Researchers have investigated the rationality of seven large AI language models using cognitive tests from psychology.
Can AI language models reason? A study by researchers at University College London set out to find out, using cognitive tests from psychology. They tested seven major language models, including OpenAI's GPT-3.5 and GPT-4, Google's LaMDA, Anthropic's Claude 2, and three versions of Meta's Llama 2.
The tests were originally designed to reveal cognitive biases and heuristics in human thinking. People often answer incorrectly because they take mental shortcuts instead of reasoning strictly logically.
The researchers wanted to know: Do the AI models show similar irrational reasoning to humans? Or do they reason illogically in their own way?
"The capabilities of these models are extremely surprising, especially for people who have been working with computers for decades, I would say," says Professor Mirco Musolesi, author of the study. "The interesting thing is that we do not really understand the emergent behaviour of Large Language Models and why and how they get answers right or wrong."
GPT-4 is more often correct and makes more human-like mistakes
The language models' responses were scored in two dimensions: correct or incorrect and human-like or non-human-like. A human-like incorrect response is one that makes errors similar to those made by humans. A non-human incorrect response is illogical in a different way.
The result: AI models often produce irrational outputs - but differently from humans. Most of the incorrect answers were not human-like, but irrational in their own way. Sometimes the explanation was correct, but the conclusion was wrong. There were often mathematical errors or violations of logic and probability rules. Their performance was also inconsistent: The same model often gave completely different answers to the same problem. Sometimes right, sometimes wrong, sometimes logical, sometimes illogical.
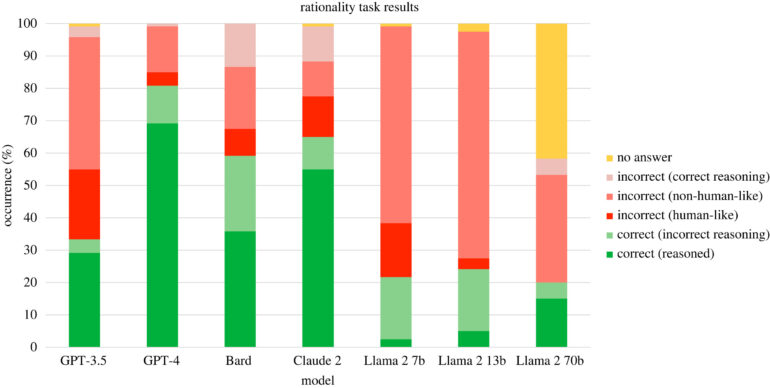
Overall, OpenAI's GPT-4 performed best. It gave correct answers with correct explanations 69.2% of the time. Claude 2 followed with 55%. Meta's Llama 2, with 7 billion parameters, performed the worst - getting it wrong 77.5% of the time.
According to the study, OpenAI's GPT-4 model was the most human-like in its responses to the cognitive tasks. GPT-4 gave human-like answers (both correct and incorrect) 73.3% of the time.
Authors call for caution in using language models in critical areas
The authors emphasize that most of the incorrect answers given by the language models are not due to human-like cognitive biases, but to other errors such as inconsistent logic or incorrect calculations. They suggest that the human-like biases in large language models such as GPT-4 are due to the training data rather than human-like reasoning.
The inconsistent and sometimes irrational results raise questions about the use of such systems in critical areas such as medicine. The study provides a methodology for evaluating and comparing the rationality of AI language models. This could be a starting point for improving the safety of these systems in terms of logical reasoning.
"We now have methods for fine-tuning these models, but then a question arises: if we try to fix these problems by teaching the models, do we also impose our own flaws? What’s intriguing is that these LLMs make us reflect on how we reason and our own biases, and whether we want fully rational machines," says Musolesi. "Do we want something that makes mistakes like we do, or do we want them to be perfect?"