TikTok company Bytedance demonstrates a new image generation method that can blend two semantic concepts into a new one.
"Semantic mixing" is what the Bytedance research team calls the process of instructing a diffusion model to mix two semantic concepts into a new one: For example, a watermelon and a lamp become a lamp in the shape of a watermelon. A tiger and a rabbit become a rabbit with tiger stripes.
The researchers describe semantic mixing as a difficult problem because even humans could not always tell exactly what the result of such mixing might be.
Melon lamps and tiger rabbits
Unlike style transfer, in which the image content remains unchanged and only the style is adapted (a rendered graphic becomes a drawn graphic, for example), semantic mixing creates new motifs. The layout and geometry of the original image are preserved, which contributes to greater precision and stability in image generation.
The Bytedance team uses Stable Diffusion 1.4 as the underlying image AI for the MagicMix process. The diffusion model, which extracts images from noise, first generates the layout and shape of an image. Only later in the denoising process does the semantic concept follow.
MagicMix takes advantage of this two-step approach: Bytedance also first determines the rough layout of the subject based on an image or text. Then it switches the prompt to the second semantic concept during generation. No spatial mask or relearning is required.
AI image generation becomes more flexible
The process even works in reverse: MagicMix can remove semantic concepts from images. However, the results are rather bizarre compared to concept mixing. For example, a cat image without a cat concept becomes a strange chameleon. A dog picture without a dog becomes a sphinx-like cat.
Mixing animal breeds is interesting. The aforementioned tiger rabbit is a fancy example, but it can also be done in more realistic ways, such as crossing dog breeds. Here we can see that the mixed concepts can reflect reality. Fictional motifs are also possible, which creatives could use as inspiration for their work.

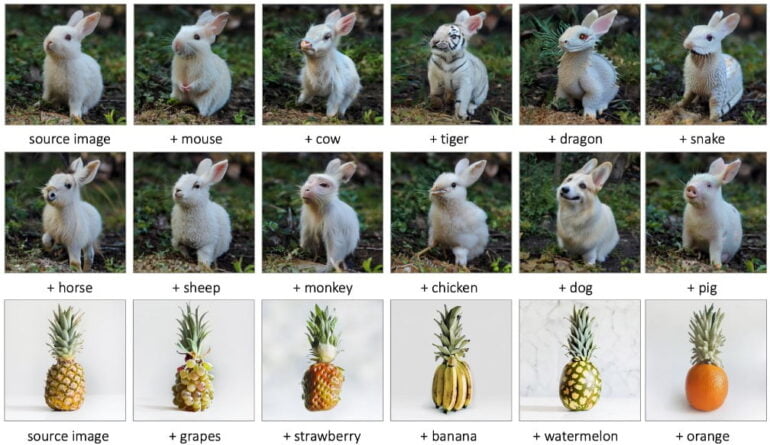
One of MagicMix's strengths, its ability to maintain the layout, is also a weakness: the method fails when it mixes content concepts without similarity in form. Mix a van with a cat, and instead of a cat with tires or a van with whiskers, you get a cat on a van or … well.
Despite this limitation, the Bytedance team is convinced that MagicMix will create further opportunities and scope for the use of image AI systems.
Thanks to the strong capability in generating novel concepts, our MagicMix supports a large variety of creative applications, including semantic style transfer(e.g., generating a new sign given a reference sign layout and a certain desired content), novel object synthesis (e.g., generating a lamp that looks like a watermelon slice), breed mixing (e.g., generating a new species by mixing “rabbit” and “tiger”) and concept removal (e.g., synthesizing a non-orange object that looks like an orange). Although the solution is simple, it paves a new direction in the computational graphics field and provides new possibilities for AI-aided designs for artists in a wide field, such as entertainment, cinematography, and CG effects.
Excerpt from the paper
Because MagicMix is based on Stable Diffusion, which in turn is trained with a LAION dataset, among others, the Bytedance team points to "social and cultural biases" in image generation.