Researchers demonstrate an AI system that can reconstruct semantic content in the form of text from fMRI data.
A brain-computer interface that reconstructs language would have numerous applications in science, medicine, and industry. Invasive methods using recordings from surgically implanted electrodes show that it is possible to reconstruct language for simple brain control.
But these interventions remain dangerous, even though companies like Elon Musk's Neuralink are working on methods to make such interventions as harmless as possible and without consequential damage. Non-invasive language decoders, however, could become commonplace and help people in the future to control technical devices by thought, for example.
Researchers train AI system with 16 hours of fMRI recordings per person
Initial attempts to train language decoders for non-invasive methods have, for example, been made by a team led by Jean-Remi King, a CNRS researcher at the Ecole Normale Supérieure and a researcher at Meta AI.
His team showed in late 2021 that human brain responses to language are predictable based on activations of a GPT language model. In June 2022, the team showed correlations between an AI model trained with speech recordings and fMRI recordings of more than 400 people listening to audiobooks.
More recently, King's team then demonstrated an AI system that can predict which words a person has heard from MEG and EEG data. A new paper by researchers at the University of Texas at Austin now replicates that result for fMRI recordings. In their paper, the team led by author Jerry Tang demonstrates an fMRI decoder that reconstructs intelligible word sequences from perceived language.
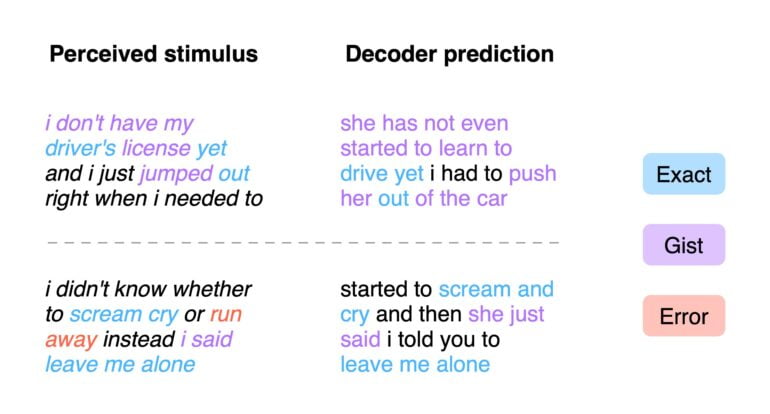
To train the AI system, the team recorded fMRI data from three people who listened to stories for 16 hours. For each person, the data was used to create an encoding model to predict brain responses based on the semantic features of stimulus words.
To reconstruct language from brain data, the decoder predicts a series of possible word sequences in response to data input. If new words are discovered in subsequent data inputs, a language model suggests continuations for each sequence. The coding model evaluates the likelihood of the predictions. The most likely continuations are retained.
AI system can reconstruct the semantic content of silent films
In addition to reconstructing perceived language, the researchers also tested their system with the reconstruction of internal speech: test subjects told themselves a short story in their heads, which the AI system was supposed to reconstruct. In both tests, the quality of the predictions was significantly above chance.
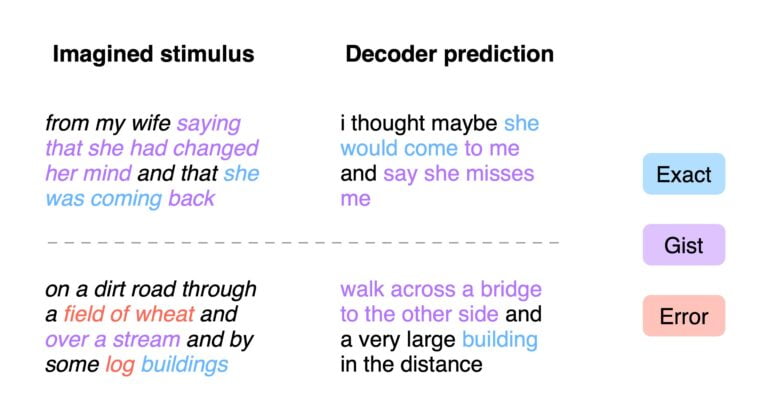
The team said that the "decoded word sequences captured not only the meaning of the stimuli, but often even recovered exact words and phrases."
The quality of the prediction also remained stable across different brain areas measured. According to the researchers, this is an indication that the brain processes semantic information in multiple locations.
To test the limits of the approach, the team showed the subjects a movie without sound and had the AI system translate the measured activities into language. The semantic content thus rendered by the system has a high correspondence with the events visible on the screen.
we pushed the limits of this zero-shot transfer by testing the decoder on brain responses while subjects watched silent movies, and found that the decoder accurately described many movie events. this suggests that our decoder can transfer to non-linguistic semantic tasks! (5/7) pic.twitter.com/z2wyWiOqv5
- Jerry Tang (@jerryptang) September 30, 2022
Does a brain reading system respect privacy?
The team's AI system is still far from a perfect reconstruction of semantic content. The researchers speculate that in the future, better decoders could resolve the inaccuracies. They could, for example, model language stimuli by combining semantic features with lower-level features such as phonemes or acoustics.
In addition, one candidate for improved decoder performance is subject feedback: "Previous invasive studies have employed a closed-loop decoding paradigm, where decoder predictions are shown to the subject in real-time. This feedback allows the subject to adapt to the decoder, providing them more control over decoder output," the paper states.
In one part of the paper, the team also addresses the dangers of the technology. In their experiments, they were able to show that the method shown requires the subjects' cooperation in training and also in using the decoder.
Future developments, however, could allow decoders to circumvent these requirements, the researchers warn. Additionally, inaccurate results could also be intentionally misinterpreted for malicious purposes.
It is therefore critical to raise awareness of the risks such decoding technologies pose to the human brain and to take steps to protect everyone's intellectual privacy, the authors write.