Generative AI systems like Stable Diffusion require costly retraining to learn new concepts. Google's Re-Imagen takes a more efficient approach.
OpenAI's DALL-E 2 or Midjourney generate high-quality images based on text. Their generative capabilities, however, are limited to objects or styles that are part of the companies' training data.
A popular alternative is therefore the Open-Source model Stable Diffusion. It runs locally on a user's graphics card or in the cloud and - thanks to some fine-tuning techniques - can learn new concepts such as styles, objects, or people, provided the appropriate hardware resources are available.
Initial attempts used Textual Inversion as a method for post-training, but Dreambooth has now become the standard. The method developed by Google to personalize large text-to-image models like Imagen has been adapted by the open-source community for Stable Diffusion.
Stable Diffusion can be personalized with your own images, but it is a laborious approach
Dreambooth lets you customize Stable Diffusion to your own needs with sample images. The method achieves good results with just a few images. While the hardware requirements were extreme at the beginning, optimized Dreambooth versions can be run with Nvidia graphics cards with 10 gigabytes of VRAM.
Dreambooth is becoming popular for creating custom Stable Diffusion models using your images.
Here is a beginner friendly thread on how it works: ? pic.twitter.com/jlLdOqbWBf
- Divam Gupta (@divamgupta) November 1, 2022
In the Dreambooth paper, Google compares DALL-E 2 and Imagen to show how the method allows a generative AI model to learn, say, a new clock face for an alarm clock.
The results are impressive, and numerous people on Twitter are sharing examples of how extensively Dreambooth can be used. So Dreambooth solves a key problem of generative AI models: they often struggle to generate images of unusual and unfamiliar objects or styles. But the method is not necessarily scalable, as each concept requires computationally intensive training.
Google's Re-Imagen shows a scalable alternative to Dreambooth
A group at Google is now demonstrating the Retrieval-Augmented Text-to-Image Generator (Re-Imagen). This new method allows a generative AI model to generate images of rare or never-before-seen objects.
As the name (Retrieval-Augmented) implies, Re-Imagen retrieves new information from an external database rather than being re-trained with additional data.
Given a text prompt, Re-Imagen accesses an external multi-modal knowledge base to retrieve relevant (image, text) pairs, and uses them as references to generate the image.
From the paper
Re-Imagen retrieves semantic and visual information about unknown or rare objects via the additional input, improving its accuracy in image generation.
To accomplish this, the Google team trained Re-Imagen with a new dataset that includes three modalities (image, text, and retrieval). Thus, the model learned to use text input and retrievals of the external database for a generation.
In some examples, Google shows that Re-Imagen achieves significantly better results for rare or unknown objects than Imagen, DALL-E 2, or Stable Diffusion.
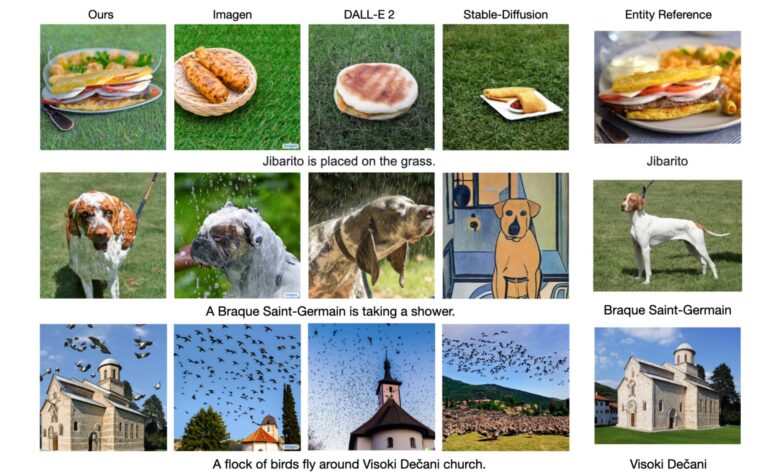
However, the new method also has disadvantages:
First, because Re-Imagen is sensitive the to retrieved image-text pairs it is conditioned on, when the retrieved image is of low-quality, there will be a negative influence on the generated image. Second, Re-Imagen sometimes still fail to ground on the retrieved entities when the entity’s visual appearance is out of the generation space. Third, we noticed that the super-resolution model is less effective, and frequently misses low-level texture details of the visual entities.
From the paper
The team plans to investigate these limitations further and address them in future work.