Generative AI models like DALL-E 2 or Stable Diffusion store their knowledge in their parameters. Meta shows a model that can access an external database instead.
Current generative AI models like DALL-E 2 generate impressive images, but store their knowledge in the model parameters. Improvements require ever larger models and ever more training data.
Researchers are therefore working on several ways to teach models new concepts or provide direct access to external knowledge.
Ideas for such retrieval-augmented methods come from another field of generative AI models: natural language processing. OpenAI, Google, or Meta have already demonstrated WebGPT and other language models that can for example access the Internet to check their answers.
Google and Meta rely on queries for multimodal models
In October, Google researchers demonstrated Re-Imagen (Retrieval-Augmented Text-to-Image Generator), a generative model that uses an external multimodal knowledge base to generate images.
Re-Imagen uses the database to retrieve semantic and visual information about unknown or rare objects, and thus improves the accuracy of image generation.
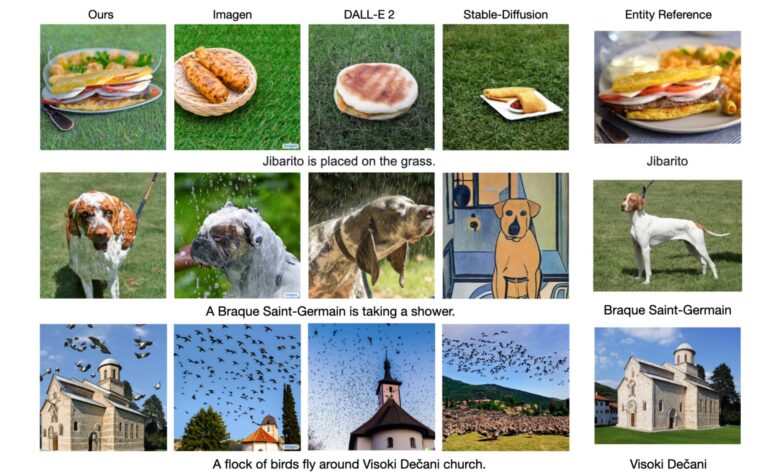
In a new paper, researchers from Meta, Stanford University, and the University of Washington now demonstrate the generative model RA-CM3 (Retrieval Augmented CM3), which also uses external data. CM3 stands for "Causal Masked Multimodal Model" and is a transformer model introduced by Meta in early 2022 that can generate images and text.
Meta's RA-CM3 relies on an external database, making it much smaller
Meta's RA-CM3 was trained using part of the LAION dataset, which was also used for Stable Diffusion. Unlike Re-Imagen, RA-CM3 can process text as well as images. Text prompts and images can serve as input.
The input is processed by a multimodal encoder and passed to a retriever, which retrieves relevant multimodal data from external memory, which is then also processed by a multimodal encoder.
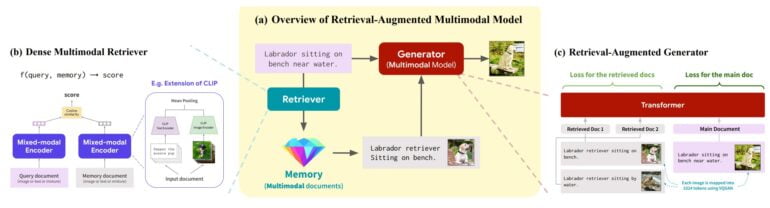
Both data streams are then passed to the multimodal generator, which then generates text or images. RA-CM3 can use external image and text data to generate images that are more accurate or to generate image captions. The database also allows the model to retrieve images of a particular mountain, building, or monument and use it to generate an image containing the object.
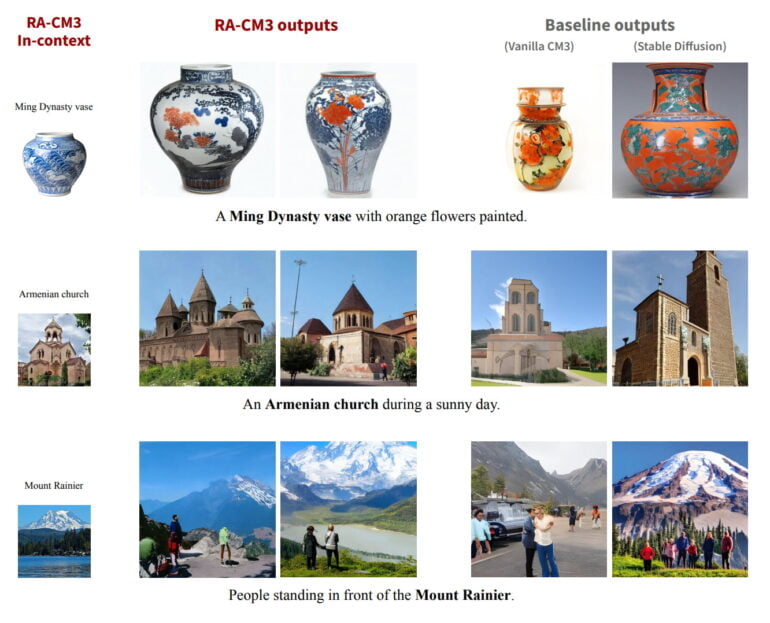
Using the external information, the model can also better complete images. RA-CM3 also exhibits one-shot and few-shot image classification abilities, the researchers write.
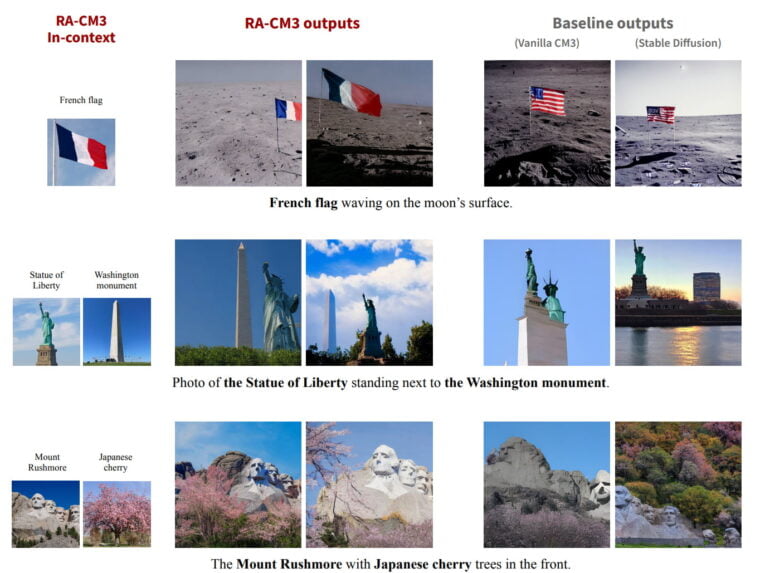
Overall, RA-CM3 uses significantly less training data and resources and is also much smaller than comparable models, the team writes. For the largest model, the researchers used 150 million images and three billion parameters. However, the average quality of the generated images is still below that of the much larger models from OpenAI or Google.
However, the effect of scaling is also evident in RA-CM3: The largest model is ahead of the smaller variants, and the team assumes that larger models will be significantly better.