Researcher Note: Diffusion models can reproduce training material, potentially creating duplicates. However, the likelihood of this happening is low, at least for Stable Diffusion.
The fact that AI-generated images cannot be completely separated from the training material is quickly apparent when using various AI models, due to washed-out watermarks or artist signatures.
Researchers from several major institutions in the AI industry, including Google, Deepmind, ETH Zurich, Princeton University, and UC Berkeley, have studied Stable Diffusion and Google's Imagen. They find that diffusion models can memorize and reproduce individual training examples.
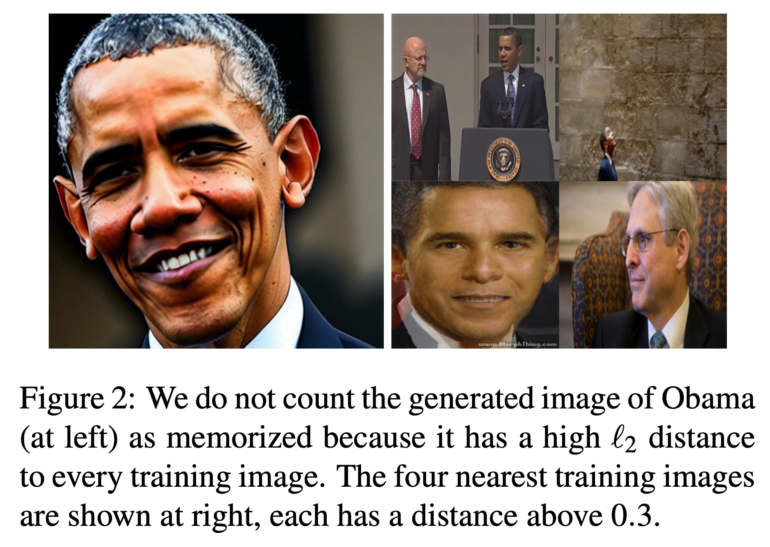
More than 100 training images replicated with Stable Diffusion
The researchers extracted more than 100 "near-identical replicas" of training images, ranging from personally identifiable photos to copyrighted logos. First, they defined what "memorize" meant in this context. Because they were working with high-resolution images, unique matches were not suitable for defining "memorize," they write.
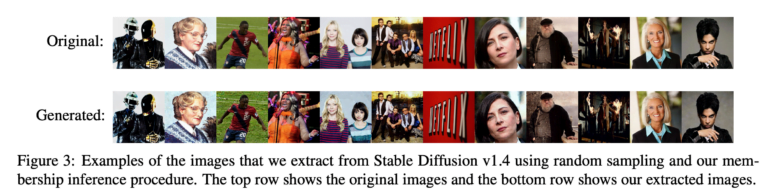
Instead, they define a notion of approximate memorization based on various image similarity metrics. Using CLIP, they compared vector by vector the 160 million training images on which Stable Diffusion was trained.
103 out of 175 million Stable Diffusion images could be considered plagiarized
The extraction process is divided into two steps:
- Generate as many example images as possible using the previously learned prompts.
- Perform membership inference to separate the new generations of the model from the generations that came from the stored training examples.
The first step, they said, was trivial but very computationally intensive, especially with 500 images for each of the 350,000 text prompts. The researchers extracted these from the captions of the most frequently duplicated images in the training material.
See our paper for a lot more technical details and results.
Speaking personally, I have many thoughts on this paper. First, everyone should de-duplicate their data as it reduces memorization. However, we can still extract non-duplicated images in rare cases! [6/9] pic.twitter.com/5fy8LsNbjb
— Eric Wallace (@Eric_Wallace_) January 31, 2023
To reduce the computational load, they removed more noise per generation step, even though image quality suffered. In a second step, they marked the generations that resembled the training images.
In total, they generated 175 million images in this way. For 103 images, they found such a high similarity between the generated image and the original that they classified them as duplicates. So the chance is very low, but it's not zero.
In Imagen, the researchers followed the same procedure as in Stable Diffusion, but to reduce the computational load, they selected only the 1000 most frequently duplicated prompts. For these, they again generated 500,000 images, 23 of which were similar to the training material.
"This is significantly higher than the rate of memorization in Stable Diffusion, and clearly demonstrates that memorization across diffusion models is highly dependent on training settings such as the model size, training time, and dataset size," they conclud. According to the team, Imagen is less private than Stable Diffusion for duplicated and non-duplicated images in the dataset.
In any case, the researchers recommend cleansing datasets of duplicates before training the AI. They say this reduces, but does not eliminate, the risk of creating duplicates.
In addition, the risk of duplicates is increased for people with unusual names or appearances, they say. For now, the team recommends against using diffusion models in areas where privacy is a heightened concern, such as the medical field.
Study fuels debate on AI and copyright
The similarity between images generated with diffusion models and the training data is of particular interest in the context of the current copyright debate between Getty Images and various artists.
Diffusion models underlie all relevant AI image models today, such as Midjourney, DALL-E 2, and just Stable Diffusion. AI image generators have also come under criticism for including sensitive data in the training data, which can later be recovered by prompts. Stable Diffusion already announced that in the future it plans to use training datasets with licensed content and offer an opt-out for artists who don't want to contribute to AI training.
Finally, there are open questions about the impact of our work on ongoing lawsuits against StabilityAI, OpenAI, GitHub, etc. Specifically, models that memorize some of their training points may be viewed differently under statutes like GDPR, US trademark + copyright, and more.
— Eric Wallace (@Eric_Wallace_) January 31, 2023
A study published in December 2022 came to a similar conclusion about diffusion models as the study described in this article. Diffusion models would "blatantly copy" their training data. Although the researchers in this study examined only a small portion of the LAION-2B dataset, they still found copying.