Nvidia's Computex presentation shows why the scaling of large AI models has only just begun. But will it solve the problems of today's models?
Nvidia's Computex 2024 presentation in Taiwan gave an outlook on its GPU and interconnect roadmap up to 2027. In his keynote, CEO Jensen Huang emphasized the need to continuously increase performance while reducing training and inference costs so every company can use AI.
Huang compared the development of GPU performance over eight years: between the "Pascal" P100 and the "Blackwell" B100 GPU generations, to be delivered this year, performance increased by more than 1,000 times. Much of this is due to reducing floating-point precision. Nvidia relies heavily on FP4 for Blackwell.
This performance boost should make it possible to train a model like GPT-4 with 1.8 trillion parameters on 10,000 B100 GPUs in just ten days, according to Huang.
However, GPU prices have also risen by a factor of 7.5 over the past eight years. A B100 is expected to cost between $35,000 and $40,000. Still, the performance increase far exceeds the price increase.
Scaling beyond model size: training data and iterations
The supercomputers planned with the new hardware create the basis for further scaling of AI models. This scaling not only involves increasing the number of parameters, but also training AI models with significantly more data. Especially multimodal datasets that combine different types of information such as text, images, video, and audio will determine the future of large AI models in the coming years. OpenAI recently introduced GPT-4o, the first iteration of such a model.
In addition, the increased computing power enables faster iterations when testing different model parameters. Companies and research institutions can thus more efficiently determine the optimal configuration for their use cases before starting a particularly compute-intensive final training run.
The acceleration also affects inference: real-time applications such as OpenAI's GPT-4o language demos become possible.
Competitors such as Elon Musk's xAI will also train such models. He recently announced that his AI startup xAI plans to put a data center with 300,000 Nvidia Blackwell B200 GPUs into operation by summer 2025. In addition, a system with 100,000 H100 GPUs is to go online in a few months. Whether his company can implement these plans is unclear. The costs are likely to be well over $10 billion. This is still well below the 100 billion that OpenAI and Microsoft are reportedly estimating for Project Stargate.
More scaling - but the same problems?
However, it is unclear whether scaling can also help solve fundamental problems of today's AI systems. These include, for example, unwanted hallucinations where language models generate convincing but factually incorrect answers.
Some researchers, including the authors of the "Platonic Representation Hypothesis," suspect that using large amounts of multimodal training data could help here. Meta's AI chief Yann LeCun and Gary Marcus, on the other hand, consider such hallucinations and the lack of understanding of the world to be a fundamental weakness of generative AI that cannot be remedied.
With the hardware soon available, these questions can be answered experimentally - reliable models would be in the interest of companies, as they would enable use in many critical application areas.
Next step: "Physical AI"?
A possible path could be AI models that understand more about the physical world: "The next generation of AI needs to be physically based, most of today's AI don't understand the laws of physics, it's not grounded in the physical world" Huang said in his presentation.
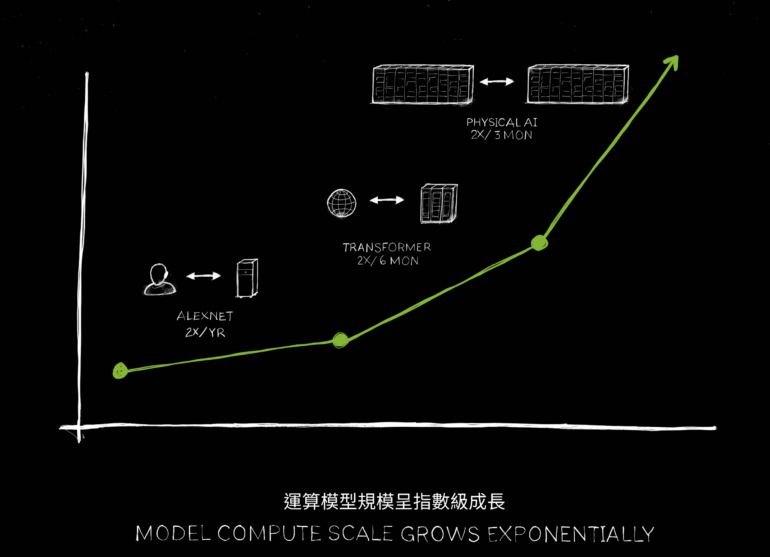
He expects such models to not only learn from videos like OpenAI's Sora, but also learn in simulations and from each other - similar to systems like DeepMind's AlphaGo. "It stands to reason that the rate of data generation will continue to advance and every single time data generation grows the amount of computation that we have to offer needs to grow with it. We are to enter a phase where AIs can learn the laws of physics and understand and be grounded in physical world data, and so we expect that models will continue to grow, and we need larger GPUs as well."
Annual chip updates to maintain Nvidia's dominance
This increase in required computing power is of course in the company's interest - and Nvidia also announced details of the next GPU generations: "Blackwell Ultra" (B200) is to follow in 2025, "Rubin" (R100) in 2026, and "Rubin Ultra" in 2027. Added to this are improvements in the associated interconnects and network switches. "Vera," a successor to Nvidia's "Grace" Arm CPU, is also planned for 2026.
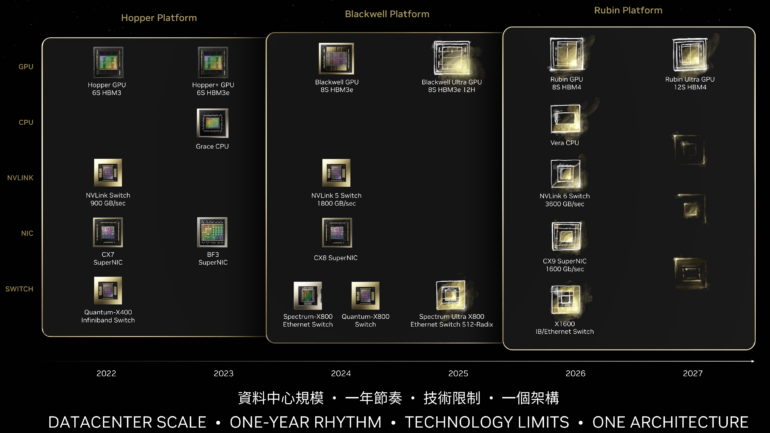
With Blackwell Ultra, Rubin and Rubin Ultra, Nvidia wants to further expand its dominance in the hardware market for AI applications through annual updates. The company has already achieved a quasi-monopoly position here with the Hopper chips. AMD and Intel are trying to shake this dominance with their own AI accelerators.