A new AI system reconstructs images from MRI data two-thirds more accurately than older systems. This is made possible by more data and diffusion models.
Can AI models decode thoughts? Experiments with large language models, such as those by a Meta research group led by Jean-Remi King, attempt to decode words or sentences from MRI data using language models.
Recently, a research group demonstrated an AI system that decodes MRI data from a person watching a video into text describing some of the visible events.
These technologies could one day lead to advanced interfaces that, for example, allow people with disabilities to better communicate with their surroundings or control a computer.
A new study now relies on diffusion models to reconstruct images from human MRI data. Diffusion models are also available in advanced image AI systems such as DALL-E 2 or Stable Diffusion. They can reconstruct images from noise.
MinD-Vis relies on diffusion and 340 hours of MRI scans
Researchers from the National University of Singapore, the Chinese University of Hong Kong, and Stanford University demonstrate "Sparse Masked Brain Modeling with Double-Conditioned Latent Diffusion Model for Human Vision Decoding" - MinD-Vis for short.
The work aims to create a diffusion-based AI model that can decode visual stimuli from brain data, laying a foundation for linking human and machine vision.
First, the AI system learns an effective representation of MRI data via self-supervised learning. Embeddings of this data then serve as a condition for image generation of the diffusion model.
For training, the team relies on data from the Human Connectome Project and the Generic Object Decoding Dataset. In total, the training data reaches 136,000 MRI segments from 340 hours of MRI scans, the largest dataset to date for a brain decoding AI system.
MinD-Vis captures semantic details and image features
While the first dataset consists entirely of MRI data, the second includes 1,250 different images from 200 classes. The team withheld 50 of the images for testing.
For further validation of their approach, the researchers also relied on the Brain, Object, Landscape Dataset, which includes 5,254 MRI-image pairs.
According to the publication, MinD-Vis significantly outperforms older models: the system is 66 percent better at capturing semantic content and 41 percent better at the quality of generated images.
In the end, however, this still leaves the system far from being able to reliably read thoughts: Despite the improvement, the accuracy in capturing semantic content is 23.9 percent.
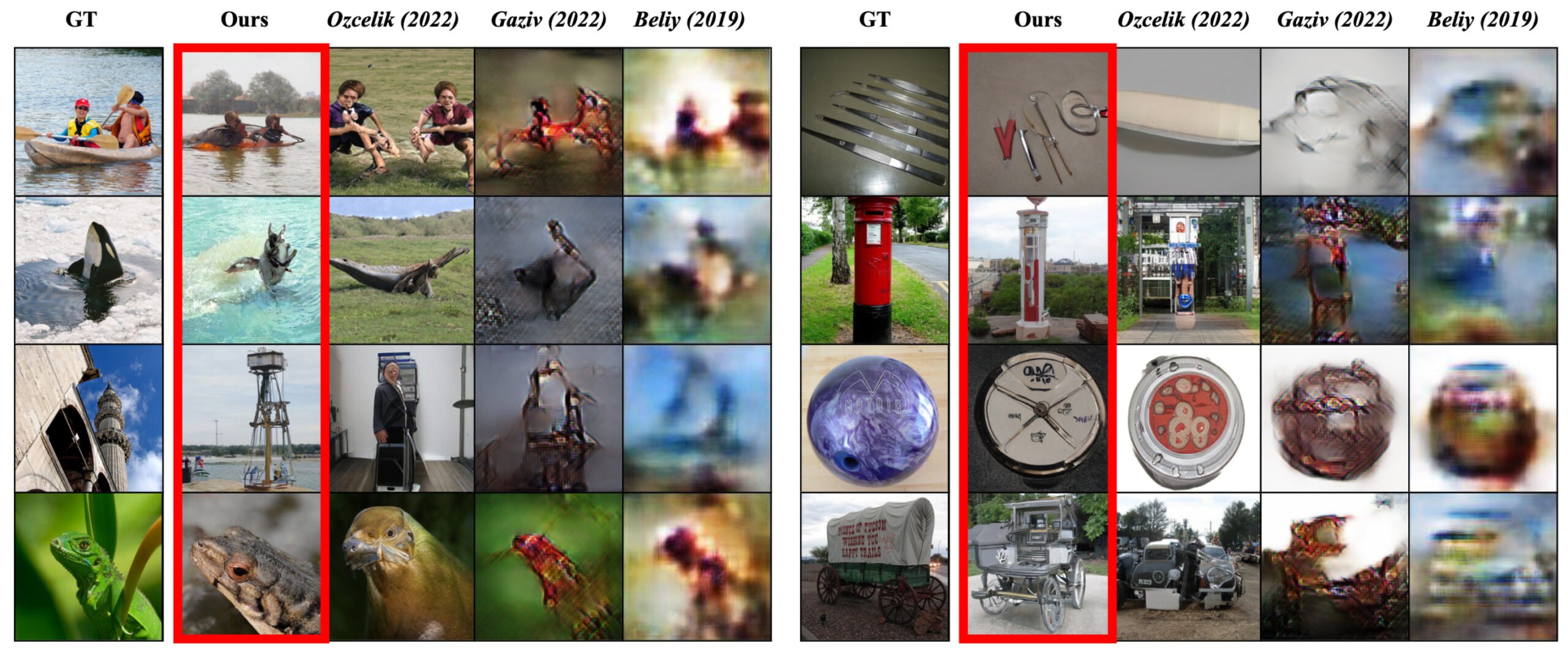
Moreover, the quality of the reconstructed images varied between different subjects. A well-known phenomenon in the research field, the team writes. However, some of the image classes tested were not included in the training dataset. More data could therefore further improve the quality of the system.
More information and examples are available on the MinD-Vis project page.