After Alpaca comes Vicuna, an open-source chatbot that, according to its developers, is even closer to ChatGPT's performance.
Vicuna follows the "Alpaca formula" and uses ChatGPT's output to fine-tune a large language model from Metas LLaMA family. The team behind Vicuna includes researchers from UC Berkeley, CMU, Stanford, and UC San Diego.
While Alpaca and other models inspired by it are based on the 7-billion-parameter version of LLaMA, the team behind Vicuna uses the larger 13-billion-parameter variant.
For fine-tuning, the team uses 70,000 conversations shared by users on the ShareGPT platform using OpenAI's ChatGPT.
Vicuna training costs half that of Alpaca
The cost of Vicuna was about $300 - half the cost of Alpaca, despite being almost twice the size. The reason: the scraped ShareGPT data is freely available, while Stanford generated its own data via the OpenAI API. Therefore, Vicuna only has training costs. Like the Stanford model, Vicuna is released for non-commercial use only.
In tests with benchmark questions, Vicuna shows significantly more detailed and better-structured answers than Alpaca after fine-tuning with ShareGPT data. They are on a comparable level with ChatGPT, the team writes.
To better assess their chatbot's performance, the team is using GPT-4, the latest model from OpenAI, which is able to provide consistent rankings and detailed scores when comparing different chatbots.
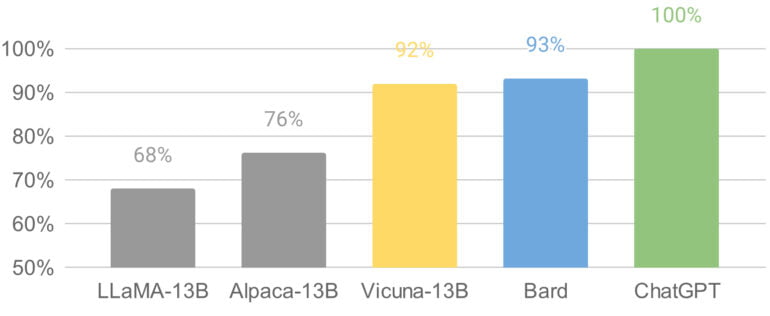
The team pits GPT-4 against a 13-billion-parameter version of Alpaca, Meta's original LLaMA model, Google's Bard, and ChatGPT. GPT-4 shows ChatGPT on top, Vicuna and Bard almost tied, Alpaca and LLaMA far behind. However, the GPT-4 benchmark is "non-scientific" and further evaluation is needed, the team said.
Vicuna model is available for non-commercial use
Vicuna has known issues such as weaknesses in reasoning and math and produces hallucinations. For the published demo, the team also relies on OpenAI's moderation API to filter out inappropriate output. "Nonetheless, we anticipate that Vicuna can serve as an open starting point for future research to tackle these limitations."
With its first release, the team released relevant code, such as for training. It has since also released the Vicuna-13B model weights, although these require an existing LLaMA-13B model.
Those who want to try Vicuna can do so via this demo.