SDFusion is an AI framework for generating 3D assets that can process images, text, or shapes as input.
Generative AI models for 3D assets could change workflows in industry or help untrained users to create their own virtual objects and worlds. Nvidia CEO Jensen Huang, for example, sees this AI-powered creative process as central to the Metaverse future.
Current AI systems use neural rendering methods such as NeRFs, which learn 3D objects based on different views, or generate NeRFs via text input, like Google's Dreamfusion. Other methods, such as CLIP-Mesh, create meshes from text input using diffusion models.
Researchers at the University of Illinois Urbana Champagne and Snap Research are now demonstrating SDFusion, a multimodal AI framework for 3D assets.
SDFusion processes text, images and shapes
Existing 3D AI models provide compelling results, but training is often time-consuming and neglects available 3D data, the researchers say.
The team, therefore, proposes a collaborative paradigm for generative models: Models trained on 3D data provide detailed and accurate geometries. Models trained on 2D data provide different appearances.
Following this paradigm, the team developed SDFusion, a diffusion-based generative model for 3D assets that can also process multimodal input such as text, images, or 3D shapes. The 3D objects can be additionally textured through the interaction of generative 3D and 2D AI models.
Video: Image: Cheng et al.
SDFusion thus allows users to create 3D assets with incomplete shapes, images, and text descriptions simultaneously. This allows for more precise control of the generative process. For example, a photo of a chair with a single leg can be blended with four digital chair legs to create a chair with four legs. The seat from the photo gets carried over.
SDFusion learns multimodally
During training, SDFusion's diffusion model learns from 3D models and via encoders that can process text and images. After training, the team can also regulate the relevance of individual inputs such as text description, image, or 3D shape to generate different 3D assets.
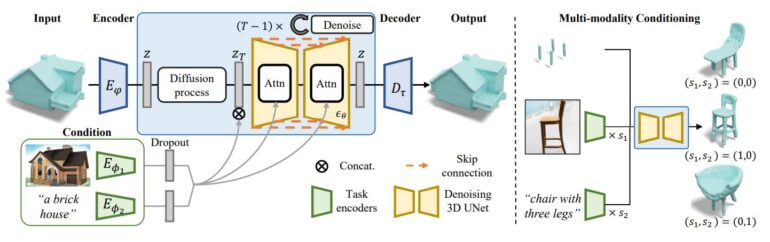
In tests, SDFusion can outperform alternatives tested by the team: "SDFusion generates shapes of better quality and diversity, while being consistent with the input partial shapes." This is true for the completion of given shapes, single-view 3D reconstruction, text-guided generation, and multi-conditional generation.
Despite the good results, however, there is still much to improve, the team writes. For example, a model that can work with numerous 3D representations would be desirable, they say. SDFusion works exclusively with high-quality SDFs, similar to Nvidia's 3D MoMa. Another area of research would also be to use SDFusion in more sophisticated scenarios, such as generating entire 3D scenes.
You can find more information and examples on the SDFusion project page.