Deepmind's Gato solves many tasks, but none of them really well. Does the new AI system nevertheless lead the way for general artificial intelligence?
Hot on the heels of OpenAI's DALL-E 2, Google's PaLM, LaMDA 2, and Deepmind's Chinchilla and Flamingo, the London-based AI company is showing off another large AI model that outperforms existing systems.
Yet Deepmind's Gato is different: The model can't text better, describe images better, play Atari better, control robotic arms better, or orient itself in 3D spaces better than other AI systems. But Gato can do a bit of everything.
Video: Deepmind
Deepmind's Gato takes what it gets
Deepmind trained the Transformer-based multi-talent with images, text, proprioception, joint moments, keystrokes, and other discrete and continuous observations and actions. In the training phase, all data is processed in a token sequence by the Transformer network, similar to a large language model.
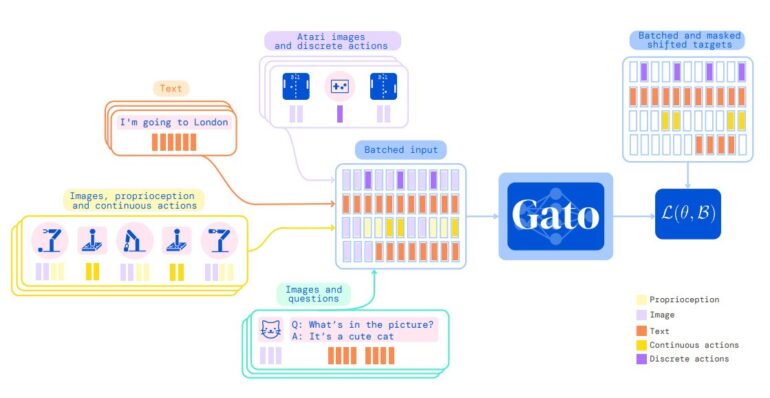
The team then tested Gato on 604 different tasks. In over 450 of them, the AI model achieved about 50 percent of the performance of an expert in the benchmark. But that's far behind specialized AI models that can reach expert levels.
Gato and the laws of scaling
With only 1.18 billion parameters, Gato is tiny compared to the 175 billion parameter GPT-3, the huge 540 billion parameter PaLM model, or the 70 billion parameter "small" Chinchilla.
According to the team, this is predominantly due to the response time of the Sawyer robot arm used - a larger model would be too slow to perform the robotic tasks on current hardware and with the present architecture.
However, these limitations could be easily addressed with new hardware and architecture, the team said. A larger Gato model could train with more data and likely perform the various tasks better.
Ultimately, this could lead to a generalist AI model that replaces specialized models - something the history of AI research also shows, the team says. It cites AI researcher Richard Sutton, who noted as a "bitter lesson" of his research, "Historically, generic models that are better at leveraging computation have also tended to overtake more specialized domain-specific approaches eventually."
Deepmind has also shown that Gato's performance increases with the number of parameters: In addition to the large model, the team trained two smaller models with 79 million and 364 million parameters. The average performance increases linearly with the parameters - at least for the benchmarks tested.
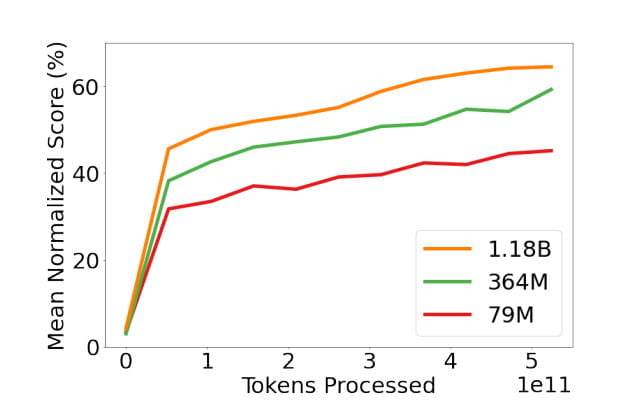
This phenomenon is already known from large-scale language models and was explored in depth in the early 2020s research paper "Scaling Laws for Neural Language Models."
To these scaling laws, Deepmind recently added the importance of larger data sets for scaling performance with the Chinchilla paper. More training data leads to better performance.
Game over or Alt Intelligence?
Will scaling a system like Gato one day enable general artificial intelligence? The confidence in the scaling principle is not shared by everyone: In a new post on Substack about Gato, cognitive scientist and AI researcher Gary Marcus speaks of a failed "Scaling-Uber-Alles" approach. All current large AI models such as GPT-3, PaLM, Flamingo or even Gato would combine moments of brilliance with absolute incomprehension.
While humans are also prone to error, he said, "anyone who is candid will recognize that these kinds of errors reveal that something is, for now, deeply amiss. If either of my children routinely made errors like these, I would, no exaggeration, drop everything else I am doing, and bring them to the neurologist, immediately."
Marcus refers to this line of research as Alt Intelligence: "Alt Intelligence isn’t about building machines that solve problems in ways that have to do with human intelligence. It’s about using massive amounts of data – often derived from human behavior – as a substitute for intelligence."
According to Marcus, this method is not new, but the accompanying hubris of achieving general artificial intelligence simply by scaling is.
With his Substack-Post Marcus is also responding to a Twitter post by Nando de Freitas, research director at Deepmind: "It's all about scale now! The Game is Over," de Freitas said in the context of Gato. "It's about making these models bigger, safer, compute efficient, faster at sampling, smarter memory, more modalities, INNOVATIVE DATA, on/offline, ... ."
De Freitas sees Deepmind on its way to general AI if the scaling problems he describes are solved. Sutton's lesson is not a bitter, but rather a sweet one, according to the Deepmind researcher. De Freitas is expressing here what many in the industry are thinking, Marcus writes.
But more cautious tones are also coming from Deepmind: "Maybe scaling is enough. Maybe," writes lead scientist Murray Shanahan. But he sees little evidence in Gato that scaling alone will lead to human-level generalization. "Thankfully we're working in multiple directions", writes Shanahan.