A new study led by Meta's Head of AI Yann LeCun demonstrates how artificial intelligence can develop basic physics understanding just by watching videos. The findings support LeCun's alternative vision to generative AI and challenge approaches like OpenAI's Sora.
The research team, which includes scientists from Meta FAIR, University Gustave Eiffel, and EHESS, shows that AI can develop intuitive physics knowledge through self-supervised video training. Their results suggest AI systems can grasp fundamental physical concepts without pre-programmed rules.
Unlike generative AI models such as OpenAI's Sora, the team's approach uses a Video Joint Embedding Predictive Architecture (V-JEPA). Instead of generating pixel-perfect predictions, V-JEPA makes predictions in an abstract representation space - closer to how LeCun believes the human brain processes information.
The researchers borrowed a clever evaluation method from developmental psychology called "Violation of Expectation." Originally used to test infants' understanding of physics, this approach shows subjects two similar scenes - one physically possible, one impossible, like a ball rolling through a wall. By measuring surprise reactions to these physics violations, researchers can assess basic physics understanding.
Better understanding of physics than large language models
The system was tested on three datasets: IntPhys for basic physics concepts, GRASP for complex interactions, and InfLevel for realistic environments. V-JEPA showed particular strength in understanding object permanence, continuity, and shape consistency. Large multimodal language models such as Gemini 1.5 Pro and Qwen2-VL-72B did not perform much better than chance.
What's particularly notable is how efficiently V-JEPA learns. The system needed just 128 hours of video to grasp basic physics concepts, and even smaller models with only 115 million parameters showed strong results.
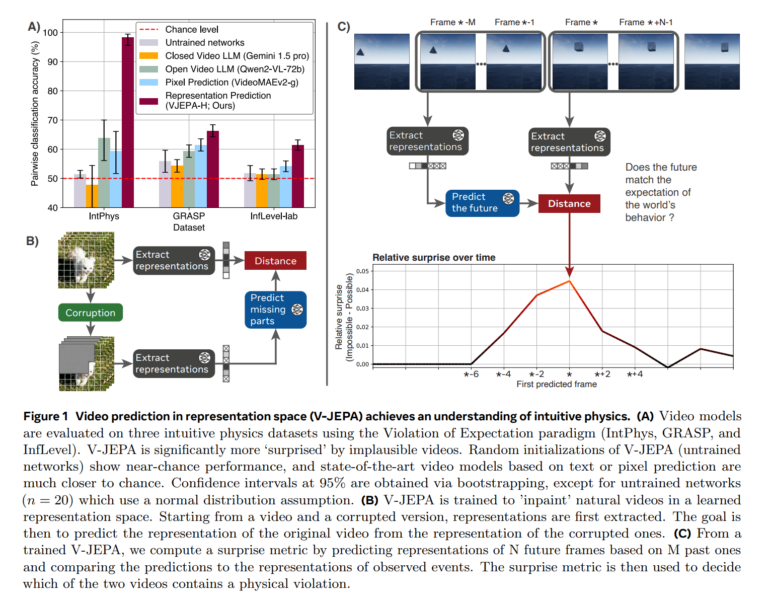
Part of a larger vision for AI development
These findings question a fundamental assumption made by some in AI research: that systems require pre-programmed "core knowledge" of physical laws. V-JEPA shows this knowledge can be learned through observation alone - similar to how infants, primates, and even young birds might develop their understanding of physics.
The study fits into Meta's broader research on the JEPA architecture, which provides an alternative to generative AI models such as GPT-4 or Sora for developing world models. Meta AI chief LeCun considers pixel-perfect generation, like Sora's approach, a dead end for developing world models.
Instead, LeCun advocates for hierarchically stacked JEPA modules that make predictions at various abstraction levels. The goal is to create comprehensive world models enabling autonomous AI systems to develop deeper environmental understanding. The team had already explored this approach with I-JEPA, an image-focused variant, before moving on to videos.