Aleph Alphas update makes generative AI models of its Luminous family explainable. This opens up new applications for generative AI, even in critical tasks.
German AI startup Aleph Alpha is introducing a new feature for its own Luminous family of generative AI models that aims to make their outputs explainable and verifiable.
Current generative AI models fail to deliver "Explainable AI"
While generative AI models such as ChatGPT or GPT-4 are transforming entire industries, they have one major problem: their outputs are not explainable. While they could still be useful in critical areas such as medicine, their lack of explainability poses many dangers.
Explainable AI (XAI) methods try to solve this problem and make the outputs of those AI models explainable and verifiable.
In early 2023, researchers from German AI startup Aleph Alpha, TU Darmstadt, the Hessian.AI research center, and the German Research Center for Artificial Intelligence (DFKI) presented AtMan, an XAI method that makes the generations of such transformer-based models for text and images explainable.
Luminous can now withhold output without trusted sources
AtMan is now available for Luminous models and can be applied to text and images, according to Aleph Alpha. This is an important step toward greater explainability and verifiability- a regulatory requirement for generative AI models that is likely to come soon with the EU AI Act.
"This transparency will enable the use of generative AI for critical tasks in law, healthcare, and banking - areas that rely heavily on trusted and accurate information," said Jonas Andrulis, CEO and founder of Aleph Alpha.
With the new feature, Aleph Alpha says it can infer facts in model output, but also directly withhold output without appropriate trusted sources.
"Our customers often have vetted internal knowledge of great value. We can now build on that and ensure that an AI assistant successfully uses only that knowledge and always provides context," Andrulis said.
How does AtMan work?
Aleph Alpha's AtMan uses a perturbation method to analyze the effect of one token on the generation of another. This is done by using perturbations to measure how the synthesis of a token changes due to the suppression of other words. An illustrative example shows how the sentence "Ben likes to eat burgers and" is continued with the word "fries".
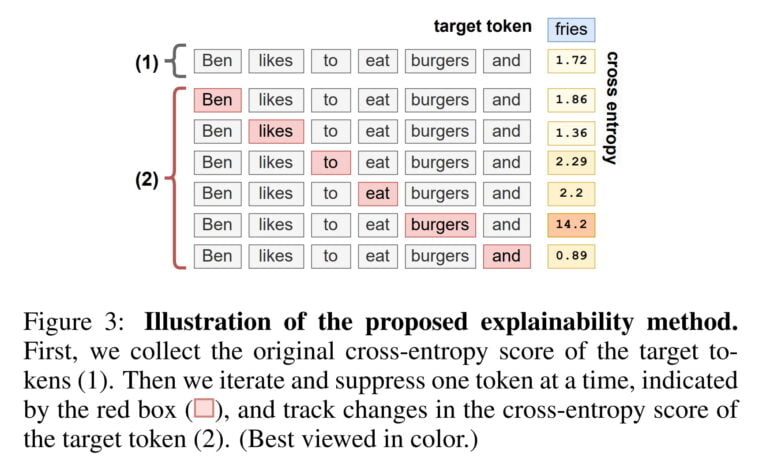
The cross-entropy value calculated by AtMan is used to determine how much the sentence as a whole influenced the generation of the word "fries". Then, each word is systematically suppressed to see how the cross-entropy value changes. In this example, the value increases significantly when the word "burger" is suppressed. The XAI method successfully identifies the word that has the greatest influence on the synthesis of "fries".
With EU privacy regulators taking a closer look at OpenAI's ChatGPT and the looming EU AI law, the U.S. company will likely have to adapt similar technologies to ensure its models remain available in the EU market.