A new study argues that different AI models converge on common representations as they grow. The convergent representation could be a statistical model of the underlying reality.
Various AI models form increasingly similar data representations as they become more powerful and larger. This is the result of a study by researchers at MIT. They hypothesize that this convergence leads to a universal representation of reality.
In their paper, "The Platonic Representation Hypothesis," the team uses a variety of experimental observations to show that artificial neural networks trained with different goals on different data and modalities, such as images and text, seem to converge on a common statistical model of reality in their representation spaces.
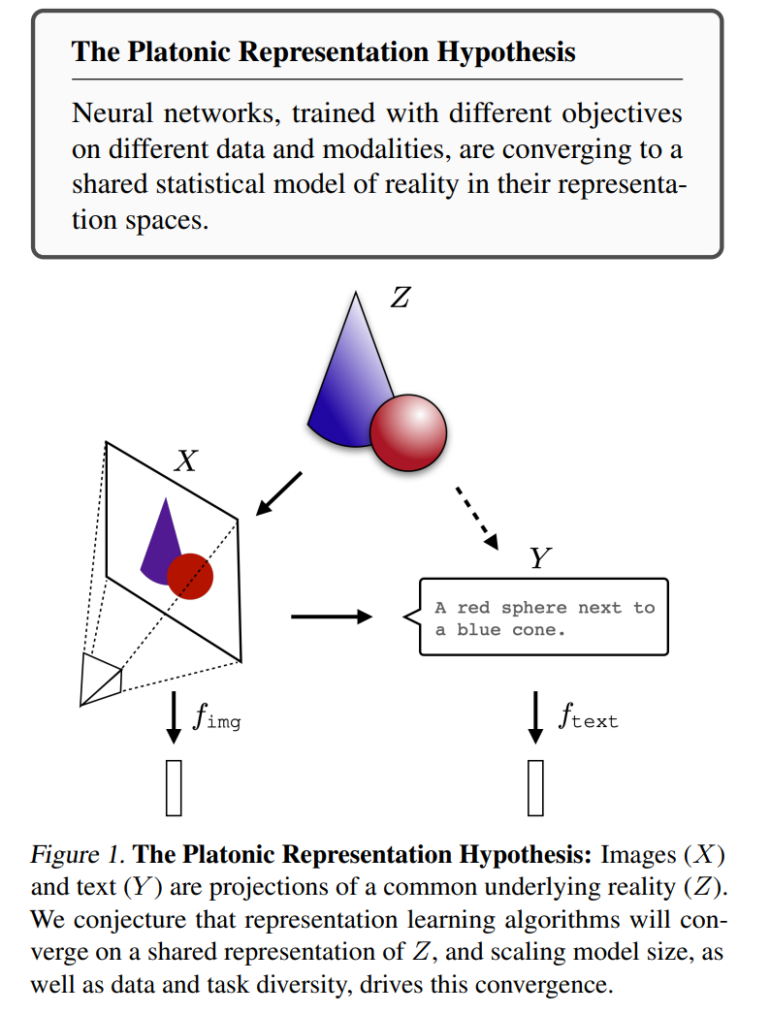
According to this, different models all try to find a representation of reality - that is, a representation of the common distribution of events in the world that generate the observed data. The larger the models become and the more data and tasks they cover, the more their internal representations converge.
The researchers call this hypothetical converged representation "platonic representation" referencing Plato's cave allegory and his idea of an ideal reality underlying our sensory impressions.
Evidence for this convergence comes from observations that models with different architectures and training data arrive at similar representations. For example, layers can be exchanged between models with similar performance without severely affecting performance. Moreover, the convergence seems to increase with increasing model size and performance.
Interestingly, the convergence even seems to take place across modalities: representations of visual models and language models are becoming increasingly similar. There are also growing similarities to representations in the human brain.
Scaling could reduce hallucinations
The researchers suspect that the convergence process is favored by several factors: On the one hand, scaling to more data and tasks leads to an ever smaller solution space. On the other hand, larger models are more likely to find a common optimum. Finally, deep nets naturally prefer simple solutions, which further drives convergence.
From their hypothesis, they derive several implications:
- Training data can be shared across modalities. To train the best language model, one should also use image data - and vice versa.
- Translation and adaptation between modalities is facilitated. Language models would achieve a certain degree of anchoring in the visual domain even without cross-modal data.
- Scaling could reduce hallucinations and bias. As scaling increases, the models should reinforce data bias less.
However, the researchers also discuss limitations of their hypothesis. For example, different modalities may contain different information, so that complete convergence is not possible. Also, in some domains such as robotics, there are no standardized representations yet.