The conventional wisdom in AI development used to be simple: bigger models meant better performance. It's still not wrong, but it's also being challenged as the latest generation of AI language models achieves similar results with far fewer parameters.
According to new research from EpochAI, GPT-4o operates with approximately 200 billion parameters, while Claude 3.5 Sonnet uses around 400 billion parameters. These numbers stand in stark contrast to the original GPT-4, which reportedly contained 1.8 trillion parameters.
Size estimates based on performance metrics
Since some AI companies no longer publicly disclose their closed model sizes, EpochAI based these estimates on text generation speed and usage costs. GPT-4o, for example, generates between 100 and 150 tokens per second and costs $10 per million tokens—making it significantly faster and more cost-effective than the original GPT-4.
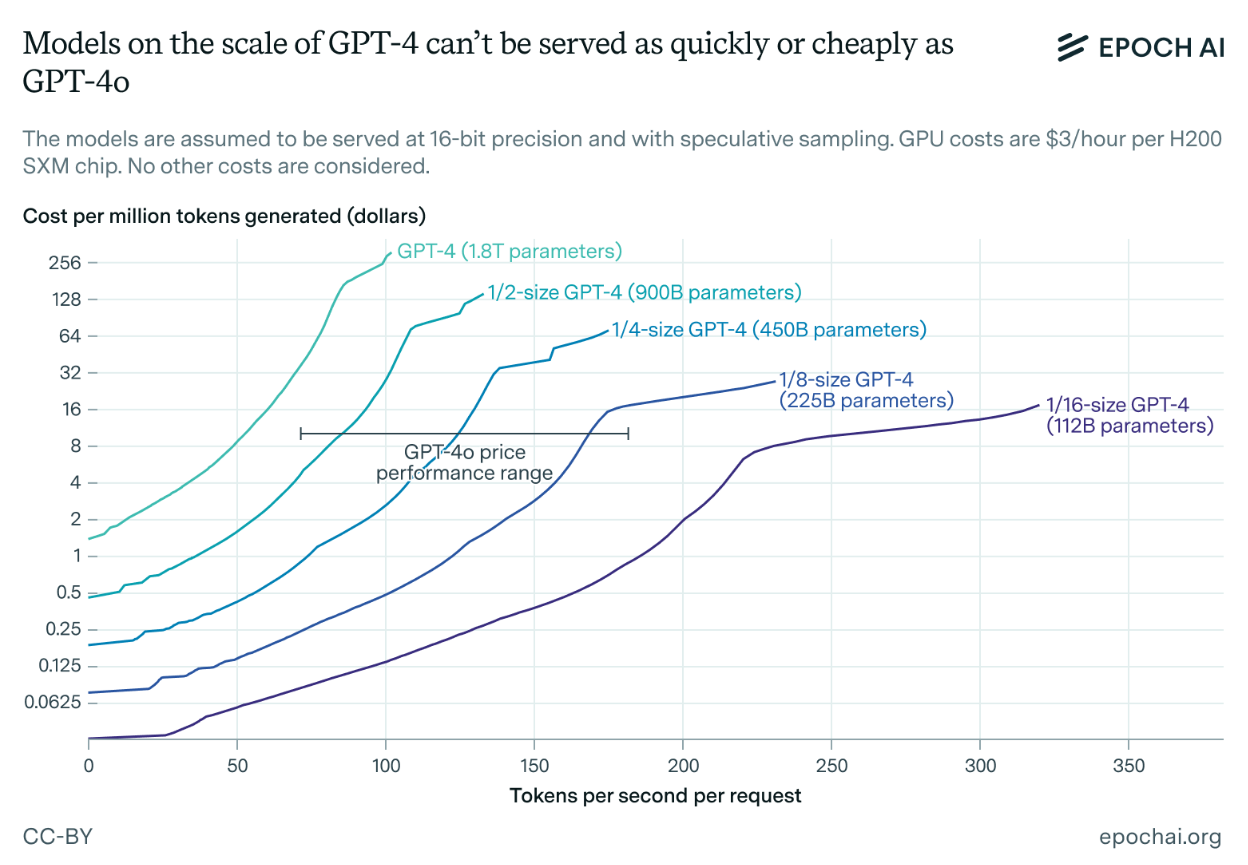
EpochAI identified four main reasons for this trend toward smaller models. First, unexpected high demand for AI services forced providers to create more efficient systems. Second, a process called "distillation" allows larger models to train smaller ones while maintaining similar performance levels.
Third, the adoption of Chinchilla scaling laws led companies to train models with fewer parameters on larger datasets. Test-time compute scaling also contributes to more efficient model designs. Fourth, improved "in-context reasoning" methods using synthetic data now enable smaller models to handle complex tasks effectively.
Altman predicted the end of size race
OpenAI CEO Sam Altman anticipated this shift shortly after GPT-4's release in April 2023. He compared the race for more parameters to the historical pursuit of higher processor clock speeds—a development that proved to be a dead end. Notably, GPT-4 was OpenAI's first model for which the company didn't disclose the parameter count.
Looking ahead, EpochAI expects the next generation of language models, including GPT-5 and Claude 4, to match or slightly exceed the original GPT-4's size. Models with 1 to 10 trillion parameters might perform better and be more cost-effective than larger 100-trillion-parameter models, even if technically feasible, thanks to test-time compute scaling. The analysts predict slower growth over the next three years, with model sizes increasing by less than a factor of 10—a slower rate than the jump from GPT-3 to GPT-4.
Former OpenAI chief scientist Ilya Sutskever recently pointed to limited training data as a key constraint on AI development. Altman previously said in June that while there is enough data for the next generation of AI, future advances will require both higher-quality data and more efficient learning methods.