Researchers at East China Normal University and Microsoft Research Asia have been studying how large language models such as GPT-4 perform in dynamic, interactive scenarios.
The team wanted to find out how well language models could make choices in rapidly changing contexts that reflect the ever-changing strategies of the business and financial world, where market fluctuations, resource scarcity, and other factors make decisions difficult.
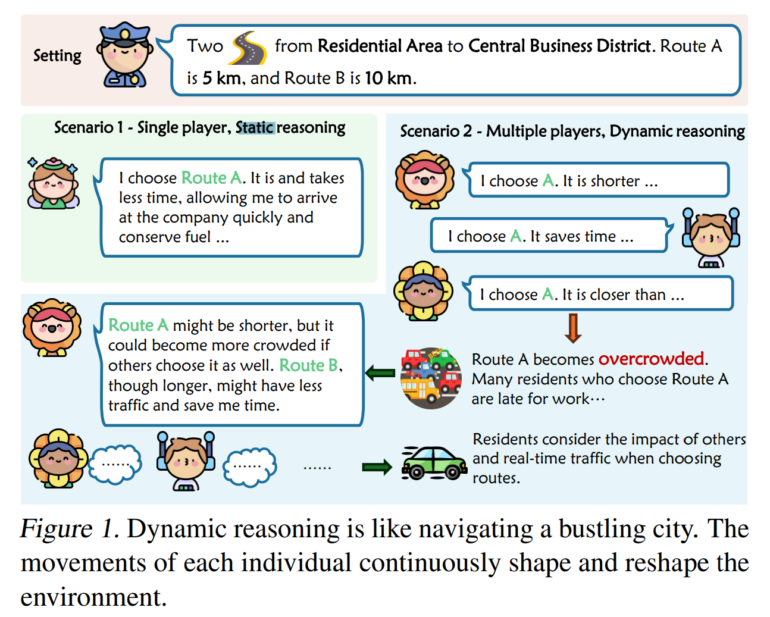
The study shows that traditional reasoning methods such as chain-of-thought, which work well for static reasoning tasks, fail in these dynamic environments. The researchers therefore developed a new method that improves the performance of language models in such tasks.
The researchers used their concept of "K-level reasoning" for language models, a new approach based on the principles of game theory. It is based on the idea of "k-level reasoning," in which a player not only thinks about his own strategy, but also tries to predict his opponent's moves. The method uses large language models to take the opponent's perspective and recursively simulate their possible moves. This process takes into account historical information, allowing the AI to make more informed decisions.
K-level reasoning vs. chain-of-thought
The method was tested on two games using GPT-4 and GPT-3.5: "Guessing 0.8 of the Average" (G0.8A) and "Survival Auction Game" (SAG). In the first game, participants must choose a number between 1 and 100, with the winner choosing the number closest to 80% of the average of all numbers chosen. In the second game, participants bid for water resources to survive a fictional drought period, balancing their health points and financial resources.
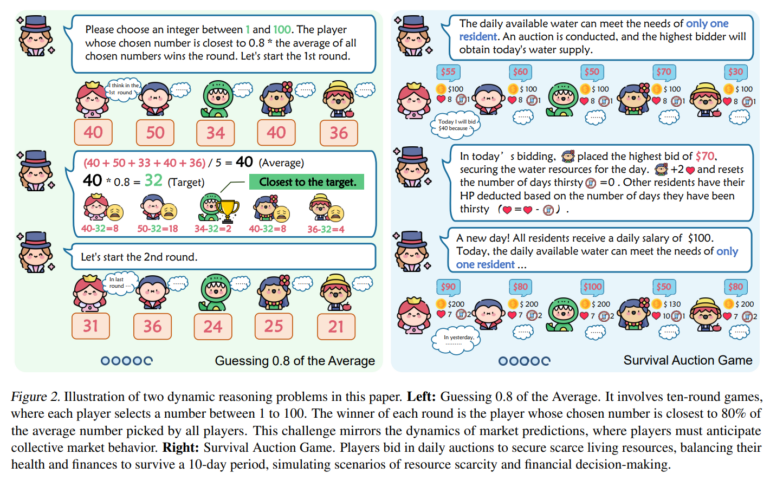
The K-Level Reasoning method showed superior performance compared to other approaches. It led to a higher win rate in the games and showed better adaptability to changing conditions. The method was also able to predict the opponent's actions more accurately and thus make strategically smarter decisions.
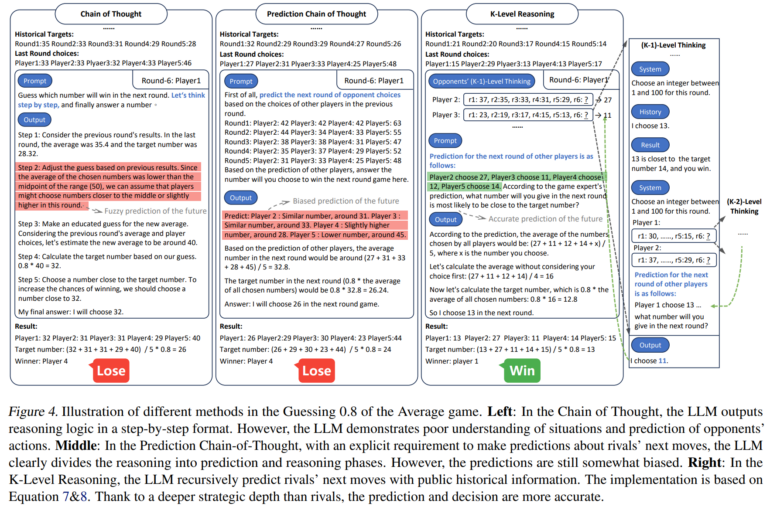
The team sees their work as a template for further testing of language models in such complex scenarios, but also as an indication of the untapped potential of language models.