, the researchers use a 3D localization mechanism that allows the models to capture spatial information by associating textual descriptions with 3D coordinates. This also facilitated the use of models such as BLIP-2 to efficiently train 3D LLMs to understand 3D scenes.
Tests with 3D language model show promising results
Experiments showed that the 3D language models were able to generate natural language descriptions of 3D scenes, conduct 3D-aware dialogues, decompose complex tasks into 3D actions, and relate language to spatial locations. This demonstrates the potential of AI to develop a more human-like perception of 3D environments by incorporating spatial reasoning capabilities, according to the researchers.
Video: Hong et al.
The researchers plan to extend the models to other data modalities, such as sound, and train them to perform additional tasks. They also say the goal is to apply these advances to embodied AI assistants that can intelligently interact with 3D environments.